Molecular biomarkers in early stage lung cancer
Introduction
Multidisciplinarity: a requirement for the current challenges in lung cancer screening and early stage management
Implementation of CT screening for lung cancer, combined with continuous improvements in conventional diagnostics, will undoubtedly increase the number of suspicious pulmonary lesions detected. These improvements highlight the importance of multidisciplinary decision making in the management of these patients (1). Consensus-based recommendations involving thoracic surgeons, radiologists, oncologists, pathologists and pulmonologists are expected to play a central role in the follow up, diagnosis, and treatment of patients with newly detected lung nodules. Screening detected nodules are becoming smaller in size, more peripheral, and frequently less solid when compared to traditionally detected lesions. Furthermore, they can represent a wide variety of histologies, some with potentially indolent growth, for which specific management guidelines are lacking (1). In the present review, we will argue in favor of including molecular biologists, laboratory scientists, and molecular geneticists, in these multidisciplinary teams. We envision that their specific expertise will be increasingly necessary in order to navigate the biological profile of lung tumors in parallel to biomarker discovery and development. In our opinion, biomarkers may help decision making related to risk assessment of asymptomatic individuals, nodule characterization and prognosis, as well as inform surgical management in the treatment of already diagnosed and/or resected tumors. Figure 1 shows a schematic representation of the different types of biomarkers that are being developed for early detection and management of early stage lung cancer. In the present manuscript we aim to define both the current unmet medical challenges in lung cancer screening and early cancer management, together with the potential contributions that may arise from the field of biomarker discovery and development.
Unmet medical challenges in the screening process
Who should be screened
A growing body of scientific evidence supports lung cancer screening with low-dose computed tomography (LDCT). The pioneer NEJM paper by Henschke and co-workers in 2006 (2) showing that LDCT can detect lung cancer, provided data of the clinical value of screening with LDCT for reducing lung cancer mortality. Since then, multiple randomized trials, including the landmark National Lung Screening Trial (NLST), the Dutch-Belgian NELSON trial, and the Italian MILD trial, have demonstrated a reduction in lung cancer mortality using LDCT as a screening tool (3-5). However, much needs to be done in order to refine recommended screening criteria, largely based on the NLST findings. Screening smokers older than 55 with more than 30 pack years of cumulative exposure to cigarette smoking clearly leaves many individuals at risk out of existing screening protocols (6-8).
The matter of selection criteria is of paramount importance. Screening all smokers may be a daunting task and prove to be quite expensive and increases the risk of performing unnecessary procedures for benign nodules. Therefore, limiting screening to those with the greatest risk may be more cost effective. On the other hand, screening only based on age or smoking exposure, may exclude those who stand to benefit the most, i.e., younger individuals with greater potential for long-term survival, thereby limiting screening benefits on quality-adjusted life years (QALY) and cost effectiveness. A biomarker capable of refining existing inclusion criteria might help in both ways, by excluding subjects theoretically at risk based on age and tobacco exposure, thereby precluding unnecessary screening, while including younger smokers who may be at greater risk than anticipated.
Adequate evaluation of the risk of malignancy, as well as the fitness of a given patient to undergo further intervention or resection, should be a multidisciplinary task centered on improving patient outcomes (1,9,10). Multiple quantitative risk assessment models have been validated in order to evaluate the risk of malignancy, mainly based in epidemiological/clinical criteria. Examples are the Liverpool Lung Project (LLP) risk model (11) or the PLCO2012 risk prediction model (12). Salient clinical features include; age (13,14), smoking status (15), history of prior malignancy (16), presence of chronic obstructive pulmonary disease (COPD), particularly the emphysema phenotype (17), and family history (18). A recent review, mainly directed to primary care physicians addresses this relevant issue (19).
Nodule characterization
LDCT based screening, while very sensitive, has a low specificity. Validated management protocols have been shown to be effective in avoiding excessive unnecessary invasive diagnostic procedures (20). Moreover, a high prevalence of indeterminate nodules detected on LDCT may lead to more testing and anxiety. Therefore, there is an unmet clinical need to develop standardized and robust biomarkers capable of refining screening inclusion criteria and inform decisions based on LDCT screening findings (21). Radiomic and molecular approaches are both currently being explored.
Current management of LDCT identified lung nodules is largely based on serial imaging at specific intervals in order to determine doubling time, which in turn informs decisions regarding follow up or invasive testing. Massion et al. have recently demonstrated that the application of a novel deep learning algorithm to chest computed tomography scans is a useful tool to correctly reclassify IPNs into low- or high-risk categories (22). Since most nodules detected by screening are relatively small, a biomarker-based risk assessment can also be helpful in this regard. The development by Vachani and co-workers (23) of a molecular biomarker based on bronchial epithelial gene expression obtained from normal-appearing epithelium during bronchoscopy is an example. This biomarker can stratify the risk of malignancy of suspicious lesions with inconclusive biopsy results, in order to help decision making in the clinical setting. However, this biomarker requires a bronchoscopy, an invasive procedure. Therefore, biomarkers analyzed in samples obtained non-invasively (either in blood, sputum, urine or exhaled breath) would be ideal. We will summarize in the next paragraphs some of the most relevant candidates.
In addition, biomarkers may prove useful during follow up of patients with screening diagnosed lung cancer, aiding prognosis, defining the need for treatment with adjuvant therapies, and detecting minimal residual disease or second primary malignancies.
Radiological challenges
The potential role of radiomics and radiogenomics
Radiomics are defined as the extraction of quantitative and qualitative data from radiological images to create databases than can help diagnose and predict prognosis and the response to treatment of different malignancies, including non-small cell lung cancer (NSCLC) (24,25). Radiomics have been applied to lung cancer screening in order to increase its sensitivity and specificity (22,26). Several radiomic-based predictive models have been already proposed for CT-based early detection of lung cancer. These models show a great potential as tools for clinical decision-making in lung cancer screening (27,28) although some caveats and limitations such as radiomic workflow lack of repeatability or reproducibility under particular circumstances still need to be considered (29). The field of radiogenomics combines radiomics with genetic biomarker data (30).
A major challenge is to obtain comprehensive information regarding tumor biology based on studying large imaging datasets using artificial intelligence. Unfortunately, due to time, costs and data analysis limitations, large scale-genome lung cancer characterization is still not available in early stage lung cancers (31). In recent years, different subtypes of lung adenocarcinoma (ADC) harboring actionable mutations or translocations (EGFR, KRAS and ALK) have been identified leading to personalized therapies (32). The search for a correlation between these genetic findings and radiological data is a worthwhile pursuit, albeit thus far yielding somewhat contradictory results. Glynn and cols. (33) were not able to find a relationship between radiological data and EGFR or KRAS-mutations. On the other hand, Lee and cols. (34) reported an association between CT and PET/CT findings [tumors >4 cm diameter or >5.0 standard uptake value (SUV) or ground glass opacity component <50%] and EGFR overexpression. The same group was able to differentiate between the two most common EGFR mutations (exon 19 deletions, and exon 21 missense mutations) based on CT-tumor morphology, finding a more prominent ground glass component in patients with exon 21 missense mutations (35). Yano and cols. (36) also highlighted the association between a tumor’s ground glass component and the likelihood of an EGFR mutation. In the study of Rizzo and colleagues (37) qualitative and quantitative features such as air bronchograms, pleural retractions, smaller size and the absence of fibrosis tended to be associated with EGFR mutations while pleural effusion was associated more frequently with ALK translocations. Furthermore, a round shape, the presence of other nodules in different lobes and smoking status were associated with KRAS mutations. These findings were confirmed by Halpenny and cols. (38), whose study showed that ALK-mutated tumors were associated with pleural effusion and mediastinal adenopathy and were larger in size.
RET and ROS1 mutations, although less frequent, have also been addressed in other models. Plodkowski and cols. (39) found that peripheral tumors were associated more frequently with ROS1 rearrangements, while solid tumors with spiculated borders tended to harbor RET, ROS1 and EGFR mutations. These subgroups of tumors rarely presented cavitation or calcification (39). Nair and colleagues (40), in their attempt to correlate genome expression with different FDG uptakes on PET-CT, found a link between SUV values and nuclear factor kappa-light-chain-enhancer of activated B cells (NFKB), a protein complex related to DNA transcription, cytokine production and cell survival. More recently, in order to try to predict tumor behavior and response to adjuvant treatment, Vaidya and cols. (41) developed and validated a quantitative radiomic risk score and an associated nomogram for early stage NSCLC (stages I and II) capable of predicting disease-free survival (DFS) and the added benefit of adjuvant chemotherapy following surgical resection.
Surgical challenges: the reduction of false positives and the decision on the type of intervention
Limiting false positive findings to a minimum remains an on-going challenge of screening. A high false-positive rate is neither ethically nor economically acceptable. However, a balance must be struck between achieving a high-detection rate with its consequent reduction in mortality, and the lowest possible false-positive rate.
With the advent of more standardized diagnostic algorithms for IPNs, the false positive rates have reduced considerably, with an average of 18% false positives in the context of lung cancer screening programs (42). In our subcohort of I-ELCAP in Pamplona, the rate of interventions for benign disease was 9.2% (43).
Several emerging biomarkers address the issue of reducing the risk of futile surgeries for benign lesions by improving the characterization of indeterminate pulmonary nodules found by LD-CT based screening or routine clinical practice. Section 3 of the present review, devoted to biomarkers in screening, provide detailed examples of these efforts to validate nodule characterization with molecular markers.
As a result of advances in surgical treatment, mortality reported after diagnostic lung surgery is lower than 1% (44,45), or practically nonexistent in the case of video-assisted thoracoscopic surgery (VATS) wedge resection (46,47). Minimally invasive approaches and sublobar resections may become the surgery of choice for diagnostic interventions, always bearing in mind the challenge of identifying and locating these small lesions intraoperatively (48,49). Biomarkers are not standard of care in surgical NSCLC, although well-established and routinely monitored biochemical data are logically considered when evaluating a patient’s general status and eligibility for a given surgical approach. The combination of systemic or local (sputum, exhaled breath, etc.) biomarkers with concurrent morbidities such as COPD or emphysema, and other clinical parameters as well as quantitative radiological imaging, will very likely be a useful multidisciplinary approach to help in the surgical decision-making process. In the context of early lung cancer in screening cohorts, the finding of molecular or integrated molecular/clinical/imaging markers that may facilitate the surgical decision making protocol is still an unmet clinical need.
Challenges in pathology
A histopathological diagnosis, precise staging and, eventually, prognostic information are of the utmost importance in any tumor, and particularly in early cancer stages. For isolated suspicious pulmonary nodules, in the context of screening, it is also important to consider the emerging roles for the pathologist. Rapid on site evaluation (ROSE) of these nodules is an example, highlighting once more the importance of a multidisciplinary approach. The widespread implementation of screening has led to the detection of small nodules, often less than 1 cm, which pose a challenge to frozen section analysis (50). There is an unmet medical need to develop robust, rapidly available and standardized molecular biomarkers to provide unequivocal diagnostic information for ROSE, quick frozen section analysis or fine needle aspiration material. Fast technological development to adapt next-generation sequencing (NGS) and other molecular techniques to cytology samples with very small amount of DNA is currently thriving in NSCLC diagnosis. Examples are the detection of EGFR mutations and ALK or ROS1 rearrangements or of the expression of PDL-1 in small cytology specimens (51).
The introduction of screening for lung cancer is increasing notably the proportion of early stage diagnoses, in particular stage I lung tumors. This has posed additional main challenges to the pathologist. The efforts of pathology research in this field are currently focused on the development of more refined algorithms (very likely using artificial intelligence) to: (I) determine definitive diagnostics, including molecular classification of tumor type; (II) refining staging by better predicting outcome and aggressiveness or the potential presence of minimal residual disease in early stage lung cancer patients; (III) advising for the need of adjuvant treatment in a given patient; and (IV) suggesting potential adjuvant treatments based on the biology of the tumor. Very likely, diagnostic, prognostic and predictive algorithms will be based on a combination of image analysis and molecular data. The recent results of the ADAURA trial showing significantly longer DFS in NSCLC patients with stage IB to IIIA EGFR mutation positive receiving osimertinib as compared to those who received placebo improves previous results with first and second generation EGFR-TKIs (52). The impressive results of this trial strongly advise for routine molecular (at least EGFR mutation) testing of NSCLC patients irrespective of the tumor stage (53). Thus, it is very likely that a call for routine deeper molecular characterization of the early stage lung tumors found in the context of CT-based screening will be around the corner.
Current biomarkers in lung cancer screening
Molecular biomarkers are biological indicators. A good biomarker should be accurately and robustly measured in accessible samples. The rationale to develop molecular biomarkers for lung cancer screening is well established, since altered molecules derived from cancer cells or the tumor microenvironment are released into fluids and can be quantified with highly sensitive technologies. A clinically meaningful biomarker should be quantified with reproducible and cost-effective methods and should provide valuable information for the clinical management of lung cancer (54).
Blood-borne biomarkers would be ideal for screening, because these samples recapitulate tumor heterogeneity and represent both the primary tumor and the different metastatic lesions. They may also reflect the host response to the presence of a neoplastic lesion. Although most of the information included in the present review refers to blood biomarkers, other potential sources of biomarkers include sputum, bronchoalveolar lavage (BAL), or bronchial aspirate samples. The biomarkers found in upper airway secretions may be more closely related to lesions in the central airways, but also more likely to miss peripheral tumors (55). Exhaled breath biomarkers (either measuring volatile compounds or different molecules found in the breath condensate) are also being studied and tested actively (56,57). Saliva (58) and urine (59) have also been explored as potential sources of lung cancer early detection biomarkers, but these studies are still in a very early discovery phase. A recent review has described three biomarker commercially available tests (Early CDT-Lung, Nodify XL2, Percepta) which have undergone extensive validation and are available to the clinician. Clinical applicability and limitations of these tests and future directions of lung cancer screening biomarkers are also discussed (60). Emerging biomarkers and new trends for the future of lung cancer screening biomarker development have been recently summarized elsewhere (61).
Many efforts have been devoted to identify molecular biomarkers, especially in blood samples, but none of the currently available candidates has been fully validated and none has been tested in a trial to show that its use contributes to a reduction in lung cancer mortality. The clinical use of a given diagnostic biomarker requires a long, tedious and expensive process of validation to demonstrate its clinical usefulness: i.e., its capacity to orient clinical decisions in order to improve patient outcomes. Full clinical validation in the lung cancer screening context has not been reached yet. Nonetheless, massive amounts of biological information are being recorded, including several steps in the clinical front for some of the candidate biomarkers. The tests in intended-to-use (LDCT screening cohorts), the development and completion of properly designed trials, and the increasing interest in developing complementary tools to render LDCT screening more cost-effective, are the engines that will lead promising candidates into routine and reliable clinical use in the upcoming years.
A range of promising biomarkers has been identified in biological samples. Table 1 and this section summarize those that we consider the most promising to address the two main unmet needs for biomarkers in the context of lung cancer screening, namely risk management and the characterization of indeterminate pulmonary nodules found on LDCT imaging of high-risk individuals. Due to its non-invasive procedure and great potential, liquid biopsy will probably become a standard diagnostic, prognostic and predictive tool in NSCLC (97).
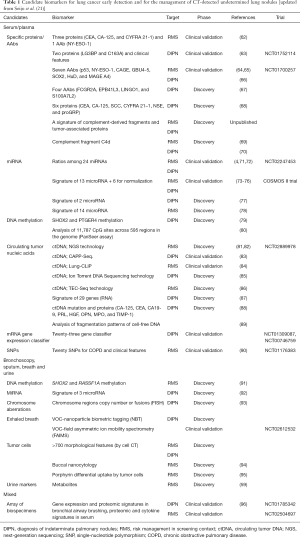
Full table
Autoantibodies (AAbs)
AAbs develop in response to tumor antigens and may be found in the plasma of asymptomatic lung cancer patients. An AAbs panel has been validated in different screening cohorts to assess lung cancer risk, and may help differentiate between benign and malignant lesions (64,65,98-100). The high specificity of AAbs indicates that they are suitable to improve the diagnostic performance of mixed panels of biomarkers and complement the results of high sensitivity imaging studies (101). The most advanced test based on AAbs. Early CDT-Lung test has been studied for clinical validation in several cohorts of patients with newly diagnosed lung cancer, showing a specificity of approximately 90% at sensitivities of around 40% (102). Recently, the results of a long time waited trial in Scotland, using the score from the Early CDT-Lung test to randomize more than 12,000 high risk individuals to CT-screening or standard of care follow up when the study begun, have been published. A reduction of the incidence of patients with stage III/IV lung cancer at diagnosis was observed. The intervention arm received the Early CDT-Lung test and, if test positive, low-dose CT scanning 6-monthly for up to 2 years. Early CDT-Lung test negative and control arm participants received standard clinical care. In the intervention arm, 58.9% lung cancers were diagnosed at stage III/IV compared to 73.2% in the control arm. The hazard ratio (HR) for stage III/IV presentation was 0.64 [95% confidence interval (95% CI): 0.41, 0.99]. Nevertheless, sensitivity in this recent trial was even lower than in previous trials, at 32.1%, with a preservation of specificity at 90.3%. Of note, sensitivity was particularly low for stage III or IV cancers, at 18.2%. There were non-significant differences in lung cancer and all-cause mortality after 2 years (103).
Blood circulating proteins other than AABs
Several panels of proteins have been proposed for early detection, the most advanced of which is a panel of proteins discovered by mass spectrometry multiple reaction monitoring technique. Initially the authors reported a 13-protein proteomic classifier that gave a 90% negative predictive value (NPV) for benign nodules (104). The same group proposed a 5-marker subset of the original 13 proteins together with 6 normalization markers (105). More recently, the same group discovered that the accuracy of two of the proteins, LG3BP and C163A (independently linked to lung cancer and the inflammatory response to cancer) could be optimized for evaluating lower risk nodules by integrating them with five clinical risk factors (nodule location, size, spiculation and patient’s age and smoking history). The classifier was commercialized as XL2TM TEST by Biodesix. An observational clinical trial (PANOPTIC) was carried out with this classifier A subgroup of 178 patients with a clinician-assessed pretest probability of cancer (pCA) ≤50% had a 16% prevalence of lung cancer. The integrated molecular/clinical classifier demonstrated a sensitivity of 97% (CI, 82–100), a specificity of 44% (CI, 36–52), and a NPV of 98% (CI, 92–100) in distinguishing benign from malignant nodules. According to the results in this trial, if the integrated classifier was used to direct care, 40% fewer procedures would be performed on benign nodules, and 3% of malignant nodules would be misclassified (ClinicalTrials.gov; No. NCT01752114) (63).
Complement fragments
The activation of the complement system has been proposed as a potential biomarker for the clinical management and diagnosis of lung cancer. Lung cancer cells activate the complement system through the classical pathway. C4d levels, a downstream molecule derived from this activation, are increased in the biological fluids obtained from lung cancer patients (69,70,106). The value of this biomarker for both lung cancer screening and the management of indeterminate pulmonary nodules has already been reviewed (21). More recently, a novel model based on the quantification in plasma of both complement activation-derived fragments and previously proposed cancer biomarkers has been shown to predict higher risk of lung cancer in asymptomatic individuals enrolled in a CT-screening program. Moreover, the scores derived from this model are associated with higher probabilities of malignancy in patients with indeterminate-risk lung nodules (unpublished data).
miRNAs
Many studies have reported the potential value of plasma miRNA reflecting underlying disease as a biomarker for the clinical management of lung cancer. A plasma signature of 13 miRNA (miR-Test) (73-75) and a signature composed by reciprocal ratios among 24 circulating miRNAs [miRNA signature classifier (MSC)] (4,71,72) have demonstrated high diagnostic accuracy in the screening setting. The prospective validation of these signatures is currently under investigation in three independent screening trials.
Circulating tumor DNA (ctDNA)
The value of ctDNA for the clinical management of lung cancer has been intensely explored in the recent past. Several NGS-based approaches have been proposed for lung cancer diagnosis [reviewed by Abbosh et al. (81)]. A prospective, multi-center, observational study enrolling at least 15,000 participants is currently evaluating the value of deep sequencing of circulating cell-free nucleic acids as a biomarker for the detection of different types of cancer at various stages. Recently, a method to improve the sensitivity of the mutational analysis of ctDNA in blood, based on CAPP-Seq, has been proposed and tested in the context of lung cancer screening. Although levels are very low in early-stage lung cancers, ctDNA is present prior to treatment in most patients and its presence may have prognostic value. Furthermore, most of the somatic mutations found in ctDNA of patients diagnosed with lung cancer and of risk-matched controls reflect clonal hematopoiesis, and are non-recurrent. A tool called ‘lung cancer likelihood in plasma’ (Lung-CLiP), integrating ctDNA findings with other molecular features, can discriminate early-stage lung cancer patients from risk-matched controls (84). The PanSeer, a noninvasive blood test based on ctDNA methylation, detects lung cancer at early stages (80). Finally, a recent study demonstrate that the fragmentation patterns of circulating cell free DNA may be a useful tool to detect lung cancer (89).
Biomarkers in exosomes
Exosomes are stable, easily collected from body fluids, and are apparently good “concentrators” of otherwise free-circulating biomarkers, thus becoming suitable candidates for liquid biopsies. Furthermore, exosomes are more abundant in cancer patients (107), indicating that tumor or host cells in cancer patients produce more exosomes than healthy individuals (108). Diagnostic studies related to exosomes have mainly focused on miRNA and proteins. miRNAs are the most abundant nucleic acids in exosomes. The following are some examples of discovery efforts of novel diagnostic biomarkers based on molecules stored in exosomes. The level of robustness, reproducibility and maturity of the results of the following examples are heterogeneous and most of them require further validation. miRNA signatures have been considered potential biomarkers in NSCLC since 2009, because miRNA isolated from NSCLC patients’ exosomes mirrored miRNA signatures in NSCLC. Several miRNA-panels have been proposed for NSCLC diagnosis in exosomes isolated from plasma/serum (109-117), BAL (118) and pleural fluid (119,120). Expression levels of exosomal MALAT-1, a long non-coding RNA (lncRNA), may have potential value in NSCLC diagnosis (121). Other nucleic acids, such as exosomal dsDNA or mRNA from NSCLC patients have shown potential, but evidence is currently limited to cell lines (122) and animal models (123). Exosome-derived proteins have emerged as a new source of potential biomarkers for NSCLC from early to advanced stages in blood (124-126), saliva (127), urine (128) and pleural fluid (129). An exosomal lipid profile from peripheral blood can discriminate normal from early and late stage NSCLC patients (130). However, exosomes clearly need further study to reach routine clinical practice, since their current sensitivity and specificity in the diagnosis and treatment of NSCLC are far from ideal. They are also still in a very preliminary phase in the validation arena.
Other possible sources of biomarkers
DNA methylation and polymorphisms, chromosomal abnormalities, RNA, tumor-associated proteins, proteomic signatures, breath volatile compounds, urine metabolites, and cytological analyses have also been proposed as potential biomarkers for the diagnosis of lung cancer (see Table 1) in the context of screening. The integration of these models with clinical and radiological (radiomics) features may improve the performance of screening for asymptomatic high-risk individuals and also discriminate between benign and malignant nodules.
Current biomarkers in early lung cancer management: prognostic factors
Correct histopathological evaluation is crucial in NSCLC clinical management. Although either SCC or ADC are considered to have a similar prognosis for a given tumor stage, additional histopathological subtyping has strong prognostic implications. There are three prognostic categories for lung ADCs (131-133), considering the current WHO classification. Adenocarcinoma in situ (AIS) and minimally invasive adenocarcinoma (MIA), which may soon be considered a single entity (132), followed closely by lepidic predominant tumors, can be classified as low risk. Acinar and papillary predominant tumors may be considered intermediate risk, while micropapillary, solid, mucinous or colloid tumors should be viewed as high risk. Recent studies (134,135) suggest that intermediate risk (acinar and papillary) ADCs can be further subdivided into low and high grade. The cribriform and fused pattern subsets of acinar predominant tumors and the complex papillary patterns are considered high grade.
Squamous cell cancer (SCC) subtypes (keratinizing, non-keratinizing, basaloid pattern, papillary growth, and clear cell feature) have limited prognostic impact. Tumor nest size (tumor budding and single cell invasion), and nuclear grade have been reported as independent prognostic factors in SCC (136).
Lymphovascular invasion (LVI) (137) is associated with worse recurrence-free survival (RFS) (HR: 3.63, 95% CI: 1.62 to 8.14; 4 studies; 1,147 patients) and overall survival (OS) (HR: 2.38, 95% CI: 1.72 to 3.3). Similarly, a recent meta-analysis suggests that high Ki-67 expression is inversely proportional to OS (HR: 1.59, 95% CI: 1.35 to 1.88) and DFS (HR: 2.21 95% CI: 1.43 to 3.42) in NSCLC patients (138). The prognostic value of immune cell infiltration of the tumor microenvironment in early-stage lung cancer has been broadly analyzed by several studies. Tumor-infiltrating lymphocytes (TILs) have been evaluated by different methods including the International Immuno-Oncology Biomarkers Working Group consensus proposal. Most studies have shown that high TIL levels are associated with improved DFS and, less frequently, with an increased OS. A meta-analysis of the role of immune cell quantification by immuno-histochemistry performed by Tuminello and cols. (139), revealed that higher levels of CD8+ cytotoxic T cells, CD56/57+ NK cells, and CD20+ B cells are associated with improved OS or DFS. Lung cancers with increased FoxP3+ T regulatory cells have worse OS. Additional prognostic and predictive biomarkers for early stage lung cancer have been proposed (140-142). Biomarkers identified by immunohistochemistry (p53, Cyclins, Her2, EGFR, etc.), gene expression signatures, methylation or genotyping, although in some cases have been validated (141), have not shown conclusive results and are still experimental. Tumor spread through air spaces (STAS) was proposed in 2015 (143) as an important prognostic factor in lung ADC, especially when sublobar resections have been performed. The surgical procedure-specific outcome can be stratified by STAS (144,145). The expected prevalence of STAS in most studies ranges from 30% to 40% in ADCs. Expertise and careful handling of specimens is required to avoid artefacts that may be misinterpreted as STAS. In our experience, STAS is more frequently found in high grade ADC subtypes (unpublished observation).
Some degree of prognostic value has been shown for more than 200 molecular markers in lung cancer, but their use in routine clinical practice is limited by the lack of both reproducibility and independent validation. Microarray technology has emerged as a powerful tool measuring the expression of hundreds of genes in a high number of samples, and different prognostic mRNA expression signatures have been described in lung cancer. In response to the concern that microarray-based profiles are difficult to apply in a clinical setting, subsequent efforts have focused on developing quantitative polymerase chain reaction (qPCR)-based molecular signatures. These gene signatures can be analyzed by q-PCR, the gold standard assay for gene expression. Only a few of the initially proposed prognostic qPCR based signatures can withstand the need for quality RNA extraction from fresh frozen specimens. The use of novel RNA extracting and preserving technologies may overcome this limitation, by allowing RNA extraction from formalin fixed, paraffin embedded (FFPE) samples. Xie and cols. developed a 59-gene prognostic signature for NSCLC using genome-wide expression profiling in FFPE samples (146). Kratz and cols. defined a 14-gene prognostic profile for non-squamous NSCLC using qPCR analysis on the same type of samples (147-149). Wistuba and cols. proposed a proliferation-based expression signature of 31 genes for ADC histology subtype (150). Finally, Bianchi and cols. have developed and validated a 10-gene prognostic signature in a large cohort of lung ADC patients using qPCR, also on FFPE samples (151). All of them show promise, but are awaiting further validation.
Recently we have developed and validated two new molecular prognostic signatures for ADC and SCC (152,153) using immunodetection-based techniques to assess the expression of 3 and 5 prognostic proteins (for ADC and SCC respectively). Because of its wide availability (including the type of sample: FFPE) and affordability, immunohistochemistry is still one of the preferred ways to introduce single novel biomarkers into clinical practice (154). Moreover, an IHC-based prognostic signature for SCC is a major advance, since the aforementioned qPCR based signatures have been validated only for non-squamous histologies or ADC. More recently, novel qPCR-based studies have been published for SCC prognostication, but require further validation (155,156). The protein panels developed by our group mentioned above identify a subset of stage I–II patients with a higher risk of recurrence and survival who may need more aggressive therapy or closer follow-up. This classifier improves the prognostic value of TNM staging, and is able to identify a group of early stage ADC or SCC patients as potential candidates for adjuvant chemotherapy. Jin and cols. have also proposed similar protein-based signatures for both ADC and SCC (157). As the lists of proteins in both studies do not overlap, it is possible that a combination of both signatures would have even stronger prognostic value.
Finally, a variety of recently published artificial intelligence-based studies (158,159) also show that complex comprehensive computational pathological image analysis can give rise to algorithms to predict lung cancer prognosis. The combination of sophisticated deep-learning based image analysis and robustly validated prognostic markers will probably be the way forward to obtain clinically useful prognostic information in the coming years.
Current biomarkers in early lung cancer management: predictive factors for adjuvant therapy
Adjuvant therapy in NSCLC is still a controversial subject, and, although supported by different societies (160-163), its indications, especially in early stage NSCLC, remain unclear. Adequate patient selection in order to maximize the benefit of these therapies, while limiting toxicity, is key (164). Current patient selection is based on the risk of recurrence determined by pathological stage (164), age, comorbidities and performance status.
In the previous section, we have described recent advances to refine personalized prognostic information, which may also be helpful in deciding whether to offer adjuvant therapy. There are ongoing efforts to combine pathological stage with biomarkers to further refine the selection criteria, bust most of them remain investigational and need to be validated in prospective clinical trials (147,152,153).
Modern clinical trials assessing the benefits of adjuvant chemotherapy in early stage lung cancer have yielded controversial results. The Adjuvant Lung Project (ALPI) trial (165), the Big Lung Trial (BLT) (166) and the CALGB 9633 trial (167) found no improvement in 5-year survival and DFS. On the other hand, the International Adjuvant Lung Cancer Trial (IALT) (168), a trial from Canada and the US (169), and the Adjuvant Navelbine International Trialist Association (ANITA) trial reported a benefit in median survival and DFS with adjuvant chemotherapy (170). Side effects of adjuvant treatment have been a constant concern in these trials, as well as the poor compliance with the planned chemotherapy schemes, especially considering the target group are post-resection patients. All of the aforementioned trials included a platinum-based chemotherapy regimen (165-170). Older studies which included patients with non-platinum based regimens, including alkylating agents (mytomicin C and ifosfamide), showed a decreased OS and increased chemotherapy-related complication rates (171). Furthermore, The Lung Adjuvant Cisplatin Evaluation (LACE) meta-analysis (172) demonstrated that the companion drug administered with cisplatin did not modify the effect of adjuvant therapy.
Adjuvant chemotherapy following complete resection may be associated with a 5.4% increase in 5-year OS for patients with stage II–IIIA NSCLC (HR: 0.83; P=0.004) (172). Although a cisplatin/vinorelbine regimen has been widely used (173), a combination of cisplatin and pemetrexed demonstrated a similar efficacy profile with better tolerability in non-squamous histology (174). Bevacizumab does not appear to improve outcomes in this setting (175), while the role of adjuvant chemotherapy in stage IB NSCLC remains controversial. Only an exploratory analysis from CALGB 9633 (167) demonstrated a significant survival benefit in favor of paclitaxel/carboplatin for patients who had tumors ≥4 cm in diameter (HR: 0.69; P=0.043). Only the non-squamous histology is predictive of efficacy in the context of pemetrexed (176).
Several molecular markers, such as excision repair cross-complement group 1 (ERCC1), ribonucleotide reductase regulatory subunit M1 (RRM1), breast cancer-specific tumor suppressor protein 1 (BRCA1), and receptor-associated protein 80 (RAP80), have been assessed, but abandoned for lack of efficacy. The value of thymidylate synthase (TS) has just been studied in the ITACA trial, pending results (177-181). Finally, there has been a great interest in identification of specific gene signatures based on gene expression (147,182), methylation of DNA (183,184), or miRNAs (185,186) that can provide prognostic and predictive guidance: however, none of the studies to date have proven their clinical value in prospective trials.
Since one of the major advances in the management of metastatic NSCLC has been the identification of driver genetic alterations that can be beneficially targeted, EGFR-TKIs have also been tested in the adjuvant scenario (187,188). The first reported trial of EGFR inhibitors in early stage lung cancer patients was terminated prematurely (189) due to lack of efficacy when compared to placebo. A subsequent trial showed an improved 2-year DFS in stage IIIA patients treated with erlotinib when compared to cisplatin and vinorelbin in patients harboring EGFR mutations (190). Osimertinib has very recently proved beneficial for the DFS in the ADAURA trial (4). The difference between the two groups (treated and placebo) was very significant in this trial. At 24 months, 90% of the patients with stage II to IIIa disease in the osimertinib group (95% CI: 84 to 93) and 445 of those of the placebo group (95% CI: 37 to 51) were alive and disease free (overall hazard ratio for disease recurrence or death, 0.17 vs. 0.26; P<0.001). We are now eagerly waiting for the OS results, which also will be relevant in the area of lung cancer screening to address the question on the relevance of targeted adjuvant therapy in early tumors diagnosed in screening cohorts. In addition to TKIs, the role of adjuvant crizotinib in ALK positive patients is also being evaluated (191).
In the field of immunotherapy, targeting melanoma-associated antigen-A3 (MAGE A3) (192) was addressed in the MAGRIT study (193). Unfortunately, no benefit in disease-free or OS was found when compared to placebo. A liposome peptide vaccine to mucin 1 (MUC1) glycoprotein known as tecemotide (L-BLP25) has also been studied. A phase III study did not report any advantage in survival of stage III NSCLC patients treated with tecemotide when compared to placebo (194). Pembrolizumab, a PD-1 inhibitor, is approved as first line therapy for metastatic NSCLC in patients with more than 50% of PD-L1 expression (195). Currently, its efficacy as adjuvant therapy in early stage lung cancer is being evaluated in multiple trials (196) with promising results. Ongoing clinical trials, including combined drugs and chimeric antigen receptor (CAR)-T cell treatment (197), will improve our understanding of immune mechanisms related to NSCLC and help develop new therapies to improve early stage NSCLC outcomes.
Future directions of molecular biomarkers in early stage lung cancer: the need for artificial-intelligence based integration
The identification and validation of phenotypes combining clinical, radiological and genetic data have the potential for great clinical impact. The development of these predictive models will not only improve evaluation, risk stratification, diagnosis and treatment of NSCLC patients, but also, make clinicians’ work less cumbersome by allowing for an integrated and practical analysis of all patient characteristics (198).
To reach this goal, it is of the utmost importance to establish prospective, independent, and properly audited databases able to capture clinical, radiologic, surgical and genetic information in a standardized, reproducible manner. Furthermore, the processing of the massive amount of data generated will be facilitated by the correct application of these models (198,199). Finally, the optimal design of clinical trials to show evidence based clinical utility of the integrated algorithms combining together imaging and clinical data need to be discussed and agreed with the regulatory agencies. There are a number of potential designs each with advantages and limitations (55). Despite the wide range of molecular biomarkers available in the laboratory, the fact is that none of them has been integrated in routine clinical practice. Their implementation and validation in early stage lung cancer patients has become the greatest challenge to date. Scant improvement in lung cancer related deaths mandates refinement of current staging systems. Whether or not these refinements will come from prognostic molecular and clinical markers alone is still unclear. The same holds true for our capacity to predict the efficacy and choose the modality of adjuvant treatments. We need a better understanding of the detailed molecular makeup of early stage lung tumors and its correlation with outcomes and response to therapy.
Furthermore, well-implemented and established lung cancer screening programs, carried out by experienced multidisciplinary teams, will set the stage for streamlined cost effective protocols. Integration of the different molecular signatures with clinical and radiological manifestations and the evaluation of their impact in long-term outcomes will be of paramount importance. The design of artificial intelligence models that can help in the integration of all the data and in the decision-making process will definitely be welcome and facilitate specialists’ work.
As researchers and clinicians, we need to adapt to the fast pace of current clinical developments. In addition, we need to continue to engage, validate, and facilitate the wide adoption of newly developed biomarkers, prioritizing their predictive ability, cost-efficiency, and generalizability. In summary, what seems clear is the need to integrate and understand the extent of all the data obtained in each patient. Combining clinical features, radiologic images and molecular markers will probably pave the road for further survival improvements in NSCLC patients.
Acknowledgments
Funding: None.
Footnote
Provenance and Peer Review: This article was commissioned by the Guest Editor (Witold Rzyman) for the series “Implementation of CT-based screening of lung cancer” published in Translational Lung Cancer Research. The article was sent for external peer review organized by the Guest Editor and the editorial office.
Conflicts of Interest: All authors have completed the ICMJE uniform disclosure form (available at http://dx.doi.org/10.21037/tlcr-20-750). The series “Implementation of CT-based screening of lung cancer” was commissioned by the editorial office without any funding or sponsorship. LMM serves as an unpaid editorial board member of Translational Lung Cancer Research from Sep 2019 to Sep 2021. DA reports other, during the conduct of the study. LMS reports personal fees from Sabartech, personal fees from Astra Zeneca, personal fees from Esteve, personal fees from Chiesi, outside the submitted work. RP has two patents licensed to Amadix: new lung cancer molecular markers, and in vitro method for the diagnosis of lung cancer. JJZ reports other from VisionGate, Inc., outside the submitted work. In addition, Dr. LMM has a patent: complement fragments for the diagnosis of lung cancer licensed. The authors have no other conflicts of interest to declare.
Ethical Statement: The authors are accountable for all aspects of the work in ensuring that questions related to the accuracy or integrity of any part of the work are appropriately investigated and resolved.
Open Access Statement: This is an Open Access article distributed in accordance with the Creative Commons Attribution-NonCommercial-NoDerivs 4.0 International License (CC BY-NC-ND 4.0), which permits the non-commercial replication and distribution of the article with the strict proviso that no changes or edits are made and the original work is properly cited (including links to both the formal publication through the relevant DOI and the license). See: https://creativecommons.org/licenses/by-nc-nd/4.0/.
References
- Al-Ayoubi AM, Flores RM. Management of CT screen-detected lung nodule: the thoracic surgeon perspective. Ann Transl Med 2016;4:156. [Crossref] [PubMed]
- Henschke CI, Yankelevitz DF, Libby DM, et al. Survival of patients with stage I lung cancer detected on CT screening. N Engl J Med 2006;355:1763-71. [Crossref] [PubMed]
- de Koning HJ, van der Aalst CM, de Jong PA, et al. Reduced lung-cancer mortality with volume CT screening in a randomized trial. N Engl J Med 2020;382:503-13. [Crossref] [PubMed]
- Pastorino U, Silva M, Sestini S, et al. Prolonged lung cancer screening reduced 10-year mortality in the MILD trial: new confirmation of lung cancer screening efficacy. Ann Oncol 2019;30:1162-9. [Crossref] [PubMed]
- Aberle DR, Adams AM, Berg CD, et al. Reduced lung-cancer mortality with low-dose computed tomographic screening. N Engl J Med 2011;365:395-409. [Crossref] [PubMed]
- Sanchez-Salcedo P, Wilson DO, de-Torres JP, et al. Improving selection criteria for lung cancer screening. The potential role of emphysema. Am J Respir Crit Care Med 2015;191:924-31. [Crossref] [PubMed]
- Pinsky PF, Berg CD. Applying the National Lung Screening Trial eligibility criteria to the US population: what percent of the population and of incident lung cancers would be covered? J Med Screen 2012;19:154-6. [Crossref] [PubMed]
- Wood DE. Point: Should lung cancer screening be expanded to persons who don’t currently meet accepted criteria set forth by the CHEST Guidelines on Lung Cancer Screening? Yes. Chest 2018;153:1299-302. [Crossref] [PubMed]
- Denton E, Conron M. Improving outcomes in lung cancer: the value of the multidisciplinary health care team. J Multidiscip Healthc 2016;9:137-44. [PubMed]
- Leo F, Venissac N, Poudenx M, et al. Multidisciplinary management of lung cancer: how to test its efficacy? J Thorac Oncol 2007;2:69-72. [Crossref] [PubMed]
- Cassidy A, Myles JP, van Tongeren M, et al. The LLP risk model: an individual risk prediction model for lung cancer. Br J Cancer 2008;98:270-6. [Crossref] [PubMed]
- Tammemagi CM, Pinsky PF, Caporaso NE, et al. Lung cancer risk prediction: prostate, lung, colorectal and ovarian cancer screening trial models and validation. J Natl Cancer Inst 2011;103:1058-68. [Crossref] [PubMed]
- Swensen SJ, Silverstein MD, Ilstrup DM, et al. The probability of malignancy in solitary pulmonary nodules. Application to small radiologically indeterminate nodules. Arch Intern Med 1997;157:849-55. [Crossref] [PubMed]
- Yonemori K, Tateishi U, Uno H, et al. Development and validation of diagnostic prediction model for solitary pulmonary nodules. Respirology 2007;12:856-62. [Crossref] [PubMed]
- Cutler SJ, Loveland DB. The risk of developing lung cancer and its relationship to smoking. J Natl Cancer Inst 1954;15:201-11. [PubMed]
- Neifeld JP, Michaelis LL, Doppman JL. Suspected pulmonary metastases. Correlation of chest X-ray, whole lung tomograms, and operative findings. Cancer 1977;39:383-7. [Crossref] [PubMed]
- de-Torres JP, Marín JM, Casanova C, et al. Identification of COPD patients at high risk for lung cancer mortality using the COPD-LUCSS-DLCO. Chest 2016;149:936-42. [Crossref] [PubMed]
- Lin H, Huang YS, Yan HH, et al. A family history of cancer and lung cancer risk in never-smokers: a clinic-based case-control study. Lung Cancer 2015;89:94-8. [Crossref] [PubMed]
- Weller DP, Peake MD, Field JK. Presentation of lung cancer in primary care. NPJ Prim Care Respir Med 2019;29:21. [Crossref] [PubMed]
- Henschke CI, Yankelevitz DF, Yip R, et al. Lung cancers diagnosed at annual CT screening: volume doubling times. Radiology 2012;263:578-83. [Crossref] [PubMed]
- Seijo LM, Peled N, Ajona D, et al. Biomarkers in lung cancer screening: achievements, promises, and challenges. J Thorac Oncol 2019;14:343-57. [Crossref] [PubMed]
- Massion PP, Antic S, Ather S, et al. Assessing the accuracy of a deep learning method to risk stratify indeterminate pulmonary nodules. Am J Respir Crit Care Med 2020;202:241-9. [Crossref] [PubMed]
- Vachani A, Whitney DH, Parsons EC, et al. Clinical utility of a bronchial genomic classifier in patients with suspected lung cancer. Chest 2016;150:210-8. [Crossref] [PubMed]
- Thawani R, McLane M, Beig N, et al. Radiomics and radiogenomics in lung cancer: A review for the clinician. Lung Cancer 2018;115:34-41. [Crossref] [PubMed]
- Lambin P, Rios-Velazquez E, Leijenaar R, et al. Radiomics: extracting more information from medical images using advanced feature analysis. Eur J Cancer 2012;48:441-6. [Crossref] [PubMed]
- Thawani R, Mustafa SA. The future of radiomics in lung cancer. Lancet Digit Health 2020;2:e103. [Crossref] [PubMed]
- Garau N, Paganelli C, Summers P, et al. External validation of radiomics-based predictive models in low-dose CT screening for early lung cancer diagnosis. Med Phys 2020;47:4125-36. [Crossref] [PubMed]
- Gillies RJ, Schabath MB. Radiomics improves cancer screening and early detection. Cancer Epidemiol Biomarkers Prev 2020;29:2556-67. [Crossref] [PubMed]
- Fornacon-Wood I, Faivre-Finn C, O'Connor JPB, et al. Radiomics as a personalized medicine tool in lung cancer: separating the hope from the hype. Lung Cancer 2020;146:197-208. [Crossref] [PubMed]
- Lo Gullo R, Daimiel I, Morris EA, et al. Combining molecular and imaging metrics in cancer: radiogenomics. Insights Imaging 2020;11:1. [Crossref] [PubMed]
- Bai HX, Lee AM, Yang L, et al. Imaging genomics in cancer research: limitations and promises. Br J Radiol 2016;89:20151030. [Crossref] [PubMed]
- Kwak EL, Bang YJ, Camidge DR, et al. Anaplastic lymphoma kinase inhibition in non-small-cell lung cancer. N Engl J Med 2010;363:1693-703. [Crossref] [PubMed]
- Glynn C, Zakowski MF, Ginsberg MS. Are there imaging characteristics associated with epidermal growth factor receptor and KRAS mutations in patients with adenocarcinoma of the lung with bronchioloalveolar features? J Thorac Oncol 2010;5:344-8. [Crossref] [PubMed]
- Lee Y, Lee HJ, Kim YT, et al. Imaging characteristics of stage I non-small cell lung cancer on CT and FDG-PET: relationship with epidermal growth factor receptor protein expression status and survival. Korean J Radiol 2013;14:375-83. [Crossref] [PubMed]
- Lee HJ, Kim YT, Kang CH, et al. Epidermal growth factor receptor mutation in lung adenocarcinomas: relationship with CT characteristics and histologic subtypes. Radiology 2013;268:254-64. [Crossref] [PubMed]
- Yano M, Sasaki H, Kobayashi Y, et al. Epidermal growth factor receptor gene mutation and computed tomographic findings in peripheral pulmonary adenocarcinoma. J Thorac Oncol 2006;1:413-6. [Crossref] [PubMed]
- Rizzo S, Petrella F, Buscarino V, et al. CT radiogenomic characterization of EGFR, K-RAS, and ALK mutations in non-small cell lung cancer. Eur Radiol 2016;26:32-42. [Crossref] [PubMed]
- Halpenny DF, Riely GJ, Hayes S, et al. Are there imaging characteristics associated with lung adenocarcinomas harboring ALK rearrangements? Lung Cancer 2014;86:190-4. [Crossref] [PubMed]
- Plodkowski AJ, Drilon A, Halpenny DF, et al. From genotype to phenotype: are there imaging characteristics associated with lung adenocarcinomas harboring RET and ROS1 rearrangements? Lung Cancer 2015;90:321-5. [Crossref] [PubMed]
- Nair VS, Gevaert O, Davidzon G, et al. Prognostic PET 18F-FDG uptake imaging features are associated with major oncogenomic alterations in patients with resected non-small cell lung cancer. Cancer Res 2012;72:3725-34. [Crossref] [PubMed]
- Vaidya P, Bera K, Gupta A, et al. CT derived radiomic score for predicting the added benefit of adjuvant chemotherapy following surgery in stage I, II resectable non-small cell lung cancer: a retrospective multicohort study for outcome prediction. Lancet Digit Health 2020;2:e116-28. [Crossref] [PubMed]
- Pastorino U. Lung cancer screening. Br J Cancer 2010;102:1681-6. [Crossref] [PubMed]
- Mesa-Guzmán M, González J, Alcaide AB, et al. Surgical outcomes in a lung cancer-screening program using low dose computed tomography. Arch Bronconeumol 2021;57:101-6. [PubMed]
- Ginsberg RJ, Rubinstein LV. Randomized trial of lobectomy versus limited resection for T1 N0 non-small cell lung cancer. Lung Cancer Study Group. Ann Thorac Surg 1995;60:615-22; discussion 622-3. [Crossref] [PubMed]
- Altorki NK, Wang X, Wigle D, et al. Perioperative mortality and morbidity after sublobar versus lobar resection for early-stage non-small-cell lung cancer: post-hoc analysis of an international, randomised, phase 3 trial (CALGB/Alliance 140503). Lancet Respir Med 2018;6:915-24. [Crossref] [PubMed]
- Cardillo G, Regal M, Sera F, et al. Videothoracoscopic management of the solitary pulmonary nodule: a single-institution study on 429 cases. Ann Thorac Surg 2003;75:1607-11. [Crossref] [PubMed]
- Smith MA, Battafarano RJ, Meyers BF, et al. Prevalence of benign disease in patients undergoing resection for suspected lung cancer. Ann Thorac Surg 2006;81:1824-8; discussion 1828-9. [Crossref] [PubMed]
- Zaman M, Bilal H, Woo CY, et al. In patients undergoing video-assisted thoracoscopic surgery excision, what is the best way to locate a subcentimetre solitary pulmonary nodule in order to achieve successful excision? Interact Cardiovasc Thorac Surg 2012;15:266-72. [Crossref] [PubMed]
- Petersen RH, Hansen HJ, Dirksen A, et al. Lung cancer screening and video-assisted thoracic surgery. J Thorac Oncol 2012;7:1026-31. [Crossref] [PubMed]
- Marchevsky AM, Changsri C, Gupta I, et al. Frozen section diagnoses of small pulmonary nodules: accuracy and clinical implications. Ann Thorac Surg 2004;78:1755-9. [Crossref] [PubMed]
- Lozano MD, Echeveste JI, Abengozar M, et al. Cytology smears in the era of molecular biomarkers in non-small cell lung cancer: doing more with less. Arch Pathol Lab Med 2018;142:291-8. [Crossref] [PubMed]
- Wu YL, Tsuboi M, He J, et al. Osimertinib in Resected EGFR-Mutated Non-Small-Cell Lung Cancer. N Engl J Med 2020;383:1711-23. [Crossref] [PubMed]
- Planchard D. Adjuvant osimertinib in EGFR-mutated non-small-cell lung cancer. N Engl J Med 2020;383:1780-2. [Crossref] [PubMed]
- Sozzi G, Boeri M. Potential biomarkers for lung cancer screening. Transl Lung Cancer Res 2014;3:139-48. [PubMed]
- Mazzone PJ, Sears CR, Arenberg DA, et al. Evaluating molecular biomarkers for the early detection of lung cancer: when is a biomarker ready for clinical use? An official American Thoracic Society policy statement. Am J Respir Crit Care Med 2017;196:e15-29. [Crossref] [PubMed]
- Mehta A, Cordero J, Dobersch S, et al. Non-invasive lung cancer diagnosis by detection of GATA6 and NKX2-1 isoforms in exhaled breath condensate. EMBO Mol Med 2016;8:1380-9. [Crossref] [PubMed]
- Marzorati D, Mainardi L, Sedda G, et al. A review of exhaled breath: a key role in lung cancer diagnosis. J Breath Res 2019;13:034001. [Crossref] [PubMed]
- Bel'skaya LV, Sarf EA, Kosenok VK, et al. Biochemical markers of saliva in lung cancer: diagnostic and prognostic perspectives. Diagnostics (Basel) 2020;10:186. [Crossref] [PubMed]
- Mathé EA, Patterson AD, Haznadar M, et al. Noninvasive urinary metabolomic profiling identifies diagnostic and prognostic markers in lung cancer. Cancer Res 2014;74:3259-70. [Crossref] [PubMed]
- Ostrin EJ, Sidransky D, Spira A, et al. Biomarkers for lung cancer screening and detection. Cancer Epidemiol Biomarkers Prev 2020;29:2411-5. [Crossref] [PubMed]
- Sears CR, Mazzone PJ. Biomarkers in lung cancer. Clin Chest Med 2020;41:115-27. [Crossref] [PubMed]
- Mazzone PJ, Wang XF, Han X, et al. Evaluation of a serum lung cancer biomarker panel. Biomark Insights 2018;13:1177271917751608. [Crossref] [PubMed]
- Silvestri GA, Tanner NT, Kearney P, et al. Assessment of plasma proteomics biomarker’s ability to distinguish benign from malignant lung nodules: results of the PANOPTIC (Pulmonary Nodule plasma proteomic classifier) trial. Chest 2018;154:491-500. [Crossref] [PubMed]
- Chapman CJ, Healey GF, Murray A, et al. EarlyCDT®-Lung test: improved clinical utility through additional autoantibody assays. Tumour Biol 2012;33:1319-26. [Crossref] [PubMed]
- Healey GF, Lam S, Boyle P, et al. Signal stratification of autoantibody levels in serum samples and its application to the early detection of lung cancer. J Thorac Dis 2013;5:618-25. [PubMed]
- Edelsberg J, Weycker D, Atwood M, et al. Cost-effectiveness of an autoantibody test (EarlyCDT-Lung) as an aid to early diagnosis of lung cancer in patients with incidentally detected pulmonary nodules. PLoS One 2018;13:e0197826. [Crossref] [PubMed]
- Lastwika KJ, Kargl J, Zhang Y, et al. Tumor-derived autoantibodies identify malignant pulmonary nodules. Am J Respir Crit Care Med 2019;199:1257-66. [Crossref] [PubMed]
- Liu L, Teng J, Zhang L, et al. The combination of the tumor markers suggests the histological diagnosis of lung cancer. Biomed Res Int 2017;2017:2013989. [Crossref] [PubMed]
- Ajona D, Pajares MJ, Corrales L, et al. Investigation of complement activation product c4d as a diagnostic and prognostic biomarker for lung cancer. J Natl Cancer Inst 2013;105:1385-93. [Crossref] [PubMed]
- Ajona D, Okrój M, Pajares MJ, et al. Complement C4d-specific antibodies for the diagnosis of lung cancer. Oncotarget 2017;9:6346-55. [Crossref] [PubMed]
- Sozzi G, Boeri M, Rossi M, et al. Clinical utility of a plasma-based miRNA signature classifier within computed tomography lung cancer screening: a correlative MILD trial study. J Clin Oncol 2014;32:768-73. [Crossref] [PubMed]
- Boeri M, Verri C, Conte D, et al. MicroRNA signatures in tissues and plasma predict development and prognosis of computed tomography detected lung cancer. Proc Natl Acad Sci U S A 2011;108:3713-8. [Crossref] [PubMed]
- Filippo L, Principe R, Cesario A, et al. Smoking cessation intervention within the framework of a lung cancer screening program: preliminary results and clinical perspectives from the "Cosmos-II" Trial. Lung 2015;193:147-9. [Crossref] [PubMed]
- Bianchi F, Nicassio F, Marzi M, et al. A serum circulating miRNA diagnostic test to identify asymptomatic high-risk individuals with early stage lung cancer. EMBO Mol Med 2011;3:495-503. [Crossref] [PubMed]
- Montani F, Marzi MJ, Dezi F, et al. miR-Test: a blood test for lung cancer early detection. J Natl Cancer Inst 2015;107:djv063. [Crossref] [PubMed]
- Maisonneuve P, Bagnardi V, Bellomi M, et al. Lung cancer risk prediction to select smokers for screening CT—a model based on the Italian COSMOS trial. Cancer Prev Res (Phila) 2011;4:1778-89. [Crossref] [PubMed]
- Yanaihara N, Caplen N, Bowman E, et al. Unique microRNA molecular profiles in lung cancer diagnosis and prognosis. Cancer Cell 2006;9:189-98. [Crossref] [PubMed]
- Fehlmann T, Kahraman M, Ludwig N, et al. Evaluating the use of circulating microRNA profiles for lung cancer detection in symptomatic patients. JAMA Oncol 2020;6:714-23. [Crossref] [PubMed]
- Weiss G, Schlegel A, Kottwitz D, et al. Validation of the SHOX2/PTGER4 DNA methylation marker panel for plasma-based discrimination between patients with malignant and nonmalignant lung disease. J Thorac Oncol 2017;12:77-84. [Crossref] [PubMed]
- Chen X, Gole J, Gore A, et al. Non-invasive early detection of cancer four years before conventional diagnosis using a blood test. Nat Commun 2020;11:3475. [Crossref] [PubMed]
- Abbosh C, Birkbak NJ, Swanton C. Early stage NSCLC - challenges to implementing ctDNA-based screening and MRD detection. Nat Rev Clin Oncol 2018;15:577-86. [Crossref] [PubMed]
- Aravanis AM, Lee M, Klausner RD. Next-generation sequencing of circulating tumor DNA for early cancer detection. Cell 2017;168:571-4. [Crossref] [PubMed]
- Newman AM, Bratman SV, To J, et al. An ultrasensitive method for quantitating circulating tumor DNA with broad patient coverage. Nat Med 2014;20:548-54. [Crossref] [PubMed]
- Chabon JJ, Hamilton EG, Kurtz DM, et al. Integrating genomic features for non-invasive early lung cancer detection. Nature 2020;580:245-51. [Crossref] [PubMed]
- Guo N, Lou F, Ma Y, et al. Circulating tumor DNA detection in lung cancer patients before and after surgery. Sci Rep 2016;6:33519. [Crossref] [PubMed]
- Phallen J, Sausen M, Adleff V, et al. Direct detection of early-stage cancers using circulating tumor DNA. Sci Transl Med 2017;9:eaan2415. [Crossref] [PubMed]
- Showe MK, Vachani A, Kossenkov AV, et al. Gene expression profiles in peripheral blood mononuclear cells can distinguish patients with non-small cell lung cancer from patients with nonmalignant lung disease. Cancer Res 2009;69:9202-10. [Crossref] [PubMed]
- Cohen JD, Li L, Wang Y, et al. Detection and localization of surgically resectable cancers with a multi-analyte blood test. Science 2018;359:926-30. [Crossref] [PubMed]
- Cristiano S, Leal A, Phallen J, et al. Genome-wide cell-free DNA fragmentation in patients with cancer. Nature 2019;570:385-9. [Crossref] [PubMed]
- Nichols JAA, Grob P, de Lusignan S, et al. Genetic test to stop smoking (GeTSS) trial protocol: randomised controlled trial of a genetic test (Respiragene) and Auckland formula to assess lung cancer risk. BMC Pulm Med 2014;14:77. [Crossref] [PubMed]
- Zhang C, Yu W, Wang L, et al. DNA methylation analysis of the SHOX2 and RASSF1A panel in bronchoalveolar lavage fluid for lung cancer diagnosis. J Cancer 2017;8:3585-91. [Crossref] [PubMed]
- Xing L, Su J, Guarnera MA, et al. Sputum microRNA biomarkers for identifying lung cancer in indeterminate solitary pulmonary nodules. Clin Cancer Res 2015;21:484-9. [Crossref] [PubMed]
- Halling KC, Rickman OB, Kipp BR, et al. A comparison of cytology and fluorescence in situ hybridization for the detection of lung cancer in bronchoscopic specimens. Chest 2006;130:694-701. [Crossref] [PubMed]
- Subramanian H, Viswanathan P, Cherkezyan L, et al. Procedures for risk-stratification of lung cancer using buccal nanocytology. Biomed Opt Express 2016;7:3795-810. [Crossref] [PubMed]
- Patriquin L, Merrick DT, Hill D, et al. Early detection of lung cancer with meso tetra (4-carboxyphenyl) porphyrin-labeled sputum. J Thorac Oncol 2015;10:1311-8. [Crossref] [PubMed]
- Billatos E, Duan F, Moses E, et al. Detection of early lung cancer among military personnel (DECAMP) consortium: study protocols. BMC Pulm Med 2019;19:59. [Crossref] [PubMed]
- Molina-Vila MA, Mayo-de-Las-Casas C, Giménez-Capitán A, et al. Liquid biopsy in non-small cell lung cancer. Front Med (Lausanne) 2016;3:69. [Crossref] [PubMed]
- Massion PP, Healey GF, Peek LJ, et al. Autoantibody signature enhances the positive predictive power of computed tomography and nodule-based risk models for detection of lung cancer. J Thorac Oncol 2017;12:578-84. [Crossref] [PubMed]
- Boyle P, Chapman CJ, Holdenrieder S, et al. Clinical validation of an autoantibody test for lung cancer. Ann Oncol 2011;22:383-9. [Crossref] [PubMed]
- Sullivan FM, Farmer E, Mair FS, et al. Detection in blood of autoantibodies to tumour antigens as a case-finding method in lung cancer using the EarlyCDT®-Lung Test (ECLS): study protocol for a randomized controlled trial. BMC Cancer 2017;17:187. [Crossref] [PubMed]
- Doseeva V, Colpitts T, Gao G, et al. Performance of a multiplexed dual analyte immunoassay for the early detection of non-small cell lung cancer. J Transl Med 2015;13:55. [Crossref] [PubMed]
- Lam S, Boyle P, Healey GF, et al. EarlyCDT-Lung: an immunobiomarker test as an aid to early detection of lung cancer. Cancer Prev Res (Phila) 2011;4:1126-34. [Crossref] [PubMed]
- Sullivan FM, Mair FS, Anderson W, et al. Earlier diagnosis of lung cancer in a randomised trial of an autoantibody blood test followed by imaging. Eur Respir J 2021;57:2000670. [Crossref] [PubMed]
- Li XJ, Hayward C, Fong PY, et al. A blood-based proteomic classifier for the molecular characterization of pulmonary nodules. Sci Transl Med 2013;5:207ra142. [Crossref] [PubMed]
- Vachani A, Pass HI, Rom WN, et al. Validation of a multiprotein plasma classifier to identify benign lung nodules. J Thorac Oncol 2015;10:629-37. [Crossref] [PubMed]
- Ajona D, Razquin C, Pastor MD, et al. Elevated levels of the complement activation product C4d in bronchial fluids for the diagnosis of lung cancer. PLoS One 2015;10:e0119878. [Crossref] [PubMed]
- Kalluri R. The biology and function of exosomes in cancer. J Clin Invest 2016;126:1208-15. [Crossref] [PubMed]
- Frydrychowicz M, Kolecka-Bednarczyk A, Madejczyk M, et al. Exosomes - structure, biogenesis and biological role in non-small-cell lung cancer. Scand J Immunol 2015;81:2-10. [Crossref] [PubMed]
- Zhao Q, Cao J, Wu YC, et al. Circulating miRNAs is a potential marker for gefitinib sensitivity and correlation with EGFR mutational status in human lung cancers. Am J Cancer Res 2015;5:1692-705. [PubMed]
- Jin X, Chen Y, Chen H, et al. Evaluation of tumor-derived exosomal miRNA as potential diagnostic biomarkers for early-stage non-small cell lung cancer using next-generation sequencing. Clin Cancer Res 2017;23:5311-9. [Crossref] [PubMed]
- Rabinowits G, Gerçel-Taylor C, Day JM, et al. Exosomal microRNA: a diagnostic marker for lung cancer. Clin Lung Cancer 2009;10:42-6. [Crossref] [PubMed]
- Wu H, Zhou J, Mei S, et al. Circulating exosomal microRNA-96 promotes cell proliferation, migration and drug resistance by targeting LMO7. J Cell Mol Med 2017;21:1228-36. [Crossref] [PubMed]
- Zhou X, Wen W, Shan X, et al. A six-microRNA panel in plasma was identified as a potential biomarker for lung adenocarcinoma diagnosis. Oncotarget 2017;8:6513-25. [Crossref] [PubMed]
- Cazzoli R, Buttitta F, Di Nicola M, et al. microRNAs derived from circulating exosomes as noninvasive biomarkers for screening and diagnosing lung cancer. J Thorac Oncol 2013;8:1156-62. [Crossref] [PubMed]
- Aushev VN, Zborovskaya IB, Laktionov KK, et al. Comparisons of microRNA patterns in plasma before and after tumor removal reveal new biomarkers of lung squamous cell carcinoma. PLoS One 2013;8:e78649. [Crossref] [PubMed]
- Liu Q, Yu Z, Yuan S, et al. Circulating exosomal microRNAs as prognostic biomarkers for non-small-cell lung cancer. Oncotarget 2017;8:13048-58. [Crossref] [PubMed]
- Liu F, Vermesh O, Mani V, et al. The exosome total isolation chip. ACS Nano 2017;11:10712-23. [Crossref] [PubMed]
- Rodríguez M, Silva J, López-Alfonso A, et al. Different exosome cargo from plasma/bronchoalveolar lavage in non-small-cell lung cancer. Genes Chromosomes Cancer 2014;53:713-24. [Crossref] [PubMed]
- Wang Y, Xu YM, Zou YQ, et al. Identification of differential expressed PE exosomal miRNA in lung adenocarcinoma, tuberculosis, and other benign lesions. Medicine (Baltimore) 2017;96:e8361. [Crossref] [PubMed]
- Lin J, Wang Y, Zou YQ, et al. Differential miRNA expression in pleural effusions derived from extracellular vesicles of patients with lung cancer, pulmonary tuberculosis, or pneumonia. Tumour Biol 2016;37:15835-45. [Crossref] [PubMed]
- Zhang R, Xia Y, Wang Z, et al. Serum long non coding RNA MALAT-1 protected by exosomes is up-regulated and promotes cell proliferation and migration in non-small cell lung cancer. Biochem Biophys Res Commun 2017;490:406-14. [Crossref] [PubMed]
- Kalluri R, LeBleu VS. Discovery of double-stranded genomic DNA in circulating exosomes. Cold Spring Harb Symp Quant Biol 2016;81:275-80. [Crossref] [PubMed]
- Yang J, Wei F, Schafer C, et al. Detection of tumor cell-specific mRNA and protein in exosome-like microvesicles from blood and saliva. PLoS One 2014;9:e110641. [Crossref] [PubMed]
- Sandfeld-Paulsen B, Jakobsen KR, Bæk R, et al. Exosomal proteins as diagnostic biomarkers in lung cancer. J Thorac Oncol 2016;11:1701-10. [Crossref] [PubMed]
- Vykoukal J, Sun N, Aguilar-Bonavides C, et al. Plasma-derived extracellular vesicle proteins as a source of biomarkers for lung adenocarcinoma. Oncotarget 2017;8:95466. [Crossref] [PubMed]
- Gao J, Qiu X, Li X, et al. Expression profiles and clinical value of plasma exosomal Tim-3 and Galectin-9 in non-small cell lung cancer. Biochem Biophys Res Commun 2018;498:409-15. [Crossref] [PubMed]
- Sun Y, Huo C, Qiao Z, et al. Comparative proteomic analysis of exosomes and microvesicles in human saliva for lung cancer. J Proteome Res 2018;17:1101-7. [Crossref] [PubMed]
- Li Y, Zhang Y, Qiu F, et al. Proteomic identification of exosomal LRG1: a potential urinary biomarker for detecting NSCLC. Electrophoresis 2011;32:1976-83. [Crossref] [PubMed]
- Park JO, Choi DY, Choi DS, et al. Identification and characterization of proteins isolated from microvesicles derived from human lung cancer pleural effusions. Proteomics 2013;13:2125-34. [Crossref] [PubMed]
- Fan TWM, Zhang X, Wang C, et al. Exosomal lipids for classifying early and late stage non-small cell lung cancer. Anal Chim Acta 2018;1037:256-64. [Crossref] [PubMed]
- Yoshizawa A, Motoi N, Riely GJ, et al. Impact of proposed IASLC/ATS/ERS classification of lung adenocarcinoma: prognostic subgroups and implications for further revision of staging based on analysis of 514 stage I cases. Mod Pathol 2011;24:653-64. [Crossref] [PubMed]
- Chen T, Luo J, Gu H, et al. Should minimally invasive lung adenocarcinoma be transferred from stage IA1 to stage 0 in future updates of the TNM staging system? J Thorac Dis 2018;10:6247-53. [Crossref] [PubMed]
- Behera M, Owonikoko TK, Gal AA, et al. Lung adenocarcinoma staging using the 2011 IASLC/ATS/ERS classification: a pooled analysis of adenocarcinoma in situ and minimally invasive adenocarcinoma. Clin Lung Cancer 2016;17:e57-64. [Crossref] [PubMed]
- Kadota K, Yeh YC, Sima CS, et al. The cribriform pattern identifies a subset of acinar predominant tumors with poor prognosis in patients with stage I lung adenocarcinoma: a conceptual proposal to classify cribriform predominant tumors as a distinct histologic subtype. Mod Pathol 2014;27:690-700. [Crossref] [PubMed]
- Kuang M, Shen X, Yuan C, et al. Clinical significance of complex glandular patterns in lung adenocarcinoma: clinicopathologic and molecular study in a large series of cases. Am J Clin Pathol 2018;150:65-73. [Crossref] [PubMed]
- Kadota K, Nitadori JI, Woo KM, et al. Comprehensive pathological analyses in lung squamous cell carcinoma: single cell invasion, nuclear diameter, and tumor budding are independent prognostic factors for worse outcomes. J Thorac Oncol 2014;9:1126-39. [Crossref] [PubMed]
- Mollberg NM, Bennette C, Howell E, et al. Lymphovascular invasion as a prognostic indicator in stage I non-small cell lung cancer: a systematic review and meta-analysis. Ann Thorac Surg 2014;97:965-71. [Crossref] [PubMed]
- Wen S, Zhou W, Li CM, et al. Ki-67 as a prognostic marker in early-stage non-small cell lung cancer in Asian patients: a meta-analysis of published studies involving 32 studies. BMC Cancer 2015;15:520. [Crossref] [PubMed]
- Tuminello S, Veluswamy R, Lieberman-Cribbin W, et al. Prognostic value of immune cells in the tumor microenvironment of early-stage lung cancer: a meta-analysis. Oncotarget 2019;10:7142-55. [Crossref] [PubMed]
- Burotto M, Thomas A, Subramaniam D, et al. Biomarkers in early-stage non-small-cell lung cancer: current concepts and future directions. J Thorac Oncol 2014;9:1609-17. [Crossref] [PubMed]
- Hernández-Prieto S, Romera A, Ferrer M, et al. A 50-gene signature is a novel scoring system for tumor-infiltrating immune cells with strong correlation with clinical outcome of stage I/II non-small cell lung cancer. Clin Transl Oncol 2015;17:330-8. [Crossref] [PubMed]
- Hofman P, Badoual C, Henderson F, et al. Multiplexed immunohistochemistry for molecular and immune profiling in lung cancer—just about ready for prime-time? Cancers (Basel) 2019;11:283. [Crossref] [PubMed]
- Kadota K, Nitadori JI, Sima CS, et al. Tumor spread through air spaces is an important pattern of invasion and impacts the frequency and location of recurrences after limited resection for small stage I lung adenocarcinomas. J Thorac Oncol 2015;10:806-14. [Crossref] [PubMed]
- Eguchi T, Kameda K, Lu S, et al. Lobectomy is associated with better outcomes than sublobar resection in spread through air spaces (STAS)-positive t1 lung adenocarcinoma: a propensity score-matched analysis. J Thorac Oncol 2019;14:87-98. [Crossref] [PubMed]
- Travis W, Aly R, Eguchi T, et al. ES12. 05 Impact of STAS in lung cancer staging. J Thorac Oncol 2019;14:S45. [Crossref]
- Xie Y, Xiao G, Coombes KR, et al. Robust gene expression signature from formalin-fixed paraffin-embedded samples predicts prognosis of non-small-cell lung cancer patients. Clin Cancer Res 2011;17:5705-14. [Crossref] [PubMed]
- Kratz JR, He J, Van Den Eeden SK, et al. A practical molecular assay to predict survival in resected non-squamous, non-small-cell lung cancer: development and international validation studies. Lancet 2012;379:823-32. [Crossref] [PubMed]
- Kratz JR, Tham PT, Mulvihill MS, et al. Analytical validation of a practical molecular assay prognostic of survival in nonsquamous non-small cell lung cancer. Diagn Mol Pathol 2013;22:65-9. [Crossref] [PubMed]
- Kratz JR, Haro GJ, Cook NR, et al. Incorporation of a molecular prognostic classifier improves conventional non-small cell lung cancer staging. J Thorac Oncol 2019;14:1223-32. [Crossref] [PubMed]
- Wistuba II, Behrens C, Lombardi F, et al. Validation of a proliferation-based expression signature as prognostic marker in early stage lung adenocarcinoma. Clin Cancer Res 2013;19:6261-71. [Crossref] [PubMed]
- Dama E, Melocchi V, Dezi F, et al. An aggressive subtype of stage I lung adenocarcinoma with molecular and prognostic characteristics typical of advanced lung cancers. Clin Cancer Res 2017;23:62-72. [Crossref] [PubMed]
- Martínez-Terroba E, Behrens C, Agorreta J, et al. 5 protein-based signature for resectable lung squamous cell carcinoma improves the prognostic performance of the TNM staging. Thorax 2019;74:371-9. [Crossref] [PubMed]
- Martínez-Terroba E, Behrens C, de Miguel FJ, et al. A novel protein-based prognostic signature improves risk stratification to guide clinical management in early-stage lung adenocarcinoma patients. J Pathol 2018;245:421-32. [Crossref] [PubMed]
- McCourt CM, Boyle D, James J, et al. Immunohistochemistry in the era of personalised medicine. J Clin Pathol 2013;66:58-61. [Crossref] [PubMed]
- Li Y, McGrail DJ, Xu J, et al. MERIT: Systematic analysis and characterization of Mutational Effect on RNA Interactome Topology. Hepatology 2019;70:532-46. [Crossref] [PubMed]
- Liu C, Li Y, Wei M, et al. Identification of a novel glycolysis-related gene signature that can predict the survival of patients with lung adenocarcinoma. Cell Cycle 2019;18:568-79. [Crossref] [PubMed]
- Jin BF, Yang F, Ying XM, et al. Signaling protein signature predicts clinical outcome of non-small-cell lung cancer. BMC Cancer 2018;18:259. [Crossref] [PubMed]
- Luo X, Zang X, Yang L, et al. Comprehensive computational pathological image analysis predicts lung cancer prognosis. J Thorac Oncol 2017;12:501-9. [Crossref] [PubMed]
- Wang S, Wang T, Yang L, et al. ConvPath: A software tool for lung adenocarcinoma digital pathological image analysis aided by a convolutional neural network. EBioMedicine 2019;50:103-10. [Crossref] [PubMed]
- Ettinger DS, Wood DE, Aisner DL, et al. Non-small cell lung cancer, version 5.2017, NCCN clinical practice guidelines in oncology. J Natl Compr Canc Netw 2017;15:504-35. [Crossref] [PubMed]
- Ramnath N, Dilling TJ, Harris LJ, et al. Treatment of stage III non-small cell lung cancer: diagnosis and management of lung cancer, 3rd ed: American College of Chest Physicians Evidence-Based Clinical Practice Guidelines. Chest 2013;143:e314S-40S.
- Howington JA, Blum MG, Chang AC, et al. Treatment of stage I and II non-small cell lung cancer: diagnosis and management of lung cancer, 3rd ed: American College of Chest Physicians Evidence-Based Clinical Practice Guidelines. Chest 2013;143:e278S-313S.
- Camps C, Felip E, García-Campelo R, et al. SEOM clinical guidelines for the treatment of non-small cell lung cancer (NSCLC) 2013. Clin Transl Oncol 2013;15:977-84. [Crossref] [PubMed]
- Artal Cortés Á, Calera Urquizu L, Hernando Cubero J. Adjuvant chemotherapy in non-small cell lung cancer: state-of-the-art. Transl Lung Cancer Res 2015;4:191-7. [PubMed]
- Scagliotti GV, Fossati R, Torri V, et al. Randomized study of adjuvant chemotherapy for completely resected stage I, II, or IIIA non-small-cell lung cancer. J Natl Cancer Inst 2003;95:1453-61. [Crossref] [PubMed]
- Waller D, Peake MD, Stephens RJ, et al. Chemotherapy for patients with non-small cell lung cancer: the surgical setting of the Big Lung Trial. Eur J Cardiothorac Surg 2004;26:173-82. [Crossref] [PubMed]
- Strauss GM, Herndon JE 2nd, Maddaus MA, et al. Adjuvant paclitaxel plus carboplatin compared with observation in stage IB non-small-cell lung cancer: CALGB 9633 with the Cancer and Leukemia Group B, Radiation Therapy Oncology Group, and North Central Cancer Treatment Group Study Groups. J Clin Oncol 2008;26:5043-51. [Crossref] [PubMed]
- Arriagada R. The international adjuvant lung cancer trial collaborative group: Cisplatin-based adjuvant chemotherapy in patients with completely resected non-small-cell lung cancer. N Engl J Med 2004;350:351-60. [Crossref] [PubMed]
- Winton T, Livingston R, Johnson D, et al. Vinorelbine plus cisplatin vs. observation in resected non-small-cell lung cancer. N Engl J Med 2005;352:2589-97. [Crossref] [PubMed]
- Douillard JY, Rosell R, De Lena M, et al. Adjuvant vinorelbine plus cisplatin versus observation in patients with completely resected stage IB-IIIA non-small-cell lung cancer (Adjuvant Navelbine International Trialist Association [ANITA]): a randomised controlled trial. Lancet Oncol 2006;7:719-27. [Crossref] [PubMed]
- Chemotherapy in non-small cell lung cancer: a meta-analysis using updated data on individual patients from 52 randomised clinical trials. Non-small Cell Lung Cancer Collaborative Group. BMJ 1995;311:899-909. [Crossref] [PubMed]
- Pignon JP, Tribodet H, Scagliotti GV, et al. Lung adjuvant cisplatin evaluation: a pooled analysis by the LACE Collaborative Group. J Clin Oncol 2008;26:3552-9. [Crossref] [PubMed]
- Douillard JY, Tribodet H, Aubert D, et al. Adjuvant cisplatin and vinorelbine for completely resected non-small cell lung cancer: subgroup analysis of the Lung Adjuvant Cisplatin Evaluation. J Thorac Oncol 2010;5:220-8. [Crossref] [PubMed]
- Kenmotsu H, Yamamoto N, Yamanaka T, et al. Randomized phase III study of pemetrexed/cisplatin (Pem/Cis) versus vinorelbine/cisplatin (Vnr/Cis) for completely resected stage II-IIIA non-squamous non-small-cell lung cancer (Ns-NSCLC): the JIPANG study. J Clin Oncol 2019;37:abstr 8501.
- Wakelee HA, Dahlberg SE, Keller SM, et al. Adjuvant chemotherapy with or without bevacizumab in patients with resected non-small-cell lung cancer (E1505): an open-label, multicentre, randomised, phase 3 trial. Lancet Oncol 2017;18:1610-23. [Crossref] [PubMed]
- Gadgeel SM. Role of chemotherapy and targeted therapy in early-stage non-small cell lung cancer. Am Soc Clin Oncol Educ Book 2017;37:630-9. [Crossref] [PubMed]
- Massuti B, Cobo M, Rodriguez-Paniagua M, et al. PL 02.04 SCAT Ph III trial: Adjuvant CT based on BRCA1 levels in NSCLC N+ resected patients. Final survival results a spanish Lung cancer group trial. J Thorac Oncol 2017;12:S1605. [Crossref]
- Moran T, Wei J, Cobo M, et al. Two biomarker-directed randomized trials in European and Chinese patients with nonsmall-cell lung cancer: the BRCA1-RAP80 Expression Customization (BREC) studies. Ann Oncol 2014;25:2147-55. [Crossref] [PubMed]
- Wislez M, Barlesi F, Besse B, et al. Customized adjuvant phase II trial in patients with non-small-cell lung cancer: IFCT-0801 TASTE. J Clin Oncol 2014;32:1256-61. [Crossref] [PubMed]
- Bepler G, Williams C, Schell MJ, et al. Randomized international phase III trial of ERCC1 and RRM1 expression-based chemotherapy versus gemcitabine/carboplatin in advanced non-small-cell lung cancer. J Clin Oncol 2013;31:2404-12. [Crossref] [PubMed]
- Novello S, Grohe C, Geissler M, et al. Preliminary results of the international tailored chemotherapy adjuvant trial: the ITACA trial. J Thorac Oncol 2015;10:S179-80.
- Chen HY, Yu SL, Chen CH, et al. A five-gene signature and clinical outcome in non-small-cell lung cancer. N Engl J Med 2007;356:11-20. [Crossref] [PubMed]
- Brock MV, Hooker CM, Ota-Machida E, et al. DNA methylation markers and early recurrence in stage I lung cancer. N Engl J Med 2008;358:1118-28. [Crossref] [PubMed]
- Sandoval J, Mendez-Gonzalez J, Nadal E, et al. A prognostic DNA methylation signature for stage I non-small-cell lung cancer. J Clin Oncol 2013;31:4140-7. [Crossref] [PubMed]
- Boeri M, Pastorino U, Sozzi G. Role of microRNAs in lung cancer: microRNA signatures in cancer prognosis. Cancer J 2012;18:268-74. [Crossref] [PubMed]
- Landi MT, Zhao Y, Rotunno M, et al. MicroRNA expression differentiates histology and predicts survival of lung cancer. Clin Cancer Res 2010;16:430-41. [Crossref] [PubMed]
- Kelly K, Altorki NK, Eberhardt WEE, et al. Adjuvant erlotinib versus placebo in patients with stage IB-IIIA nonsmall-cell lung cancer (RADIANT): a randomized, double-blind, Phase III trial. J Clin Oncol 2015;33:4007-14. [Crossref] [PubMed]
- Pennell NA, Neal JW, Chaft JE, et al. SELECT: A multicenter phase II trial of adjuvant erlotinib in resected early-stage EGFR mutation-positive NSCLC. J Clin Oncol 2014;35:abstr 7514.
- Goss GD, O'Callaghan C, Lorimer I, et al. Gefitinib versus placebo in completely resected non-small-cell lung cancer: results of the NCIC CTG BR19 study. J Clin Oncol 2013;31:3320-6. [Crossref] [PubMed]
- Yue D, Xu S, Wang Q, et al. Erlotinib versus vinorelbine plus cisplatin as adjuvant therapy in Chinese patients with stage IIIA EGFR mutation-positive non-small-cell lung cancer (EVAN): a randomised, open-label, phase 2 trial. Lancet Respir Med 2018;6:863-73. [Crossref] [PubMed]
- Govindan R, Mandrekar SJ, Gerber DE, et al. ALCHEMIST trials: a golden opportunity to transform outcomes in early-stage non-small cell lung cancer. Clin Cancer Res 2015;21:5439-44. [Crossref] [PubMed]
- Cuppens K, Vansteenkiste J. Vaccination therapy for non-small-cell lung cancer. Curr Opin Oncol 2014;26:165-70. [Crossref] [PubMed]
- Vansteenkiste JF, Cho BC, Vanakesa T, et al. Efficacy of the MAGE-A3 cancer immunotherapeutic as adjuvant therapy in patients with resected MAGE-A3-positive non-small-cell lung cancer (MAGRIT): a randomised, double-blind, placebo-controlled, phase 3 trial. Lancet Oncol 2016;17:822-35. [Crossref] [PubMed]
- Butts C, Socinski MA, Mitchell PL, et al. Tecemotide (L-BLP25) versus placebo after chemoradiotherapy for stage III non-small-cell lung cancer (START): a randomised, double-blind, phase 3 trial. Lancet Oncol 2014;15:59-68. [Crossref] [PubMed]
- Reck M, Rodríguez-Abreu D, Robinson AG, et al. Pembrolizumab versus chemotherapy for PD-L1-positive non-small-cell lung cancer. N Engl J Med 2016;375:1823-33. [Crossref] [PubMed]
- Owen D, Chaft JE. Immunotherapy in surgically resectable non-small cell lung cancer. J Thorac Dis 2018;10:S404-11. [Crossref] [PubMed]
- Kimura H, Matsui Y, Ishikawa A, et al. Randomized controlled phase III trial of adjuvant chemo-immunotherapy with activated killer T cells and dendritic cells in patients with resected primary lung cancer. Cancer Immunol Immunother 2015;64:51-9. [Crossref] [PubMed]
- Ather S, Kadir T, Gleeson F. Artificial intelligence and radiomics in pulmonary nodule management: current status and future applications. Clin Radiol 2020;75:13-9. [Crossref] [PubMed]
- Jha S, Topol EJ. Adapting to artificial intelligence: radiologists and pathologists as information specialists. JAMA 2016;316:2353-4. [Crossref] [PubMed]