Comprehensive analysis of blood-based biomarkers for predicting immunotherapy benefits in patients with advanced non-small cell lung cancer
Introduction
Treatment with immune checkpoint inhibitors (ICIs) has improved the survival of patients with advanced non-small cell lung cancer (NSCLC), especially those without driver mutations (1-4). The combination of ICI and targeted therapeutic agents or cytotoxic chemotherapeutics has improved durable antitumor responses in a subset of patients with potentially refractory metastatic NSCLC (5-8). However, some patients do not respond to ICI. Programmed death-ligand 1 (PD-L1) on tumor cell surface, with a tumor proportion score (TPS) of ≥50%, is a reliable biomarker for ICI treatment response. However, the predictive value of PD-L1 may be poor among patient subgroups with TPS <50% (9). Therefore, studies are ongoing to identify additional predictive tumor-based markers for ICI response, such as tumor-infiltrating lymphocytes, microsatellite instability (MSI), mismatch-repair deficiency, and tumor mutational burden (TMB) (10). However, PD-L1 expression on the tumor cell is the only biomarker for ICI response used in routine practice (11), and other potential candidates are not currently clinically relevant.
Several types of liquid biopsy have been considered as potential sources of biomarkers for ICI treatment response. Liquid biopsy is a minimally invasive and easily accessible method that can aid in early diagnosis, monitoring treatment responses, and predicting survival in patients with lung cancer. Circulating tumor cells (CTCs), which can promote cancer metastasis, can be either directly or indirectly associated with antitumor or pro-tumor cell populations of the immune system, including various lymphocyte subtypes (12). Furthermore, CTCs released from the primary tumor survive in the blood microenvironment by interacting with other peripheral blood cells (PBCs), including neutrophils, platelets, and macrophages (13). Inflammation contributes to cancer pathogenesis and progression (14). Peripheral blood markers of systemic inflammation such as absolute neutrophil count (ANC) (15), neutrophil-to-lymphocyte ratio (NLR) (16,17), derived NLR (dNLR) (15,17), and platelet-to-lymphocyte ratio (PLR) (16), are considered potential biomarkers for predicting the survival of patients with cancer. Analysis of circulating cell-free tumor DNA (ctDNA) levels can provide real-time information regarding tumor cell death because ctDNA is released from dying cancer cells and is rapidly cleared from the blood (half-life of 2 h) (18). Additionally, ctDNA is a better cancer biomarker than serum protein biomarkers because tumor-specific mutations can be detected with it (19).
Previous studies on individual blood-based biomarkers for ICI response have yielded promising candidates with marked prognostic and predictive value. Despite liquid biopsy having several advantages compared with tumor biopsy, validated and consistent biomarkers in liquid biopsy samples have not yet been identified for cancer. This could be attributed to several factors: limited availability of robust technologies, decreased reproducibility, lack of standardized sample preparation methods, timing of sample collection, and types of liquid samples (20). Hence, there is a need to develop a comprehensive one-stop analysis method for various blood sources. This study aimed to investigate the potential of CTCs, PBCs, and circulating cell-free DNA (cfDNA) in liquid biopsy as predictive biomarkers for ICI treatment response in patients with advanced NSCLC. We present the following article in accordance with the STROBE reporting checklist (available at http://dx.doi.org/10.21037/tlcr-21-100).
Methods
Patients and study design
This study utilized a prospective design and involved collection of blood samples from patients receiving anti-PD-1 or anti-PD-L1 treatment for advanced NSCLC. The inclusion criteria were as follows: patients aged >18 years with stage IIIB–IV NSCLC receiving pembrolizumab or atezolizumab at the Chonnam National University Hwasun Hospital between July 2019 and June 2020. Patients were included irrespective of the number of previous therapies or PD-L1 expression levels (Figure 1A). An additional inclusion criterion was the presence of at least one measurable lesion that was not previously irradiated; the lesion was defined using the Response Evaluation Criteria in Solid Tumors (RECIST) version 1.1 (21).
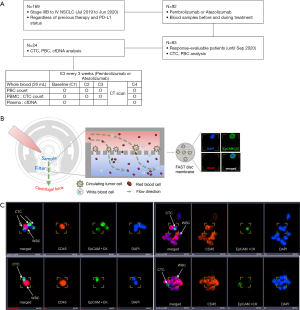
The trial was conducted in accordance with the Declaration of Helsinki (as revised in 2013). The study was approved by the institutional review board of the relevant institution (No.: CNUHH-2019-108) and informed consent was taken from all individual participants.
Blood sample collection, processing, treatment, and assessment
The study flowchart is shown in Figure 1A. Complete blood cells were analyzed to record the number of PBCs such as white blood cells (WBCs), ANC, NLR, dNLR, and PLR before each ICI administration cycle [cycles 1–4 (C1 to C4)]. Additional blood samples were prospectively collected in K2-EDTA tubes (BD, NJ, USA) to analyze CTCs and cfDNA after C1–C4. Whole blood samples (20 mL) were collected and centrifuged to obtain plasma and peripheral blood mononuclear cells (PBMCs). Plasma and PBMC samples were stored to extract cfDNA and enrich CTCs, respectively, until the samples were transported to central laboratories (Clinomics, Daejeon, South Korea for CTC and Asan Medical Center, Seoul, South Korea for cfDNA).
Blood samples were subjected to centrifugation at 1,000 g for 10 min to obtain plasma. Next, the plasma was transferred to a new 2 mL centrifuge tube and subjected to centrifugation at 16,000 g and 4 °C for 10 min to remove cellular debris. The clear plasma was stored in 1 mL aliquots at −80 °C until use. To prevent DNA contamination from blood cells, the plasma was isolated within 2 h of blood collection. PBMCs were isolated using density-gradient centrifugation with Ficoll-PaqueTM PLUS (GE Healthcare, Chicago, USA) (d =1.077 g/mL) following the manufacturer’s instructions. In brief, an equal volume of Ficoll-PaqueTM PLUS solution was added to the blood sample after removing the plasma. The mixture was centrifuged at 800 g for 20 min. The PBMC fraction was recovered from the separated blood cell fraction. PBMCs were cryopreserved in liquid nitrogen with fetal calf serum (Invitrogen) containing 10% dimethyl sulfoxide (Thermo Fisher Scientific) and stored at −80 or −140 °C (liquid nitrogen) until further analyses.
Patients received ICI once every three weeks. They were intravenously administered with pembolizumab and atezolizumab at fixed doses of 200 and 1,200 mg, respectively. Treatment continued until the patient either experienced a serious adverse event, had confirmed disease progression, or withdrew informed consent. Computed tomography (CT) was performed to analyze the tumor at baseline and every three cycles thereafter. Clinical responses to the treatment were defined according to RECIST (version 1.1) (21). In cases where the investigator expected further clinical benefits, patients could continue treatment beyond radiological disease progression.
CTC enrichment, isolation, and identification
The CD-PRIMETM system (Clinomics Inc., Ulsan, Korea), comprising CD-FASTTM solo (disc) and CD-OPR-1000TM (disc operating machine), was used for CTC enrichment (22-25). CTCs were isolated on the membrane by rotating the disc using a programmed spin program. Immunofluorescent (IF) staining and CTC identification were performed on the fluid-assisted separation technology (FAST) disc membrane (Figure 1B). Isolated CTCs on the FAST disc membrane were fixed with 4% formaldehyde for 20 min at room temperature (15–25 °C) and permeabilized with 0.1% Triton-X 100 in phosphate-buffered saline (PBS, pH 7.4) for 5 min. Next, CTCs were washed and blocked with 10 µg/mL IgG in PBS. The CTCs were then stained with the following antibody mixture: fluorescein isothiocyanate (FITC)-conjugated anti-cytokeratin (CK) (CAM5.2; BD, NJ, USA), Alexa 488-conjugated anti-pan-CK (AE1/AE3; eBioscience, CA, USA), and FITC-conjugated anti-epithelial cell adhesion molecule (EpCAM) (9C4; BioLegend, CA, USA). The cells were incubated in the antibody mixture, which was added to the filter, for 20 min. The CTCs were washed with 0.01% Tween 20 in PBS, and the nuclei were stained with 4,6-diamidino-2-phenylindole (DAPI). To visualize CTCs on the filter, fluorescent images were captured using a fluorescence microscope (Bioview, Rehovot, Israel) at 40× magnification. The CTCs were identified according to the following criteria: phenotype, EpCAM/CK+, CD45–, and DAPI+; and diameter, >8 µm. The number of CTCs was counted (Figure 1B,C).
Preparation of cfDNA
The cfDNA was isolated from 2.8–6.0 mL (mean 4.4 mL; maximized plasma volume available) plasma via affinity-based binding to magnetic beads following the manufacturer’s instructions (QIAamp MinElute cfDNA kit, QIAGEN, Hilden, Germany). The cfDNA was eluted in 50 µL of ultraclean water, followed by measuring its quantity using a Qubit™ dsDNA HS Assay kit (Thermo Fisher Scientific, MA, USA) and quality using a Bioanalyzer 2100 (Agilent Technologies, CA, USA).
Statistical analyses
Baseline characteristics were analyzed in a response-evaluable population, which was defined as patients who received at least one dose of ICI and for whom response evaluation was available (Figure 1A). The dNLR was calculated as follows: dNLR = ANC/(WBC − ANC) (26). The comparative analysis of continuous and categorical variables between groups was performed using the Mann-Whitney U-test and Pearson’s chi-square or Fisher’s exact tests, respectively. The cut-off values for ANC, NLR, dNLR, PLR, and cfDNA at C1 were calculated based on analyses of receiver operating characteristic (ROC) curves and area under the curve (AUC). The optimal cut-off value was determined as the point at which the Youden index was maximized by the ROC curve.
The study endpoints were durable clinical benefit (DCB), progression-free survival (PFS), and overall survival (OS). DCB was defined as survival without disease progression [complete response (CR) + partial response (PR) + stable disease (SD)] at six months (27). PFS was defined as the time (in months) from the first day of ICI treatment to the day of objective disease progression or death, irrespective of whether the patient was withdrawn from therapy or received another anticancer therapy before progression. OS was measured from the day of the first ICI administration to the day of death or the last day of follow-up. Survival times were estimated for each group using the Kaplan-Meier method. Univariable and multivariable analyses of survival were performed using a Cox proportional hazards model. All statistical analyses were performed using SPSS ver. 25 (IBM Corp., NY, USA) and MedCalc ver. 19.6 (MedCalc Software Ltd., Ostend, Belgium). The differences were considered significant at P<0.05.
Results
Patient characteristics and efficacy of ICI treatment
Clinical characteristics of the patients and classification of patients based on ICI treatment response are summarized in Table 1. The objective response and DCB rates in 83 response-evaluable patients were 19% and 34%, respectively. Patients with DCB were associated with higher proportions of well-known favorable factors for immunotherapy, including male sex, smoking history, presence of wild-type EGFR, and PD-L1 TPS ≥50%, than patients without DCB (non-durable benefit, NDB). Treatment modalities before ICI treatment and duration between previous therapy and ICI treatment were similar between patients with DCB and those with NDB. Most patients were prescribed with ICI as second- or third-line treatment.

Full table
The median PFS and OS of the study cohort were 2.2 months [95% confidence interval (CI): 1.7–2.6] and 9.2 months (95% CI: 7.2–11.1), respectively. The median follow-up duration was 7.3 months (95% CI: 5.5–9.1). Immune-related adverse events developed in 13 patients [thyroiditis (n=7), skin eruption (n=2), adrenal insufficiency (n=2), and pneumonitis (n=2)]. At the cut-off date, 15 patients (18%) were receiving ICI treatment and two discontinued ICI treatment because of adverse events (grade 3 pneumonitis and adrenal insufficiency).
CTC count as a predictive biomarker for ICI treatment response
The median CTC count per 7.5 mL at baseline (C1) was 4.6 (range, 0.0–58.9) in the study cohort. The median CTC count at C1 was not significantly different based on tumor stage (4.8 for stage IVA vs. 5.4 for stage IVB; P=0.390), brain metastasis (4.6 for absent vs. 5.2 for present; P=0.568), and ICI treatment response (5.0 for DCB vs. 4.6 for NDB; P=0.935). However, patients with DCB had lower CTC counts from C2 to C4 of ICI treatment. In contrast, patients with NDB had higher CTC counts from C2 to C4 (Figure 2A). Additionally, the highest differences in median CTC counts between the DCB and NDB groups were observed at the initiation of C2 (Figure 2B). The number of patients with decreased CTC counts at C2 (compared with those at C1) was higher in the DCB group than in the NDB group (57% vs. 34%, P=0.081; Table S1).
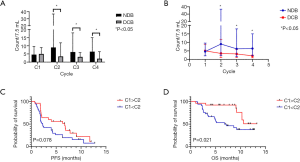
The median PFS (1.9 vs. 2.3 months; P=0.373) and OS (9.1 vs. 10.6 months; P=0.353) were similar between the two groups classified based on median CTC counts at C1 (below vs. above). However, the median PFS (6.7 vs. 2.3 months; Figure 2C) and OS [not reached (NR) vs. 6.8 months; Figure 2D] of patients with decreased CTC counts at C2 (compared with those at C1) was significantly higher than that of those with increased CTC counts at C2 was.
PBC counts at baseline and during treatment as a potential biomarker for ICI treatment response
The values of ANC, NLR, dNLR, and PLR at C1 were not significantly different between the DCB and NDB groups. However, patients with DCB had significantly lower values of ANC, NLR, dNLR, and PLR from C2 to C4 than those with NDB (Figure 3A,B,C,D).
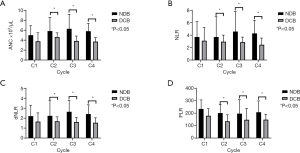
Patients were divided into two groups based on the cut-off value for each component of PBCs at C1, as determined from the ROC analysis (ANC, count =4.3×103, AUC =0.608, P=0.102; NLR, count =4.0, AUC =0.603, P=0.105; dNLR, count =2.0, AUC =0.637, P=0.028; PLR, count =210, AUC =0.613, P=0.087). Patients with lower values of NLR, dNLR, and PLR at C1 had a significantly higher median PFS (NLR, 3.5 vs. 2.0 months; dNLR, 4.3 vs. 1.9 months; PLR, 4.3 vs. 1.9 months; Figure 4A,B,C,D) and OS (NLR, 10.3 vs. 8.1 months; dNLR, 10.6 vs. 7.1 months; PLR, 10.6 vs. 7.7 months; Figure 5A,B,C,D). The values of ANC at C1 did not aid in distinguishing patients with survival benefit (PFS and OS) from the total population.
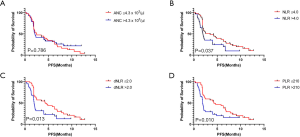
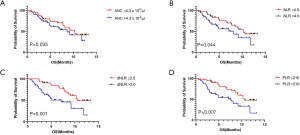
Correlation between clinical outcome and cfDNA levels at baseline and during treatment
Among 24 patients from whom DNA was extracted from the plasma, the median amount of extracted cfDNA at C1 was 6.9 (range, 2.5–98.0) ng/mL. The median value of extracted cfDNA at C1 was not significantly different based on tumor stage (6.9 ng/mL for stage IVA vs. 6.9 ng/mL for stage IVB; P=0.558), brain metastasis (6.8 ng/mL for absent vs. 7.0 ng/mL for present; P=0.465), and ICI treatment response (4.4 ng/mL for DCB vs. 6.9 ng/mL for NDB; P=0.935; Figure 6A). However, patients with markedly decreased cfDNA levels from C1 to C4 had DCB, whereas those with markedly increased cfDNA levels had NDB (Figure 6B).
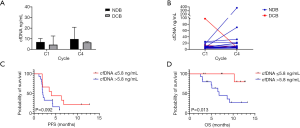
The patients were classified into two groups based on the cut-off cfDNA value at C1 using the ROC analysis (5.8 ng/mL; AUC =0.694, P=0.089). The median PFS (4.0 vs. 2.0 months; Figure 6C) and OS (NR vs. 6.8 months; Figure 6D) were higher in patients with a low cfDNA level at C1. However, the degree of relative change (increase or decrease) in cfDNA levels from C1 to C4 (ΔcfDNA) was not correlated with PFS or OS (Figure S1).
Multivariable analysis of survival and comprehensive monitoring of tumor response to immunotherapy
Multivariable analyses revealed that age, sex, smoking history, EGFR mutation status, PD-L1 expression, dNLR at C1, and CTC count change from C1 to C2 were significant factors for both PFS [hazard ratio (HR) =0.41, 95% CI: 0.18–0.92 for dNLR; HR =0.33, 95% CI: 0.14–0.78 for CTC] and OS (HR =0.25, 95% CI: 0.07–0.92 for dNLR, and HR =0.27, 95% CI: 0.08–0.93 for CTC) (Table S2).
The dNLR and CTC counts were used to stratify patients into the following three subgroups: Group 1 (n=12), dNLR at C1 ≤2.0 and CTC count decreased from C1 to C2 (C1 > C2); Group 2 (n=29), dNLR at C1 ≤2.0 and CTC count increased from C1 to C2 (C1 < C2) or dNLR at C1 >2.0 and CTC count decreased from C1 to C2 (C1 > C2); and Group 3 (n=17), dNLR at C1 >2.0 and CTC count increased from C1 to C2 (C1 < C2). Multivariable analysis revealed that a lower dNLR at baseline and a decreased CTC count from C1 to C2 were associated with improved survival (Figure 7A,B). Kaplan-Meier analyses of PFS and OS revealed a clear difference among the three groups (Figure 7C,D).
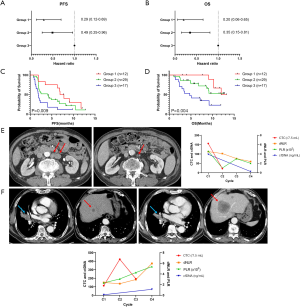
Figure 7E,F shows representative examples of the comprehensive monitoring of tumor response using CTC and PBC count and cfDNA. These cases represent a linear correlation between blood-based biomarkers and tumor burden, especially extra-thoracic metastatic lesions (para-aortic lymph nodes and liver in Figure 7E,F, respectively). Furthermore, CTC, PBC, and cfDNA exhibited dynamic and coherent changes from the early phase of treatment before CT response assessment.
Discussion
This study followed a prospective design and used blood samples obtained from patients receiving ICI treatment. We investigated the feasibility of comprehensive analysis of CTC, PBC, and cfDNA levels in a liquid biopsy sample as predictive biomarkers for ICI treatment response in patients with advanced NSCLC. In this study, patients with lower NLR, dNLR, and PLR values as well as those with lower cfDNA levels at C1 and decreased CTC counts at C2 had significantly improved PFS and OS. Multivariable analysis revealed that low dNLR (≤2.0) values at C1 and decreased CTC counts at C2 (compared with those at C1) were each a significant predictive factor for survival benefit.
Recent technological advances have contributed to improvements in CTC detection methods (28). However, only a few methods have been approved for clinical use, and only a few methods are utilized in the research field because of limitations in method standardization as CTCs exhibit heterogeneity and diverse metastatic potential. Most studies on CTCs in lung cancer have used the CellSearchTM system (Menarini Silicon Biosystems, PA, USA) (29), which is the only United States Food and Drug Administration-approved method for detecting CTCs in metastatic colorectal, prostate, and breast cancers. This method utilizes an immunomagnetic separation technique with anti-EpCAM antibody-based positive selection (DAPI+/CK8,18+/CD45−). However, these surface markers enable the detection of only a subpopulation of epithelial cells and not tumor or mesenchymal cells. The present study utilized the CD-PRIMETM system (Clinomics) with CD-FASTTM solo, a lab-on-a-disc platform using FAST, that enables rapid size-based isolation of CTCs. CTCs are isolated based on size, irrespective of the antigen expression status. This system overcomes the limitations of inconsistency arising from CTC heterogeneity. The other advantages of CD-FASTTM solo (compared with the immunomagnetic separation method) include high-throughput isolation using centrifugal force, label-free isolation, high detection rate (22,23), and minimal loss of mesenchymal and stem cell-like tumor cells with low EpCAM expression. CD-FASTTM also provided uniform, clog-free, ultrafast cell enrichment with pressure drops much less than conventional size-based filtration (24,25).
CTC counts determined using the CellSearchTM system at baseline and during or after treatment have been considered prognostic and predictive biomarkers in several studies on lung cancer (30-32). High numbers (≥5) of CTCs before and after chemotherapy in untreated patients with advanced NSCLC are prognostic factors for poor clinical outcomes (30,31). However, the ability of CTC count to predict ICI treatment response in lung cancer has only been examined in limited studies. Tamminga et al. recently examined patients with NSCLC receiving ICI mainly as a second-line treatment (84%) (32). They demonstrated that the presence of CTC at baseline (32%) and after four weeks (27%) of ICI treatment was an independent predictive factor of poor treatment response, PFS, and OS in patients with advanced NSCLC. In the present study, dynamic changes in CTC counts (in 7.5 mL of whole blood) from baseline to C2 (three weeks) were significant predictive factors for survival. However, CTC counts at baseline were poor biomarkers of treatment response and survival, possibly because CTC detection in high proportions of patients at baseline (88%) and after three weeks (53%) of treatment was attributed to patients previously receiving two or more therapies (47%). Additionally, the CD-PRIMETM system could have been responsible for higher CTC detection rates than CellSearchTM. Therefore, CTC analysis after initiating treatment, rather than at baseline, might aid in predicting ICI treatment response in patients with NSCLC who previously underwent therapy.
Several studies have analyzed CTC surface markers (including PD-L1) and CTC clusters, as well as absolute counts of CTCs. The presence of high numbers of PD-L1-positive CTCs before treatment is associated with poor survival in patients with locally advanced and metastatic NSCLC (33-35). Additionally, Nicolazzo et al. demonstrated that PD-L1-positive CTCs can exhibit dynamic changes during ICI treatment. The absence of PD-L1-positive CTCs at follow-up (6 months) is a predictive marker for clinical benefit (36). In this study, limitations in performing simultaneous and multiple IF staining prevented the implementation of PD-L1 staining of CTCs. CTC clusters comprise immune cells, platelets, dendritic cells, cancer-associated fibroblasts, and tumor stroma, and these types of microenvironments can protect CTC clusters from blood shear damage and immune attacks and consequently promote CTC survival and metastasis (37). The presence of CTC clusters is associated with poor outcomes in patients with lung cancer (38). CTC clusters can be subjected to molecular and genomic analyses to determine optimal therapeutic strategies (39,40). As shown in Figure 1C, clustered CTCs were surrounded by CD45-positive leukocytes. Furthermore, many cell clusters positive for all three markers (EpCAM/CK+/CD45+) were observed using the BioViewCCBSTM system (not shown in the figures). The number of these cells in the NDB subgroup gradually increased after starting ICI treatment (Figure S2). Triple-positive cells are presumed to be circulating atypical cells (derived from cell fusion or interaction between cancer cells and macrophages or mesenchymal cells, or macrophages with phagocytosis of the apoptotic body from cancer cells) with both epithelial-specific and myeloid-specific markers (41). However, further studies are needed to identify the origin of these cells.
The ctDNA is a stratification biomarker for ICI treatment response (42). Detection of residual disease or recurrence in the adjuvant setting (43,44), and estimation of tumor load in the metastatic setting can be achieved by analyzing pre-treatment ctDNA levels (45). Furthermore, identification of specific mutations (46), TMB (47), and MSI (48), using ctDNA analysis can predict ICI treatment response. Additionally, longitudinal monitoring of quantitative changes in ctDNA levels can complement imaging in predicting tumor response, especially in the early phase of ICI treatment (19,45,49). The present study demonstrated that patients with low cfDNA levels at baseline had significantly improved survival. The kinetics of cfDNA levels were correlated with tumor burden. However, this analysis was performed in only 24 patients at C1 and C4, with a limited number of samples. The absolute changes in cfDNA level from C1 to C4 (ΔcfDNA) were not associated with survival. To utilize ctDNA as a biomarker, future studies must analyze blood-based TMB or somatic mutational profiles at baseline and the kinetics of ctDNA levels or variant allele frequency at the initial phase of ICI treatment (earlier than C4) in a large cohort.
The current understanding of the prediction of ICI treatment efficacy suggests that a single biomarker cannot identify the population that will benefit from ICI treatment. The predictive power can be improved by combining various factors representing immune status (50,51). The peripheral blood of patients with cancer comprises products derived from the primary tumor and metastatic sites including CTCs and immune cells of the tumor microenvironment as well as tumor-derived DNA, RNA, and proteins (52). Comprehensive analysis of blood-based biomarkers can provide real-time information on tumor-associated changes in an individual patient with cancer (51). Additionally, liquid biopsy analysis has several advantages compared with tumor biopsy (20), as it enables serial monitoring with repeated examination, detection of minimal residual disease, and prediction of disease progression in extra-thoracic lesions, which are difficult to analyze through routine inspection or chest radiography (Figure 7).
This study had several limitations. Most patients were treated with ICI as a second- or third-line treatment, while a quarter of patients received ICI as a fourth or higher line of treatment. The proportion of patients receiving ICI as a fourth or higher line of treatment was markedly greater in the NDB group than in the DCB group (36% vs. 4%). There is a need to comparatively analyze variables among homogenous populations in previous treatment lines. Additionally, a bias could have been introduced owing to ICI selection, possibly because the Korean Health Insurance System criteria for reimbursement of individual drug costs are set according to PD-L1 TPS (50% or more for pembrolizumab and all comers for atezolizumab in a second or higher line of treatment), although most patients (78%) received atezolizumab. The results of cfDNA analysis could be underpowered due to small numbers of cases (n=24). The integration of additional sources of liquid biopsy, such as exosomes, cytokines, soluble proteins, or immune cells, may be necessary for advanced comprehensive analysis.
Conclusions
The study findings provide novel insights regarding the comprehensive analysis of liquid biopsy in patients with advanced NSCLC who received anti-PD-1/PD-L1 immunotherapy. This study results suggest several potential biomarkers (CTCs, PBCs, and cfDNA) to predict ICI treatment response. The simultaneous analysis of CTC, PBC, and cfDNA levels at baseline or during treatment could aid in stratifying patients at risk for progression and predict disease progression, especially the development of extra-thoracic metastasis. Furthermore, a bioinformatics-based predictive model integrated with an artificial intelligence system must be established for effective screening of populations that can benefit from ICI treatment.
Acknowledgments
Clinomics identified CTCs using immunofluorescent staining and calculated the number of CTCs. This company had no role in the study design, data collection and analysis, decision to publish, or preparation of the manuscript. We would like to thank Editage (
Funding: This research was supported by the Bio & Medical Technology Development Program of the National Research Foundation (NRF) funded by the Korean government (MSIT) (NRF-2019M3E5D1A02067951) and a grant (HCRI 19136) from the Chonnam National University Hwasun Hospital Institute for Biomedical Science.
Footnote
Reporting Checklist: The authors have completed the STROBE reporting checklist. Available at http://dx.doi.org/10.21037/tlcr-21-100
Data Sharing Statement: Available at http://dx.doi.org/10.21037/tlcr-21-100
Peer Review File: Available at http://dx.doi.org/10.21037/tlcr-21-100
Conflicts of Interest: All authors have completed the ICMJE uniform disclosure form (available at http://dx.doi.org/10.21037/tlcr-21-100). IJO reports a grant from Chonnam National University Hwasun Hospital Institute for Biomedical Science for this study. CKP reports NRF Funding for this study. The other authors have no conflicts of interest to declare.
Ethical Statement: The authors are accountable for all aspects of the work in ensuring that questions related to the accuracy or integrity of any part of the work are appropriately investigated and resolved. The trial was conducted in accordance with the Declaration of Helsinki (as revised in 2013). The study was approved by the institutional review board of the relevant institution (No.: CNUHH-2019-108) and informed consent was taken from all individual participants.
Open Access Statement: This is an Open Access article distributed in accordance with the Creative Commons Attribution-NonCommercial-NoDerivs 4.0 International License (CC BY-NC-ND 4.0), which permits the non-commercial replication and distribution of the article with the strict proviso that no changes or edits are made and the original work is properly cited (including links to both the formal publication through the relevant DOI and the license). See: https://creativecommons.org/licenses/by-nc-nd/4.0/.
References
- Herbst RS, Baas P, Kim DW, et al. Pembrolizumab versus docetaxel for previously treated, PD-L1-positive, advanced non-small-cell lung cancer (KEYNOTE-010): a randomised controlled trial. Lancet 2016;387:1540-50. [Crossref] [PubMed]
- Reck M, Rodríguez-Abreu D, Robinson AG, et al. Updated Analysis of KEYNOTE-024: Pembrolizumab Versus Platinum-Based Chemotherapy for Advanced Non-Small-Cell Lung Cancer With PD-L1 Tumor Proportion Score of 50% or Greater. J Clin Oncol 2019;37:537-46. [Crossref] [PubMed]
- Rittmeyer A, Barlesi F, Waterkamp D, et al. Atezolizumab versus docetaxel in patients with previously treated non-small-cell lung cancer (OAK): a phase 3, open-label, multicentre randomised controlled trial. Lancet 2017;389:255-65. [Crossref] [PubMed]
- Herbst RS, Giaccone G, de Marinis F, et al. Atezolizumab for First-Line Treatment of PD-L1-Selected Patients with NSCLC. N Engl J Med 2020;383:1328-39. [Crossref] [PubMed]
- Gadgeel S, Rodríguez-Abreu D, Speranza G, et al. Updated Analysis From KEYNOTE-189: Pembrolizumab or Placebo Plus Pemetrexed and Platinum for Previously Untreated Metastatic Nonsquamous Non-Small-Cell Lung Cancer. J Clin Oncol 2020;38:1505-17. [Crossref] [PubMed]
- Paz-Ares L, Vicente D, Tafreshi A, et al. A Randomized, Placebo-Controlled Trial of Pembrolizumab Plus Chemotherapy in Patients With Metastatic Squamous NSCLC: Protocol-Specified Final Analysis of KEYNOTE-407. J Thorac Oncol 2020;15:1657-69. [Crossref] [PubMed]
- Socinski MA, Jotte RM, Cappuzzo F, et al. Atezolizumab for First-Line Treatment of Metastatic Nonsquamous NSCLC. N Engl J Med 2018;378:2288-301. [Crossref] [PubMed]
- Jotte R, Cappuzzo F, Vynnychenko I, et al. Atezolizumab in Combination With Carboplatin and Nab-Paclitaxel in Advanced Squamous NSCLC (IMpower131): Results From a Randomized Phase III Trial. J Thorac Oncol 2020;15:1351-60. [Crossref] [PubMed]
- Mok TSK, Wu YL, Kudaba I, et al. Pembrolizumab versus chemotherapy for previously untreated, PD-L1-expressing, locally advanced or metastatic non-small-cell lung cancer (KEYNOTE-042): a randomised, open-label, controlled, phase 3 trial. Lancet 2019;393:1819-30. [Crossref] [PubMed]
- Tray N, Weber JS, Adams S. Predictive Biomarkers for Checkpoint Immunotherapy: Current Status and Challenges for Clinical Application. Cancer Immunol Res 2018;6:1122-8. [Crossref] [PubMed]
- Park HY, Oh IJ, Kho BG, et al. Clinical Characteristics of Korean Patients with Lung Cancer Who Have Programmed Death-Ligand 1 Expression. Tuberc Respir Dis (Seoul) 2019;82:227-33. [Crossref] [PubMed]
- Leone K, Poggiana C, Zamarchi R. The Interplay between Circulating Tumor Cells and the Immune System: From Immune Escape to Cancer Immunotherapy. Diagnostics (Basel) 2018;8:59. [Crossref] [PubMed]
- Heeke S, Mograbi B, Alix-Panabières C, et al. Never Travel Alone: The Crosstalk of Circulating Tumor Cells and the Blood Microenvironment. Cells 2019;8:714. [Crossref] [PubMed]
- Diakos CI, Charles KA, McMillan DC, et al. Cancer-related inflammation and treatment effectiveness. Lancet Oncol 2014;15:e493-503. [Crossref] [PubMed]
- Ferrucci PF, Ascierto PA, Pigozzo J, et al. Baseline neutrophils and derived neutrophil-to-lymphocyte ratio: prognostic relevance in metastatic melanoma patients receiving ipilimumab. Ann Oncol 2016;27:732-8. [Crossref] [PubMed]
- Diem S, Schmid S, Krapf M, et al. Neutrophil-to-Lymphocyte ratio (NLR) and Platelet-to-Lymphocyte ratio (PLR) as prognostic markers in patients with non-small cell lung cancer (NSCLC) treated with nivolumab. Lung Cancer 2017;111:176-81. [Crossref] [PubMed]
- Capone M, Giannarelli D, Mallardo D, et al. Baseline neutrophil-to-lymphocyte ratio (NLR) and derived NLR could predict overall survival in patients with advanced melanoma treated with nivolumab. J Immunother Cancer 2018;6:74. [Crossref] [PubMed]
- Diehl F, Schmidt K, Choti MA, et al. Circulating mutant DNA to assess tumor dynamics. Nat Med 2008;14:985-90. [Crossref] [PubMed]
- Goldberg SB, Narayan A, Kole AJ, et al. Early Assessment of Lung Cancer Immunotherapy Response via Circulating Tumor DNA. Clin Cancer Res 2018;24:1872-80. [Crossref] [PubMed]
- Quandt D, Dieter Zucht H, Amann A, et al. Implementing liquid biopsies into clinical decision making for cancer immunotherapy. Oncotarget 2017;8:48507-20. [Crossref] [PubMed]
- Eisenhauer EA, Therasse P, Bogaerts J, et al. New response evaluation criteria in solid tumours: revised RECIST guideline (version 1.1). Eur J Cancer 2009;45:228-47. [Crossref] [PubMed]
- Lim M, Park J, Lowe AC, et al. A lab-on-a-disc platform enables serial monitoring of individual CTCs associated with tumor progression during EGFR-targeted therapy for patients with NSCLC. Theranostics 2020;10:5181-94. [Crossref] [PubMed]
- Kim H, Lim M, Kim JY, et al. Circulating Tumor Cells Enumerated by a Centrifugal Microfluidic Device as a Predictive Marker for Monitoring Ovarian Cancer Treatment: A Pilot Study. Diagnostics (Basel) 2020;10:249. [Crossref] [PubMed]
- Lee A, Park J, Lim M, et al. All-in-one centrifugal microfluidic device for size-selective circulating tumor cell isolation with high purity. Anal Chem 2014;86:11349-56. [Crossref] [PubMed]
- Kim TH, Lim M, Park J, et al. FAST: Size-Selective, Clog-Free Isolation of Rare Cancer Cells from Whole Blood at a Liquid-Liquid Interface. Anal Chem 2017;89:1155-62. [Crossref] [PubMed]
- Proctor MJ, McMillan DC, Morrison DS, et al. A derived neutrophil to lymphocyte ratio predicts survival in patients with cancer. Br J Cancer 2012;107:695-9. [Crossref] [PubMed]
- Qin A, Street L, Cease K, et al. Clinical Determinants of Durable Clinical Benefit of Pembrolizumab in Veterans With Advanced Non-Small-Cell Lung Cancer. Clin Lung Cancer 2017;18:559-64. [Crossref] [PubMed]
- Kowalik A, Kowalewska M, Gozdz S. Current approaches for avoiding the limitations of circulating tumor cells detection methods-implications for diagnosis and treatment of patients with solid tumors. Transl Res 2017;185:58-84.e15. [Crossref] [PubMed]
- Wang L, Balasubramanian P, Chen AP, et al. Promise and limits of the CellSearch platform for evaluating pharmacodynamics in circulating tumor cells. Semin Oncol 2016;43:464-75. [Crossref] [PubMed]
- Krebs MG, Sloane R, Priest L, et al. Evaluation and prognostic significance of circulating tumor cells in patients with non-small-cell lung cancer. J Clin Oncol 2011;29:1556-63. [Crossref] [PubMed]
- Lindsay CR, Faugeroux V, Michiels S, et al. A prospective examination of circulating tumor cell profiles in non-small-cell lung cancer molecular subgroups. Ann Oncol 2017;28:1523-31. [Crossref] [PubMed]
- Tamminga M, de Wit S, Hiltermann TJN, et al. Circulating tumor cells in advanced non-small cell lung cancer patients are associated with worse tumor response to checkpoint inhibitors. J Immunother Cancer 2019;7:173. [Crossref] [PubMed]
- Ilié M, Szafer-Glusman E, Hofman V, et al. Detection of PD-L1 in circulating tumor cells and white blood cells from patients with advanced non-small-cell lung cancer. Ann Oncol 2018;29:193-9. [Crossref] [PubMed]
- Wang Y, Kim TH, Fouladdel S, et al. PD-L1 Expression in Circulating Tumor Cells Increases during Radio(chemo)therapy and Indicates Poor Prognosis in Non-small Cell Lung Cancer. Sci Rep 2019;9:566. [Crossref] [PubMed]
- Guibert N, Delaunay M, Lusque A, et al. PD-L1 expression in circulating tumor cells of advanced non-small cell lung cancer patients treated with nivolumab. Lung Cancer 2018;120:108-12. [Crossref] [PubMed]
- Nicolazzo C, Raimondi C, Mancini M, et al. Monitoring PD-L1 positive circulating tumor cells in non-small cell lung cancer patients treated with the PD-1 inhibitor Nivolumab. Sci Rep 2016;6:31726. [Crossref] [PubMed]
- Giuliano M, Shaikh A, Lo HC, et al. Perspective on Circulating Tumor Cell Clusters: Why It Takes a Village to Metastasize. Cancer Res 2018;78:845-52. [Crossref] [PubMed]
- Hou JM, Krebs MG, Lancashire L, et al. Clinical significance and molecular characteristics of circulating tumor cells and circulating tumor microemboli in patients with small-cell lung cancer. J Clin Oncol 2012;30:525-32. [Crossref] [PubMed]
- Gorges TM, Penkalla N, Schalk T, et al. Enumeration and Molecular Characterization of Tumor Cells in Lung Cancer Patients Using a Novel In Vivo Device for Capturing Circulating Tumor Cells. Clin Cancer Res 2016;22:2197-206. [Crossref] [PubMed]
- Zhang Z, Shiratsuchi H, Palanisamy N, et al. Expanded Circulating Tumor Cells from a Patient with ALK-Positive Lung Cancer Present with EML4-ALK Rearrangement Along with Resistance Mutation and Enable Drug Sensitivity Testing: A Case Study. J Thorac Oncol 2017;12:397-402. [Crossref] [PubMed]
- Broncy L, Paterlini-Bréchot P. Cancer-associated circulating atypical cells with both epithelial and macrophage-specific markers. J Lab Precis Med 2018;3:91. [Crossref]
- Cabel L, Proudhon C, Romano E, et al. Clinical potential of circulating tumour DNA in patients receiving anticancer immunotherapy. Nat Rev Clin Oncol 2018;15:639-50. [Crossref] [PubMed]
- Chaudhuri AA, Chabon JJ, Lovejoy AF, et al. Early Detection of Molecular Residual Disease in Localized Lung Cancer by Circulating Tumor DNA Profiling. Cancer Discov 2017;7:1394-403. [Crossref] [PubMed]
- Moding EJ, Liu Y, Nabet BY, et al. Circulating tumor DNA dynamics predict benefit from consolidation immunotherapy in locally advanced non-small-cell lung cancer. Nat Cancer 2020;1:176-83. [Crossref]
- Bratman SV, Yang SYC, Iafolla MAJ, et al. Personalized circulating tumor DNA analysis as a predictive biomarker in solid tumor patients treated with pembrolizumab. Nat Cancer 2020;1:873-81. [Crossref]
- Wang M, Yu L, Wei X, et al. Role of tumor gene mutations in treatment response to immune checkpoint blockades. Precis Clin Med 2019;2:100-9. [Crossref]
- Wang Z, Duan J, Wang G, et al. Allele Frequency-Adjusted Blood-Based Tumor Mutational Burden as a Predictor of Overall Survival for Patients With NSCLC Treated With PD-(L)1 Inhibitors. J Thorac Oncol 2020;15:556-67. [Crossref] [PubMed]
- Zhao P, Li L, Jiang X, et al. Mismatch repair deficiency/microsatellite instability-high as a predictor for anti-PD-1/PD-L1 immunotherapy efficacy. J Hematol Oncol 2019;12:54. [Crossref] [PubMed]
- Anagnostou V, Forde PM, White JR, et al. Dynamics of Tumor and Immune Responses during Immune Checkpoint Blockade in Non-Small Cell Lung Cancer. Cancer Res 2019;79:1214-25. [Crossref] [PubMed]
- Bai R, Lv Z, Xu D, et al. Predictive biomarkers for cancer immunotherapy with immune checkpoint inhibitors. Biomark Res 2020;8:34. [Crossref] [PubMed]
- Nabet BY, Esfahani MS, Moding EJ, et al. Noninvasive Early Identification of Therapeutic Benefit from Immune Checkpoint Inhibition. Cell 2020;183:363-76 e13. [Crossref] [PubMed]
- Joosse SA, Pantel K. Tumor-Educated Platelets as Liquid Biopsy in Cancer Patients. Cancer Cell 2015;28:552-4. [Crossref] [PubMed]