Risk factors for immune checkpoint inhibitor-related pneumonitis in non-small cell lung cancer
Introduction
Cancer immunotherapy with immune checkpoint inhibitors (ICIs), including antibodies against programmed cell death protein 1 (PD-1) or its ligand PD-L1, has been shown to rescue dysregulated T cell antitumor function to mediate cancer cell destruction (1), and has spearheaded a paradigm shift in the therapeutic management of metastatic non-small cell lung cancer (NSCLC) (2). However, disinhibition of T cell function by ICIs can lead to a constellation of organ-specific inflammatory side effects, termed immune-related adverse events (irAEs), which can be fatal without timely intervention. Therefore, identifying risk factors for development of irAEs is important to identify patients who may warrant increased monitoring for irAE while on therapy; however, these are not yet fully understood (3). Among the reported irAEs, immune checkpoint inhibitor-related pneumonitis (CIP) is the most frequent pulmonary toxicity in patients receiving ICIs, especially in those with NSCLC (4,5).
The clinical presentation of CIP is variable, ranging from asymptomatic disease, to respiratory symptoms such as cough and shortness of breath, respiratory failure, and even death (6,7). Clinical trials of ICIs in NSCLC have reported that the incidence of CIP is low (about 3–5%) (8); however, real-world studies have reported incidence up to 5–19% (6,9-12). There is an urgent need to identify the risk factors of CIP due to its high incidence and the widespread use of ICIs. Prior studies have indicated that age, smoking history, underlying lung pathology, and history of thoracic radiotherapy may be predictors of CIP (6,12,13). Recently, we showed that chronic obstructive pulmonary disease (COPD) is associated with better clinical outcomes and that circulating cytokines were potential prognostic factors in NSCLC patients treated with ICIs (14). Although the chronic pulmonary diseases and circulating cytokines of response to ICIs-based therapy have been widely investigated (15-18), there have been few studies exploring the chronic pulmonary diseases and circulating cytokines that predict risk of CIP (19-22).
In this retrospective study of NSCLC patients receiving ICIs, we analyze the influence of clinical characteristics, cancer therapies, COPD, and circulating cytokines on CIP risk, and subsequently describe real-world CIP clinical features. In addition, we develop a predictive model based on these risk factors for the occurrence of CIP.
We present the following article in accordance with the TRIPOD reporting checklist (available at https://tlcr.amegroups.com/article/view/10.21037/tlcr-22-72/rc).
Methods
Patients
We performed a retrospective study to determine the incidence of CIP and risk factors for its development by reviewing patients with NSCLC who received an anti-PD-1/anti-PD-L1 agent during any treatment line between March 2017 and December 2020 at Zhongshan Hospital Fudan University. Demographic, clinical, and survival data were retrieved from electronic medical records. This study was conducted following the Declaration of Helsinki (as revised in 2013) and approved by the Ethics Committee of Zhongshan Hospital, Fudan University (No. B2020-431R). The written informed consent of participants was waived due to the retrospective nature of the study.
Data collection
The clinical information collected for all participants included age-at-treatment, gender, smoking history, Eastern Cooperative Oncology Group performance status (ECOG PS), tumor-node-metastasis (TNM) stage according to the eighth edition of American Joint Committee on Cancer (23), tumor histologic findings, PD-L1 expression status, and therapeutic regimens. Baseline plasma cytokines [tumor necrosis factor (TNF), interleukin-1β (IL-1β), interleukin-2 receptor (IL-2R), interleukin-6 (IL-6), interleukin-8 (IL-8), and interleukin-10 (IL-10)] were analyzed for research purposes using the IMMULITE assay (Siemens, Munich, Bavaria, Germany) based on a chemiluminescence principle. All patients routinely underwent systematic physical examination, routine blood tests and blood biochemistry prior to ICIs treatment. The clinical data of each participant were recorded and checked by trained professional doctors. The follow-up period ended on 15 July 2021.
Assessment of COPD and diagnosis of CIP
A composite measure of COPD that included baseline spirometry [post-bronchodilator forced expiratory volume in 1 second (FEV1)/forced vital capacity (FVC) <0.70], chest computed tomography (CT) imaging (extensive emphysema without alternative diagnoses), or clinical symptoms (chronic cough, expectoration, and exertional dyspnea) was implemented to improve identification of the COPD and non-COPD sub-cohorts as previously described (14).
Since CIP is a diagnosis of exclusion, each case was carefully reviewed to assess the diagnostic certainty of CIP. Patients with clinically apparent alternative diagnoses such as pulmonary infection, tumor progression, heart failure, or other etiologies were excluded. The date of CIP diagnosis and the maximum grade of CIP were recorded according to the Common Terminology Criteria for Adverse Events (CTCAE 5.0; https://ctep.cancer.gov/protocoldevelopment/electronic_applications/docs/ctcae_v5_quick_reference_5x7.pdf). The maximum CIP grade referred to the highest grade of CIP that each participant developed. In line with previous reports (6), we categorized CIP into five groups according to the radiological features: cryptogenic organizing pneumonitis (COP), ground-glass opacity (GGO), interstitial, hypersensitivity, and pneumonitis not otherwise specified (pneumonitis-NOS). For all participants, the serial chest CT scans were retrospectively reviewed by the treating investigator, and if CIP developed meeting criteria as mentioned above, the patient was included in the CIP group for all analyses.
Statistical analysis
The clinical and demographic data of the patients between the groups were analyzed with the Pearson χ2 test or Fisher’s exact tests for categorical variables, and the Mann-Whitney U test for continuous variables. For the exploratory assessment of the relationship between baseline plasma cytokine levels and the prediction of CIP, a receiver operating characteristic (ROC) curve was used to analyze the best cutoff value for IL-8. Univariate logistic regression analysis was used to assess significance of each single factor for predicting CIP, and those significant variables at univariate analysis used in multivariate analysis to determine potential risk factors of CIP. The odds ratio (OR) and its 95% of confidence interval (CI) were presented. Statistical tests were 2-sided, and 5% was set as the level of significance. All variables with a P value <0.05 on the univariate logistic regression analysis were included in the multivariate analyses to produce an OR and 95% CI.
The logistic regression nomogram was based on independent risk factors identified by multivariate analyses, and built by the ‘rms’ package in R (https://cran.r-project.org/web/packages/rms/index.html). To evaluate the discriminative ability of the nomogram model, the ROC curve was plotted by Hmisc package (https://cran.r-project.org/web/packages/Hmisc/index.html) and ROCR package (https://cran.r-project.org/web/packages/ROCR/index.html). The area under curve (AUC) of the ROC curve, also known as the concordance statistic (C-statistic), was used to appraise the nomogram’s validity. Also, the robustness of nomogram model was calculated by bootstrapping 1,000 random resamples for internal validation, and the calibration curves were subsequently drawn to indicate how predictions from the nomogram compare to the observed risks. Statistical analyses were performed using SPSS 23.0 (IBM Corp., Chicago, IL, USA). Additional analytical software used included R software version 4.0.5 (R Core Team, Vienna, Austria), and GraphPad Prism 8.0 (GraphPad Software, La Jolla, CA, USA).
Results
Participant characteristics
A total of 164 patients with NSCLC receiving ICIs treatment were involved in this study, of whom 20 cases (12.2%) developed CIP following ICIs treatment. The detailed demographic, clinical, and therapeutic characteristics are summarized in Table 1. The cohort was predominantly male (83.5%) and current or former smokers (61.0%). Most participants (97.6%) received immunotherapy for stage III/IV NSCLC, while 4 participants with stage I/II were given immunotherapy as neoadjuvant therapy. The distribution of COPD among the participants with and without CIP was significantly different (P=0.012). Although less than half of the patients in the cohort (43.9%) had a history of COPD, 70% of the patients in the CIP group had COPD. Among the 136 participants whose PD-L1 expression was evaluated, PD-L1 expression in ≥50% of tumor cells were observed in 51 participants (37.5%), while 85 participants (62.5%) were shown to have below 50% tumor PD-L1 expression (P=0.028). Notably, participants with CIP had significantly lower baseline IL-8 than those without CIP (6.5 vs. 11.0 pg/mL, P<0.001). No statistically significant differences were observed in the associations between CIP and other peripheral blood biomarkers and clinical characteristics (Table 1).
Table 1
Variable | All patients (n=164) | No CIP (n=144) | CIP (n=20) | P value |
---|---|---|---|---|
Age-at-treatment, year | 65 [60–69] | 65 [60–69] | 64 [62–68] | 0.901 |
<65 | 82 (50.0) | 71 (49.3) | 11 (55.0) | 0.633 |
≥65 | 82 (50.0) | 73 (50.7) | 9 (45.0) | |
Gender | 0.894 | |||
Male | 137 (83.5) | 121 (84.0) | 16 (80.0) | |
Female | 27 (16.5) | 23 (16.0) | 4 (20.0) | |
Smoking history | 0.694 | |||
Never smoker | 64 (39.0) | 57 (39.6) | 7 (35.0) | |
Anytime smoker | 100 (61.0) | 87 (60.4) | 13 (65.0) | |
ECOG PS at initiation of ICI | 0.939 | |||
0–1 | 151 (92.1) | 133 (92.4) | 18 (90.0) | |
≥2 | 13 (7.9) | 11 (7.6) | 2 (10.0) | |
Initial cancer stage | 0.278 | |||
I | 1 (0.6) | 1 (0.7) | 0 (0.0) | |
II | 3 (1.8) | 3 (2.1) | 0 (0.0) | |
III | 32 (19.5) | 25 (17.4) | 7 (35.0) | |
IV | 128 (78.1) | 115 (79.9) | 13 (65.0) | |
COPD | 0.012 | |||
No | 92 (56.1) | 86 (59.7) | 6 (30.0) | |
Yes | 72 (43.9) | 58 (40.3) | 14 (70.0) | |
Tumor histologic type | 0.585 | |||
Adenocarcinoma | 91 (55.5) | 82 (56.9) | 9 (45.0) | |
Squamous cell carcinoma | 55 (33.5) | 47 (32.6) | 8 (40.0) | |
Other | 18 (11.0) | 15 (10.4) | 3 (15.0) | |
PD-L1 expression status | 136 | 120 | 16 | 0.028 |
<50% | 85 (62.5) | 79 (65.8) | 6 (37.5) | |
≥50% | 51 (37.5) | 41 (34.2) | 10 (62.5) | |
Plasma cytokines, median (range) | ||||
TNF (pg/mL) | 7.8 (5.3–10) | 7.7 (5.2–9.9) | 9.2 (7.7–15.4) | 0.659 |
IL-1β (pg/mL) | 5.0 (5.0–5.0) | 5.0 (5.0–5.0) | 5.0 (5.0–5.0) | 0.550 |
IL-2R (U/mL) | 560.0 (455.0–761.0) | 579.0 (466.0–761.0) | 509.5 (439.5–1,117.0) | 0.777 |
IL-6 (pg/mL) | 7.4 (3.9–18.9) | 7.0 (4.0–18.3) | 10.1 (2.5–23.6) | 0.694 |
IL-8 (pg/mL) | 10.0 (7.0–18.5) | 11.0 (8.0–21.5) | 6.5 (6.0–8.8) | <0.001 |
IL-10 (pg/mL) | 5.0 (5.0–5.0) | 5.0 (5.0–5.0) | 5.0 (5.0–8.8) | 0.320 |
Therapeutic regimen | 0.923 | |||
Monotherapy | 59 (36.0) | 52 (36.1) | 7 (35.0) | |
Combined chemotherapy | 105 (64.0) | 92 (63.9) | 13 (65.0) | |
Treatment line | 0.922 | |||
First-line | 70 (42.7) | 62 (43.1) | 8 (40.0) | |
Second-line | 43 (26.2) | 38 (26.4) | 5 (25.0) | |
Subsequent line | 51 (31.1) | 44 (30.6) | 7 (35.0) |
All data are presented as median (interquartile range) or number (%). P values comparing No CIP and CIP cases are from the Chi-squared test, Fisher’s exact test, or Mann-Whitney U test. CIP, immune checkpoint inhibitor-related pneumonitis; ECOG PS, Eastern Cooperative Oncology Group performance status; ICI, immune checkpoint inhibitor; COPD, chronic obstructive pulmonary disease; PD-L1, programmed cell death-ligand 1; TNF, tumor necrosis factor; IL, interleukin.
Clinical and radiological features of CIP
Median time from the initiation of immunotherapy to the development of CIP was 2.9 months, with large variability in individual times (13 days to 19.2 months). We observed no difference in the time to CIP when stratified by CIP severity (P=0.588; Figure 1).
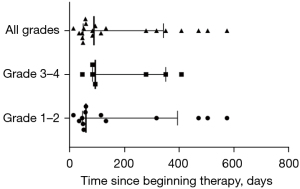
As shown in Figure 2A, more than half of CIP patients (13/20, 65%) experienced grade 1–2 pneumonitis, only 2 (10%) experienced grade 4, and no participants developed to grade 5 CIP in our cohort during the follow-up period. The predominant radiologic features of CIP found on chest CT were COP-like (7/20, 35%), followed by interstitial (6/20, 30%), hypersensitivity (3/20, 15%), pneumonitis-NOS (3/20, 15%), and GGO (1/20, 5%; Figure 2B). The most common symptoms of CIP were shortness of breath (11/20, 55%), cough (10/20, 50%), and expectoration (10/20, 50%). Fever (5/20, 25%) and hemoptysis (2/20, 10%) were less common. A total of 4 participants (4/20, 20%) were asymptomatic at the onset of pneumonitis during regular chest CT evaluation for the immunotherapy efficacy (Figure 2C,2D).
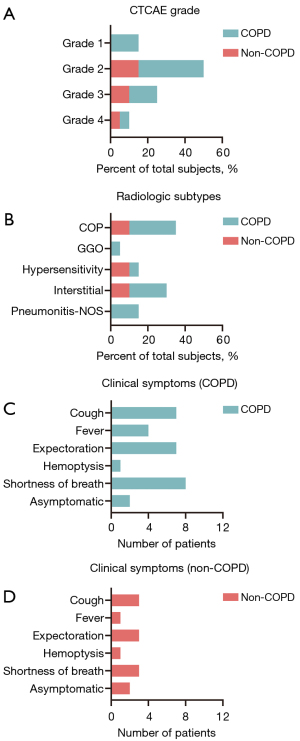
It was also shown that COPD patients experienced a lower grade of CIP, mainly grade 2 (Figure 2A). In the subgroup analysis, COP and interstitial patterns were most common imaging finding of COPD, but the imaging findings of CIP in non-COPD group were varied (Figure 2B). When CIP developed, the COPD patients had relatively higher proportion of clinical symptoms, such as cough, expectoration, and shortness of breath (Figure 2C), and there was similar distribution of clinical symptoms in non-COPD patients (Figure 2D).
Risk factors associated with CIP
Univariate logistic regression analyses assessing hypothesized risk factors for CIP showed that COPD (OR, 3.460; 95% CI: 1.257 to 9.525), and PD-L1 expression status ≥50% (OR, 3.211; 95% CI: 1.090 to 9.458) were associated with increased risk of CIP developing, whereas the baseline of peripheral-blood IL-8 level was found to be associated with a decreased risk (OR, 0.831; 95% CI: 0.691 to 0.999; Table 2). However, a history of smoking, PS at initiation of ICI, tumor histologic subtype, treatment regimens, line of treatment, and other peripheral blood biomarkers did not significantly affect the risk of CIP (Table 2). Multivariate logistic regression analysis revealed that COPD (OR, 7.194; 95% CI: 1.130 to 45.798, P=0.037), PD-L1 expression of ≥50% (OR, 7.184; 95% CI: 1.154 to 44.721, P=0.035), and the baseline IL-8 level (OR, 0.758; 95% CI: 0.587 to 0.978, P=0.033) were significantly and independently associated with the risk of CIP developing (Table 2).
Table 2
CharacteristicsOR (95% CI) | Univariate analysis | Multivariate analysis | |||
---|---|---|---|---|---|
Age-at-treatment, year | |||||
<65 | 1 (ref) | NA | |||
≥65 | 0.796 (0.311–2.036) | 0.634 | |||
Gender | |||||
Male | 1 (ref) | NA | |||
Female | 1.315 (0.403–4.293) | 0.650 | |||
Smoking history | |||||
Never smoker | 1 (ref) | NA | |||
Anytime smoker | 1.217 (0.458–3.234) | 0.694 | |||
ECOG PS at initiation of ICI | |||||
0–1 | 1 (ref) | NA | |||
≥2 | 1.343 (0.275–6.555) | 0.715 | |||
COPD | |||||
No | 1 (ref) | NA | |||
Yes | 3.460 (1.257–9.525) | 0.016 | 7.194 (1.130–45.798) | 0.037 | |
Tumor histologic type | |||||
Adenocarcinoma | 1 (ref) | NA | |||
Squamous cell carcinoma | 1.551 (0.561–4.291) | 0.398 | |||
Other | 1.822 (0.441–7.522) | 0.407 | |||
PD-L1 expression status | |||||
<50% | 1 (ref) | NA | |||
≥50% | 3.211 (1.090–9.458) | 0.034 | 7.184 (1.154–44.721) | 0.035 | |
Peripheral blood cytokines | |||||
TNF (pg/mL) | 1.021 (0.931–1.120) | 0.657 | |||
IL-1β (pg/mL) | 0.838 (0.470–1.496) | 0.551 | |||
IL-2R (U/mL) | 1.000 (0.999–1.001) | 0.776 | |||
IL-6 (pg/mL) | 1.006 (0.977–1.035) | 0.691 | |||
IL-8 (pg/mL) | 0.831 (0.691–0.999) | 0.049 | 0.758 (0.587–0.978) | 0.033 | |
IL-10 (pg/mL) | 1.095 (0.989–1.213) | 0.082 | |||
Therapeutic regimen | |||||
Monotherapy | 1 (ref) | NA | |||
Combined chemotherapy | 1.050 (0.394–2.796) | 0.923 | |||
Treatment line | |||||
First-line | 1 (ref) | NA | |||
Second-line | 1.020 (0.311–3.346) | 0.974 | |||
Subsequent line | 1.233 (0.416–3.651) | 0.705 |
CIP, immune checkpoint inhibitor-related pneumonitis; OR, odds ratio; CI, confidence interval; ECOG PS, Eastern Cooperative Oncology Group performance status; ICI, immune checkpoint inhibitor; COPD, chronic obstructive pulmonary disease; PD-L1, programmed cell death-ligand 1; TNF, tumor necrosis factor; IL, interleukin.
We further performed ROC curve analysis to determine the optimal cutoff value of baseline IL-8 level for the prediction of CIP. The optimal cutoff value of IL-8 level to differentiate the occurrence of CIP was 9.0 pg/mL (AUC =0.744, sensitivity =75.0%, specificity =71.0%; Figure 3A), and divided into ≥9.0 and <9.0 pg/mL for subsequent analysis. The results of multivariate logistic regression analysis showed that having COPD, PD-L1 expression ≥50%, and an IL-8 level <9.0 pg/mL were still independent risk factors for the occurrence of CIP (Figure 3B).
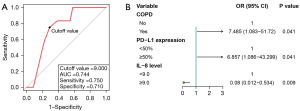
Nomogram construction and validation
The independent risk factors from final multivariate analysis, including COPD, PD-L1 expression status, and the baseline IL-8 level of 9.0 pg/mL, were incorporated to construct a new nomogram for predicting the occurrence of CIP (Figure 4). The nomogram model intuitively revealed the important contribution of COPD and PD-L1 expression, meanwhile, the baseline IL-8 level conferred a similar influence on the occurrence of CIP. The nomogram integrates these factors into an estimated probability of CIP. The C-statistic for the proposed nomogram was 0.883 (95% CI: 0.806 to 0.959), which represents high predictive accuracy of the model (Figure 5A). The calibration plot indicated good agreement between observed and predicted probabilities for the risk of CIP (Figure 5B). Furthermore, we provide a supplementary figure to quickly assess the risk of CIP based on the nomogram (Figure S1).
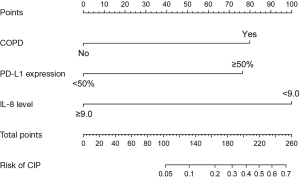
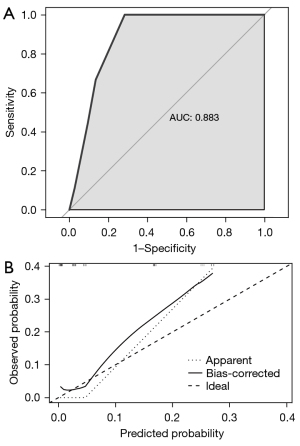
Discussion
Herein, we have presented the incidence of CIP and reports important risk factors for CIP developing in a cohort of NSCLC patients receiving ICIs. We built a nomogram model to predict CIP development, which can be used in clinical practice to determine individual patient risk of CIP. The incidence of CIP in this study (12.2%) was appreciably higher than which had previously been reported in clinical trials (24,25), but is consistent with other real-world data (6,9-12). In clinical practice, there are no strict requirements for patients receiving ICIs as in clinical trials, which may lead to some higher-risk populations of patient receiving ICIs and increase the incidence of CIP. As CIP is potentially fatal but also treatable in its early stages, identification of CIP risk factors and prediction of individual risk of CIP has potential to reduce patient morbidity and mortality by alerting clinicians to patients at high risk of CIP.
We described time-to-onset analysis of CIP, and the varied clinical and radiologic features of the participants who experienced CIP. Our data showed that the onset time of CIP ranged widely (13 days to 19.2 months), as previously reported (a few days to more than one year) (6,11,19). Most cases of CIP tended to occur early (within 6 months of the initiation of ICIs therapy) irrespective of grade, which was consistent with previous research (12). We also noticed that one-fifth of cases were asymptomatic and identified by routine chest CT in our study. As the timing of CIP onset is uncertain and asymptomatic patients are diagnosed by chance during imaging, it is suggested that continuous pharmacovigilance for the signs and symptoms is needed during immunotherapy.
Risk factors of CIP remain incompletely characterized (26). Our study showed that NSCLC patients with COPD are at high-risk of CIP. The inflammatory microenvironment of COPD patients is different than that of other patients: it is chronically inflamed with recruitment and activation of macrophages, CD4+ and CD8+ T cells, dendritic cells, B cells, and neutrophils (27). These activated T cells are increased in tumors and normal lung tissue, and ultimately mediate an inflammatory response of CIP (28,29).
In addition to COPD being associated with the development of CIP, we observed that high expression of PD-L1 was associated with increased risk of CIP. The expression of PD-L1 on at least 50% of tumor cells is associated with significantly longer progression-free and overall survival with ICI (30). Interestingly, in our study, we observed that PD-L1 tumor proportion score of 50% or greater was an independent factor to influence the development of CIP. Increased PD-L1 expression is associated with increased T cell activation (31); however, the activated immune system may cause collateral damage the normal lung tissue, which clinically manifests as CIP (29,32). A prior study by Suresh et al. observed that lymphocytosis in bronchoalveolar lavage fluid (BALF) of CIP patients showed predominance of CD4+ T cells (33). In another study that examined patients at autopsy, the lung histopathology of clinically suspected CIP was also found to involve a significant increase in CD8+ T cells (34).
Current evidence indicates that the adverse events caused by ICIs may develop through a combination of pathways including autoreactive T cells and cytokines (3). The activation of T cells involves the release of excessive inflammatory cytokines, which can contribute to the development of irAEs (35,36). Herein, we showed that elevated baseline plasma IL-8 levels are associated with decreased incidence of CIP. By analyzing previous ICIs clinical trials, we observed that greater IL-8 expression pretreatment likely reflects an immunosuppressive myeloid-enriched tumor microenvironment with limited adaptive T cell responses (16,17). With decreased T cell responsiveness, the likelihood of developing CIP is also reduced. Together, these findings suggest that COPD, PD-L1 expression, and IL-8 as independent risk factors playing critical roles in the increased levels of T cells development of CIP.
To develop a tool that could be used in clinical practice, we created a nomogram model to predict the incidence of CIP in ICIs-treated patients according to the independent risk factors which were COPD, PD-L1 expression, and baseline plasma IL-8 levels. With a user-friendly graphical interface, the predicted probability of each factor is mapped to points from 0 to 100 and summed up to evaluate the probability of developing into a CIP. Validation of the nomogram was assessed through its discriminative power and calibration capabilities. Internal validation demonstrated the excellent calibration and discrimination of our model’s predictive performance, which had a C-statistic of 0.883. This nomogram can identify patients at higher risk for CIP, who can then be monitored more closely for development of CIP and thereby potentially targeted for earlier treatment.
The present study had several limitations. As a single-center retrospective study, our study population may not be representative of the lung cancer patient population as a whole. Our power to discriminate differences in risk factors was limited by the relatively small number of CIP patients. In addition, the threshold values of the baseline plasma IL-8 levels require validation in prospective studies. We are collecting relevant patient samples to reveal the role of cytokines in immunotherapy. The proposed nomogram needs external validation, especially using independent data sets from multiple centers.
Conclusions
In summary, CIP is an adverse event of ICIs therapy, which is more common in a real-world lung cancer cohort than has been previously reported. Risk for development of CIP was shown to be independently associated with COPD, PD-L1 expression, and plasma IL-8 levels. This nomogram model might assist clinical decision-making with CIP risk assessment and the subsequent administration of ICIs. Early recognition of the various clinical features and radiological subtypes of CIP and proper immunosuppressive management are crucial to patients with NSCLC.
Acknowledgments
The authors appreciate the academic support from the AME Lung Cancer Collaborative Group.
Funding: This study was supported by The National Natural Science Foundation of China (No. 82041003, 81630001); Science and Technology Commission of Shanghai Municipality (No. 20DZ2261200, 20Z11901000, 20XD1401200); National key R&D plan (No. 2020YFC2003700); Clinical Research Plan of SHDC (No. SHDC2020CR5010-002); and Shanghai Municipal Key Clinical Specialty (No. SHSLCZDZK02201).
Footnote
Reporting Checklist: The authors have completed the TRIPOD reporting checklist. Available at https://tlcr.amegroups.com/article/view/10.21037/tlcr-22-72/rc
Data Sharing Statement: Available at https://tlcr.amegroups.com/article/view/10.21037/tlcr-22-72/dss
Conflicts of Interest: All authors have completed the ICMJE uniform disclosure form (available at https://tlcr.amegroups.com/article/view/10.21037/tlcr-22-72/coif). Dr. ZZ has received consulting fees from AstraZeneca. Dr. JWN received Research Funding from Companies Genentech/Roche, Merck, Novartis, Boehringer Ingelheim, Exelixis, Nektar Therapeutics, Takeda Pharmaceuticals, Adaptimmune, GSK, Janssen and AbbVie; Consulting or Advisory Role from Companies AstraZeneca, Genentech/Roche, Exelixis, Jounce Therapeutics, Takeda Pharmaceuticals, Eli Lilly and Company, Calithera Biosciences, Amgen, Iovance Biotherapeutics, Blueprint Pharmaceuticals, Regeneron Pharmaceuticals, Natera, Sanofi/Regeneron, D2G Oncology, Surface Oncology and Turning Point Therapeutics; Honoraria from Companies CME Matters, Clinical Care Options CME, Research to Practice CME, Medscape CME, Biomedical Learning Institute CME, MLI Peerview CME, Prime Oncology CME, Projects in Knowledge CME, Rockpointe CME and MJH Life Sciences CME. The other authors have no conflicts of interest to declare.
Ethical Statement: The authors are accountable for all aspects of the work in ensuring that questions related to the accuracy or integrity of any part of the work are appropriately investigated and resolved. The study was conducted in accordance with the Declaration of Helsinki (as revised in 2013). The study was approved by the Ethics Committee of Zhongshan Hospital, Fudan University (No. B2020-431R) and individual consent was waived due to the retrospective nature of the study.
Open Access Statement: This is an Open Access article distributed in accordance with the Creative Commons Attribution-NonCommercial-NoDerivs 4.0 International License (CC BY-NC-ND 4.0), which permits the non-commercial replication and distribution of the article with the strict proviso that no changes or edits are made and the original work is properly cited (including links to both the formal publication through the relevant DOI and the license). See: https://creativecommons.org/licenses/by-nc-nd/4.0/.
References
- Zou W, Wolchok JD, Chen L. PD-L1 (B7-H1) and PD-1 pathway blockade for cancer therapy: Mechanisms, response biomarkers, and combinations. Sci Transl Med 2016;8:328rv4. [Crossref] [PubMed]
- Network NCC. Non-Small Cell Lung Cancer (Version 5.2021).
- Kennedy LB, Salama AKS. A review of cancer immunotherapy toxicity. CA Cancer J Clin 2020;70:86-104. [Crossref] [PubMed]
- Nishino M, Giobbie-Hurder A, Hatabu H, et al. Incidence of Programmed Cell Death 1 Inhibitor-Related Pneumonitis in Patients With Advanced Cancer: A Systematic Review and Meta-analysis. JAMA Oncol 2016;2:1607-16. [Crossref] [PubMed]
- Puzanov I, Diab A, Abdallah K, et al. Managing toxicities associated with immune checkpoint inhibitors: consensus recommendations from the Society for Immunotherapy of Cancer (SITC) Toxicity Management Working Group. J Immunother Cancer 2017;5:95. [Crossref] [PubMed]
- Naidoo J, Wang X, Woo KM, et al. Pneumonitis in Patients Treated With Anti-Programmed Death-1/Programmed Death Ligand 1 Therapy. J Clin Oncol 2017;35:709-17. [Crossref] [PubMed]
- Martins F, Sofiya L, Sykiotis GP, et al. Adverse effects of immune-checkpoint inhibitors: epidemiology, management and surveillance. Nat Rev Clin Oncol 2019;16:563-80. [Crossref] [PubMed]
- Suresh K, Naidoo J, Lin CT, et al. Immune Checkpoint Immunotherapy for Non-Small Cell Lung Cancer: Benefits and Pulmonary Toxicities. Chest 2018;154:1416-23. [Crossref] [PubMed]
- Cho JY, Kim J, Lee JS, et al. Characteristics, incidence, and risk factors of immune checkpoint inhibitor-related pneumonitis in patients with non-small cell lung cancer. Lung Cancer 2018;125:150-6. [Crossref] [PubMed]
- Suresh K, Voong KR, Shankar B, et al. Pneumonitis in Non-Small Cell Lung Cancer Patients Receiving Immune Checkpoint Immunotherapy: Incidence and Risk Factors. J Thorac Oncol 2018;13:1930-9. [Crossref] [PubMed]
- Chu X, Zhao J, Zhou J, et al. Association of baseline peripheral-blood eosinophil count with immune checkpoint inhibitor-related pneumonitis and clinical outcomes in patients with non-small cell lung cancer receiving immune checkpoint inhibitors. Lung Cancer 2020;150:76-82. [Crossref] [PubMed]
- Atchley WT, Alvarez C, Saxena-Beem S, et al. Immune Checkpoint Inhibitor-Related Pneumonitis in Lung Cancer: Real-World Incidence, Risk Factors, and Management Practices Across Six Health Care Centers in North Carolina. Chest 2021;160:731-42. [Crossref] [PubMed]
- Shaverdian N, Lisberg AE, Bornazyan K, et al. Previous radiotherapy and the clinical activity and toxicity of pembrolizumab in the treatment of non-small-cell lung cancer: a secondary analysis of the KEYNOTE-001 phase 1 trial. Lancet Oncol 2017;18:895-903. Erratum in: Lancet Oncol 2017;18:e371. [Crossref] [PubMed]
- Zhou J, Chao Y, Yao D, et al. Impact of chronic obstructive pulmonary disease on immune checkpoint inhibitor efficacy in advanced lung cancer and the potential prognostic factors. Transl Lung Cancer Res 2021;10:2148-62. [Crossref] [PubMed]
- Liu CH, Chen Z, Chen K, et al. Lipopolysaccharide-Mediated Chronic Inflammation Promotes Tobacco Carcinogen-Induced Lung Cancer and Determines the Efficacy of Immunotherapy. Cancer Res 2021;81:144-57. [PubMed]
- Schalper KA, Carleton M, Zhou M, et al. Elevated serum interleukin-8 is associated with enhanced intratumor neutrophils and reduced clinical benefit of immune-checkpoint inhibitors. Nat Med 2020;26:688-92. [Crossref] [PubMed]
- Yuen KC, Liu LF, Gupta V, et al. High systemic and tumor-associated IL-8 correlates with reduced clinical benefit of PD-L1 blockade. Nat Med 2020;26:693-8. [Crossref] [PubMed]
- Shi Y, Liu X, Liu J, et al. Correlations between peripheral blood biomarkers and clinical outcomes in advanced non-small cell lung cancer patients who received immunotherapy-based treatments. Transl Lung Cancer Res 2021;10:4477-93. [Crossref] [PubMed]
- Yamaguchi T, Shimizu J, Hasegawa T, et al. Pre-existing pulmonary fibrosis is a risk factor for anti-PD-1-related pneumonitis in patients with non-small cell lung cancer: A retrospective analysis. Lung Cancer 2018;125:212-7. [Crossref] [PubMed]
- Kowalski B, Valaperti A, Bezel P, et al. Analysis of cytokines in serum and bronchoalveolar lavage fluid in patients with immune-checkpoint inhibitor-associated pneumonitis: a cross-sectional case-control study. J Cancer Res Clin Oncol 2021; [Epub ahead of print]. [Crossref] [PubMed]
- Tyan K, Baginska J, Brainard M, et al. Cytokine changes during immune-related adverse events and corticosteroid treatment in melanoma patients receiving immune checkpoint inhibitors. Cancer Immunol Immunother 2021;70:2209-21. [Crossref] [PubMed]
- Lim SY, Lee JH, Gide TN, et al. Circulating Cytokines Predict Immune-Related Toxicity in Melanoma Patients Receiving Anti-PD-1-Based Immunotherapy. Clin Cancer Res 2019;25:1557-63. [Crossref] [PubMed]
- Amin MB, Greene FL, Edge SB, et al. The Eighth Edition AJCC Cancer Staging Manual: Continuing to build a bridge from a population-based to a more "personalized" approach to cancer staging. CA Cancer J Clin 2017;67:93-9.
- Khunger M, Rakshit S, Pasupuleti V, et al. Incidence of Pneumonitis With Use of Programmed Death 1 and Programmed Death-Ligand 1 Inhibitors in Non-Small Cell Lung Cancer: A Systematic Review and Meta-Analysis of Trials. Chest 2017;152:271-81. [Crossref] [PubMed]
- De Velasco G, Je Y, Bossé D, et al. Comprehensive Meta-analysis of Key Immune-Related Adverse Events from CTLA-4 and PD-1/PD-L1 Inhibitors in Cancer Patients. Cancer Immunol Res 2017;5:312-8. Erratum in: Cancer Immunol Res 2018;6:498-9. [Crossref] [PubMed]
- Owen DH, Wei L, Bertino EM, et al. Incidence, Risk Factors, and Effect on Survival of Immune-related Adverse Events in Patients With Non-Small-cell Lung Cancer. Clin Lung Cancer 2018;19:e893-900. [Crossref] [PubMed]
- Caramori G, Ruggeri P, Mumby S, et al. Molecular links between COPD and lung cancer: new targets for drug discovery? Expert Opin Ther Targets 2019;23:539-53. [Crossref] [PubMed]
- Day D, Hansen AR. Immune-Related Adverse Events Associated with Immune Checkpoint Inhibitors. BioDrugs 2016;30:571-84. [Crossref] [PubMed]
- Postow MA, Sidlow R, Hellmann MD. Immune-Related Adverse Events Associated with Immune Checkpoint Blockade. N Engl J Med 2018;378:158-68. [Crossref] [PubMed]
- Majem M, Cobo M, Isla D, et al. PD-(L)1 Inhibitors as Monotherapy for the First-Line Treatment of Non-Small-Cell Lung Cancer Patients with High PD-L1 Expression: A Network Meta-Analysis. J Clin Med 2021;10:1365. [Crossref] [PubMed]
- Li X, Shao C, Shi Y, et al. Lessons learned from the blockade of immune checkpoints in cancer immunotherapy. J Hematol Oncol 2018;11:31. [Crossref] [PubMed]
- Naidoo J, Page DB, Li BT, et al. Toxicities of the anti-PD-1 and anti-PD-L1 immune checkpoint antibodies. Ann Oncol 2015;26:2375-91. [Crossref] [PubMed]
- Suresh K, Naidoo J, Zhong Q, et al. The alveolar immune cell landscape is dysregulated in checkpoint inhibitor pneumonitis. J Clin Invest 2019;129:4305-15. [Crossref] [PubMed]
- Koelzer VH, Rothschild SI, Zihler D, et al. Systemic inflammation in a melanoma patient treated with immune checkpoint inhibitors-an autopsy study. J Immunother Cancer 2016;4:13. [Crossref] [PubMed]
- Tarhini AA, Zahoor H, Lin Y, et al. Baseline circulating IL-17 predicts toxicity while TGF-β1 and IL-10 are prognostic of relapse in ipilimumab neoadjuvant therapy of melanoma. J Immunother Cancer 2015;3:39. [Crossref] [PubMed]
- Khan S, Khan SA, Luo X, et al. Immune dysregulation in cancer patients developing immune-related adverse events. Br J Cancer 2019;120:63-8. [Crossref] [PubMed]