Cancer-associated changes of emotional brain network in non-nervous system metastatic non-small cell lung cancer patients: a structural connectomic diffusion tensor imaging study
Introduction
Non-small cell lung cancer (NSCLC) is the most prevalent pathological type of lung cancer (1-4). Cancer patients often experience depression and anxiety, which have negative effects on the quality of life and medical treatment of patients (5-11). Moreover, these psychological problems are not only concomitant conditions but also risk factors for cancer occurrence (12). Many cancer patients experience depressive and anxious symptoms meeting established criterion for major depression disorder and anxiety diagnosis, which can change the course of disease of patients with newly diagnosed cancer (13). In addition, abnormal brain structure and function were identified in patients with psychological distress by previous neuroimaging studies (14-16). Therefore, we assess anxious and depressive symptoms of NSCLC patients.
A specified group of brain regions exhibit abnormal functional brain activity and brain structural abnormalities in depressive and anxious patients, which are implicated in the affective processing and emotional regulation (17-23). The impaired brain regions are components of frontolimbic network including prefrontal cortex, temporal cortex, cingulate cortex, insula and thalamus (24). Under-activation of prefrontal regions are found in depressed subjects especially during the induction of negative affect, while both positive and negative emotional stimuli can induce the activation of prefrontal cortex in healthy controls (25-27). Moreover, the functional or structural abnormalities of prefrontal regions may lead to secondary effects on the function of subcortical brain regions (such as putamen and thalamus) and limbic cortex (hippocampus, amygdala and other temporal regions), which are associated with the abnormal appraisal of negative stimuli (28,29).
Previous functional MRI (fMRI) investigations have revealed that pre-chemotherapy cancer patients had increased activations in the inferior frontal gyrus, insula and thalamus during working memory, which may be associated with the self-reported cognitive deficits of cancer patients (30,31). Functional connectivity differences are found between patients before and following chemotherapy (32,33). Treatment-naïve lung cancer patients exhibit decreased functional connectivity in the posterior cingulate gyrus, middle temporal gyrus, inferior parietal, precuneus and middle occipital gyrus, which are associated with the cancer-related cognitive impairment (32). Lung cancer patients before chemotherapy have memory deficits and damaged microstructural architecture of white matter (33). Moreover, Cognitive decline is also found in the cancer patients treated with chemotherapy and disrupted regional network characteristics are found in the frontal, temporal and striatal areas in the chemotherapy-treated cancer patients (34-36).
Although previous research has found functional and structural changes in patients with lung cancer, topological properties damage of the whole brain structural networks associated with cancer patients before chemotherapy are still less clear. Moreover, how these brain areas interact during tumorigenesis and whether these brain areas are coordinated with other particular brain regions involved in the process of emotion remain unclear. The graph theoretical analysis used in our study can overcome the limitations of seed-based approaches used in previous neuroimaging studies and can provide a unique framework for measuring topological characteristics of the brain networks.
This is the first study to document brain structural network changes in a cohort of NSCLC patients before chemotherapy. Based on previous research, we aimed to examine the emotions and topological characteristics of brain white matter network in newly diagnosed non-nervous system metastatic NSCLC patients prior to chemotherapy by the method of graph-theoretic analysis. Since previous neuroimaging studies have demonstrated that the brain function and structure were disrupted in the lung cancer patients after chemotherapy, we hypothesized the cancer may also have effects on the brain network of NSCLC patients.
We present the following article in accordance with the STROBE Reporting Checklist (available at http://dx.doi.org/10.21037/tlcr-20-273).
Methods
Participants
Twenty-four patients with non-nervous system metastatic (identified by structural MRI) NSCLC prior to chemotherapy and 25 gender-, age- and education-matched healthy controls (HC) volunteered in the current study. Patients prior to chemotherapy were recruited from the inpatients at Jiangsu Cancer Hospital after a histologic diagnosis of NSCLC. All participants were aged 18 to 70 years old, right-handed and the years of education are more than 9 years. This study was approved by the ethical commission of the Affiliated Cancer Hospital of Nanjing Medical University (No. ZM201923), conducted in accordance with the Declaration of Helsinki (as revised in 2013). Additionally, written informed consents were obtained from all participants. A summary for the detailed demographic and clinical characteristics of all participants were presented in Table 1.
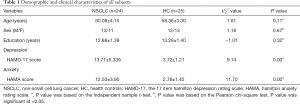
Full table
Exclusion criterion for participants were as follows: (I) previous history of any metastatic tumor; (II) any history of neurological disorders, head trauma, stroke or other central nervous system injury or disease; (III) previous history of severe psychiatric disorders; (IV) any treatment with psychotropic medication; (V) substance abuse history.
Emotion evaluation
The anxious and depressive symptoms were assessed by the 17 item Hamilton Depression Rating Scale (HAMD-17) (37) and Hamilton Anxiety Rating Scale (HAMA) (38).
MRI acquisition parameters
All participates were imaged on a 3.0 T Philips Aachieva scanner. T1-weighted images were acquired under the following conditions: repetition time (TR), 9 ms; echo time (TE), 2.48 ms, slice thickness, 1 mm. Diffusion tensor imaging (DTI) images were acquired with the following scanning parameters: TR, 6,600 ms; TE, 93 ms; slice thickness, 3 mm; non-linear directions, 32; b (noncollinear directions), 1,000 s/mm2.
MRI post-processing
Imaging data were preprocessed using FMRIB’s Software Library (https://www.fMRIb.ox.ac.uk/fsl). After eddy currents and motion artifacts, the diffusion tensor matrix was calculated using the Stejskal and Tanner equation. Then fractional anisotropy (FA) maps were calculated. Finally, individual datasets were normalized to the Montreal neurological institute 152 (MNI-152) space.
In addition, the tractography of white matter was conducted using the diffusion toolkit (http://trackvis.org/dtk/). The streamline was propagated using Fiber Assignment by Continuous Tracking (FACT) algorithm.
Network construction
The whole-brain was parcellated 90 cortical and subcortical regions by the automated anatomical labeling (AAL) template (39). The regions were defined as nodes of the brain network. Additionally, anatomical connections between regions made up edges of the graph. More details about the procedures can be found in previous studies (40,41).
Graph theory analyses
Human brain functional and structural networks exhibit economical small-world properties, which are characterized by densely local connections and few long connections (42). Previous studies showed that the small-world properties of brain networks were affected by depressive disorder and other psychiatric disorders (43,44).
The topological features of brain network may characterize one or several aspects of global and local brain connectivity, which can detect aspects of functional integration and segregation. We estimated the topological properties of the white matter brain networks using graph theory (40). The clustering coefficient (Cnet) and shortest path length (Lnet) of the brain networks have been proposed to quantify the small-world properties of the brain network (42). The clustering coefficient is used to estimate the fraction of the node's neighbors that are also neighbors of each other, which can reflect the level of functional segregation (local efficiency) of the brain network (40). Functional segregation of brain is the ability for specialized processing to occur within densely interconnected groups of brain areas. The shortest path length is proposed as a measure of functional integration (global efficiency) of the brain network (40). Functional integration of brain is the ability to rapidly combine specialized information from distributed brain areas. Moreover, the evaluation of small-world properties requires 100 random networks (The clustering coefficient of random network, Crand and the shortest path length of random network, Lrand), which should preserve the same number of nodes and edges with the brain network (44). The brain network is considered a small-world property (small-worldness=Cnrmal/Lnrmal) if it meets the following conditions: Cnrmal=Cnet/Crand>>1 and Lnrmal=Lnet/Lrand≈1. More details about the measures of brain networks can be found in previous studies (43).
Statistical analysis
In the present study, two-sample t-test was used to compare the age, education and emotional scores, while chi-square test was used to compare the gender of NSCLC patients and HC. In addition, we performed two-sample t-test to compare the differences of the measures of brain networks between NSCLC patients and HC. The method of false discovery rate (FDR) was used to adjust the multiple comparisons. Moreover, the associations between the altered measures of brain regions and the scores of HAMD-17 and HAMA were assessed by the method of Pearson correlation. The significance level was set at P<0.05.
Results
Increased scores of HAMD-17 and HAMA of NSCLC
Compared with HC, NSCLC patients showed increased scores of HAMD-17 (t=9.14, P=0.00) and HAMA (t=11.70, P=0.00), which suggested that NSCLC patients had depressive and anxious emotion (Table 1).
Small-world properties of brain networks in both NSCLC and HC
Both NSCLC patients and HC had small-world properties of the white matter brain networks (Cnet/Crand>>1 and Lnet/Lrand≈1) (Figure 1A,B). Decreased Cnet (t=−2.12, P=0.039) and Lnet (t=2.17, P=0.035) were found in NSCLC patients when compared with HC (Figure 1C). However, there was no differences of small-worldness between groups (t=−1.08, P=0.29) (Figure 1C).
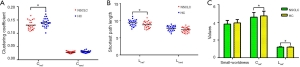
Reduced clustering coefficient in NSCLC
NSCLC patients showed reduced clustering coefficient in the left hippocampus (survived FDR correction) (Table 2; Figure 2A,B). Reduced clustering coefficient was also found in the left middle frontal gyrus (orbital part), inferior frontal gyrus (opercular part), postcentral gyrus, insula, caudate nucleus and right rectus gyrus, Heschl’s gyrus, superior temporal gyrus (temporal pole), inferior temporal gyrus, lenticular nucleus (putamen), however, the differences of these brain regions did not survive FDR correction (Table 2; Figure 2A,B).
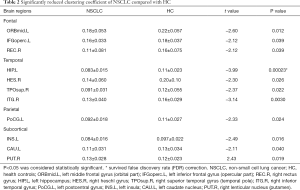
Full table
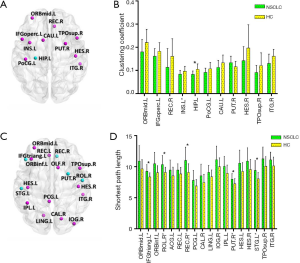
Increased shortest path length in NSCLC
Increased shortest path length was found in the left middle frontal gyrus (orbital part), superior temporal gyrus and right Rolandic operculum, rectus gyrus, lenticular nucleus (putamen) of NSCLC patients (Table 3; Figure 2C,D). NSCLC patients also showed increased shortest path length in the left inferior frontal gyrus (triangular part), inferior frontal gyrus (orbital part), rectus gyrus, posterior cingulate gyrus, inferior parietal (but supramarginal and angular gyri), lingual gyrus, Heschl’s gyrus and right olfactory cortex, calcarine fissure and surrounding cortex, inferior occipital gyrus, Heschl’s gyrus, superior temporal gyrus (temporal pole), inferior temporal gyrus, however, the differences of these brain regions did not survive FDR correction (Table 3; Figure 2C,D).
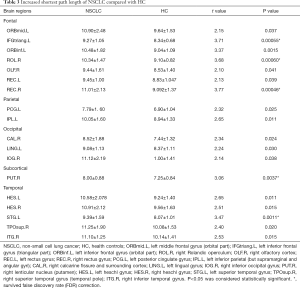
Full table
Associations between altered measures and scores of HAMD-17 and HAMA
For all the impaired measures of the brain network of NSCLC patients, there were correlations were found between the measures and scores of HAMD-17 and HAMA (Table 4).
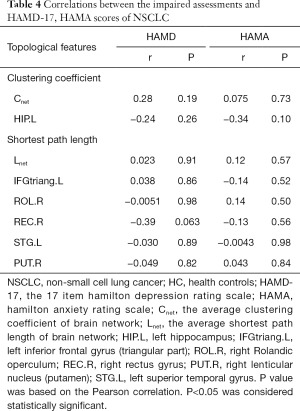
Full table
Discussion
This study investigated the emotions and small-world properties of brain structural networks in non-nervous system metastatic NSCLC patients prior to chemotherapy. We found that NSCLC patients had emotional distress including depression and anxiety. In addition, brain networks exhibited economical small-world topology in both NSCLC patients and HC groups. However, reduced clustering coefficient and increased shortest path length were found in the brain of NSCLC patients. The results revealed that nodal measures were profoundly affected at several regions of prefrontal, temporal and subcortical brain regions, which were considered to be involved in the process and regulation of emotion. These findings indicated that widely distributed structural brain networks were altered in treatment-naïve NSCLC patients with abnormal emotion, thus providing further evidences for the pathological neuromechanisms associated with cancer.
Previous neuroimaging studies have demonstrated that human brain networks have small-world configuration (41,43,44). We also observed that treatment-naïve NSCLC patients had small-world architecture in the white matter brain networks, which provided further supports for the opinion that the small-world property had the ability of displaying tolerance to the brain diseases. The brain networks with small-world features often have higher clustering coefficient (high efficiency of parallel information transfer) and lower shortest path length (low cost) when compared with random networks (40,41). Therefore, the brain networks have economical properties with maximized cost efficiency of parallel information processing, consistent with previous functional and structural MRI studies (41,42).
Although NSCLC patients had economical small-world properties, reduced clustering coefficient and increased shortest path length were found in NSCLC group when compared to HC group. The global efficiency of information processing is often affected by the long range path lengths in the brain network (40). The reduced clustering coefficient and increased shortest path length of NSCLC patients suggested a tendency of decreased local and global efficiency of the brain networks (40). In addition, the decreased clustering coefficient indicated the lower level of fault tolerance of the brain networks during external attacks (41). We thus suspected that the lower values of global and local efficiency might be related to the depressive and anxious emotion of NSCLC patients.
Abnormal nodal measures were found in several brain regions, involving the left middle frontal gyrus (orbital part), superior temporal gyrus, hippocampus and right Rolandic operculum, rectus gyrus, lenticular nucleus (putamen). The prefrontal cortex is associated with the emotional regulation of human (21,45). Previous neuroimaging studies have found that depressive and anxious emotion is associated with the dysfunction and structural abnormalities of prefrontal cortex in depression and anxiety (21,46). The reduced clustering coefficient and increased shortest path length in the prefrontal regions suggested the abnormalities of emotional regulation in NSCLC patients prior to chemotherapy. In addition, several regions belonging to temporal and subcortical cortices were also found to have aberrant topological properties, which might also be associated with the cancer-related depression and anxiety of NSCLC patients.
The functions of hippocampus and putamen are known to be related to emotional perception (47,48). Previous studies indicated that both hippocampus (limbic structures) and putamen (striatal areas) played a key role in the pathophysiology of depression (49). Individuals diagnosed with anxiety and depression were found to be characterized by structural and functional abnormalities in the hippocampus and putamen, which were conceptualized as a central component of affective processing (18,50). In addition, the hippocampus and putamen are considered to receive multimodal inputs from different cortices, which involve in the depression-related memory bias (51). Therefore, the impaired hippocampus and putamen are associated with increased memory sensitivity for negative materials, which may lead to the development of depression and anxiety (51). These findings suggest that cancer-induced emotional distress of treatment-naïve NSCLC patients may be associated with the abnormal topological properties of the prefrontal, temporal and subcortical brain regions of patients.
Conclusions
In summary, this study reveals abnormal emotion and impaired topological properties of brain structural networks in non-nervous system metastatic NSCLC patients prior to chemotherapy using the method of graph-theoretic analysis. Our results provide further supports for the cancer-induced depression and anxiety of NSCLC patients, which may be associated with the abnormal topological properties of the prefrontal, temporal and subcortical brain regions. These findings enhance our understanding of the underlying pathophysiology of cancer-related negative emotion of NSCLC patients.
Acknowledgments
Funding: The work was supported by the grants of: National Natural Science Foundation of China (No. 81871873), Jiangsu Provincial Key Medical Discipline (No. ZDXKA2016009), Foundation of Jiangsu Cancer Hospital (No. ZM201923).
Footnote
Reporting Checklist: The authors have completed the STROBE Reporting Checklist. Available at http://dx.doi.org/10.21037/tlcr-20-273
Data Sharing Statement: Available at http://dx.doi.org/10.21037/tlcr-20-273
Conflicts of Interest: All authors have completed the ICMJE uniform disclosure form (available at http://dx.doi.org/10.21037/tlcr-20-273). The authors have no conflicts of interest to declare.
Ethical Statement: The authors are accountable for all aspects of the work in ensuring that questions related to the accuracy or integrity of any part of the work are appropriately investigated and resolved. This study was approved by the ethical commission of the Affiliated Cancer Hospital of Nanjing Medical University (No. ZM201923), conducted in accordance with the Declaration of Helsinki (as revised in 2013). Additionally, written informed consents were obtained from all participants.
Open Access Statement: This is an Open Access article distributed in accordance with the Creative Commons Attribution-NonCommercial-NoDerivs 4.0 International License (CC BY-NC-ND 4.0), which permits the non-commercial replication and distribution of the article with the strict proviso that no changes or edits are made and the original work is properly cited (including links to both the formal publication through the relevant DOI and the license). See: https://creativecommons.org/licenses/by-nc-nd/4.0/.
References
- Radziszewska A, Karczmarek-Borowska B, Grądalska-Lampart M, et al. Epidemiology, prevention and risk morbidity factors for lung cancer. Polski merkuriusz lekarski: organ Polskiego Towarzystwa Lekarskiego 2015;38:113-8.
- She J, Yang P, Hong Q, et al. Lung cancer in China: challenges and interventions. Chest 2013;143:1117-26. [Crossref] [PubMed]
- Brahmer J, Reckamp KL, Baas P, et al. Nivolumab versus docetaxel in advanced squamous-cell non–small-cell lung cancer. N Engl J Med 2015;373:123-35. [Crossref] [PubMed]
- Reck M, Rodríguez-Abreu D, Robinson AG, et al. Pembrolizumab versus chemotherapy for PD-L1–positive non–small-cell lung cancer. N Engl J Med 2016;375:1823-33. [Crossref] [PubMed]
- Vodermaier A, Linden W, Siu C. Screening for emotional distress in cancer patients: a systematic review of assessment instruments. J Natl Cancer Inst 2009;101:1464-88. [Crossref] [PubMed]
- Faller H, Schuler M, Richard M, et al. Effects of psycho-oncologic interventions on emotional distress and quality of life in adult patients with cancer: systematic review and meta-analysis. Journal of Clinical Oncology 2013;31:782-93. [Crossref] [PubMed]
- Min JA, Yoon S, Lee CU, et al. Psychological resilience contributes to low emotional distress in cancer patients. Support Care Cancer 2013;21:2469-76. [Crossref] [PubMed]
- Steinberg T, Roseman M, Kasymjanova G, et al. Prevalence of emotional distress in newly diagnosed lung cancer patients. Support Care Cancer 2009;17:1493. [Crossref] [PubMed]
- Pinquart M, Duberstein P. Depression and cancer mortality: a meta-analysis. Psychological medicine 2010;40:1797-810. [Crossref] [PubMed]
- Fann JR, Thomas-Rich AM, Katon WJ, et al. Major depression after breast cancer: a review of epidemiology and treatment. Gen Hosp Psychiatry 2008;30:112-26. [Crossref] [PubMed]
- Reich M, Lesur A, Perdrizet-Chevallier C. Depression, quality of life and breast cancer: a review of the literature. Breast Cancer Res Treat 2008;110:9-17. [Crossref] [PubMed]
- Satin JR, Linden W, Phillips MJ. Depression as a predictor of disease progression and mortality in cancer patients: a meta‐analysis. Cancer 2009;115:5349-61. [Crossref] [PubMed]
- Linden W, Vodermaier A, Mackenzie R, et al. Anxiety and depression after cancer diagnosis: prevalence rates by cancer type, gender, and age. J Affect Disord 2012;141:343-51. [Crossref] [PubMed]
- Koch SB, Van Zuiden M, Nawijn L, et al. Aberrant resting‐state brain activity in posttraumatic stress disorder: A meta‐analysis and systematic review. Depress Anxiety 2016;33:592-605. [Crossref] [PubMed]
- Fitzgerald PB, Laird AR, Maller J, et al. A meta‐analytic study of changes in brain activation in depression. Hum Brain Mapp 2008;29:683-95. [Crossref] [PubMed]
- Miller CH, Hamilton JP, Sacchet MD, et al. Meta-analysis of functional neuroimaging of major depressive disorder in youth. JAMA Psychiatry 2015;72:1045-53. [Crossref] [PubMed]
- Norman LJ, Carlisi C, Lukito S, et al. Structural and functional brain abnormalities in attention-deficit/hyperactivity disorder and obsessive-compulsive disorder: a comparative meta-analysis. JAMA Psychiatry 2016;73:815-25. [Crossref] [PubMed]
- Chen CH, Suckling J, Lennox BR, et al. A quantitative meta‐analysis of fMRI studies in bipolar disorder. Bipolar disord 2011;13:1-15. [Crossref] [PubMed]
- Krain AL, Gotimer K, Hefton S, et al. A functional magnetic resonance imaging investigation of uncertainty in adolescents with anxiety disorders. Biol Psychiatry 2008;63:563-8. [Crossref] [PubMed]
- Picó-Pérez M, Radua J, Steward T, et al. Emotion regulation in mood and anxiety disorders: a meta-analysis of fMRI cognitive reappraisal studies. Prog Neuropsychopharmacol Biol Psychiatry 2017;79:96-104. [Crossref] [PubMed]
- Jalbrzikowski M, Larsen B, Hallquist MN, et al. Development of white matter microstructure and intrinsic functional connectivity between the amygdala and ventromedial prefrontal cortex: associations with anxiety and depression. Biol Psychiatry 2017;82:511-21. [Crossref] [PubMed]
- Besteher B, Gaser C, Langbein K, et al. Effects of subclinical depression, anxiety and somatization on brain structure in healthy subjects. J Affect Disord 2017;215:111-7. [Crossref] [PubMed]
- Smith R, Baxter LC, Thayer JF, et al. Disentangling introspective and exteroceptive attentional control from emotional appraisal in depression using fMRI: A preliminary study. Psychiatry Res Neuroimaging 2016;248:39-47. [Crossref] [PubMed]
- Gong Q, He Y. Depression, neuroimaging and connectomics: a selective overview. Biol Psychiatry 2015;77:223-35. [Crossref] [PubMed]
- Kim JU, Weisenbach SL, Zald DH. Ventral prefrontal cortex and emotion regulation in aging: A case for utilizing transcranial magnetic stimulation. Int J Geriatr Psychiatry 2019;34:215-22. [Crossref] [PubMed]
- Lapate RC, Rokers B, Tromp D, et al. Awareness of emotional stimuli determines the behavioral consequences of amygdala activation and amygdala-prefrontal connectivity. Sci Rep 2016;6:25826. [Crossref] [PubMed]
- Morawetz C, Bode S, Baudewig J, et al. Changes in effective connectivity between dorsal and ventral prefrontal regions moderate emotion regulation. Cerebral Cortex 2016;26:1923-37. [Crossref] [PubMed]
- Zuo Z, Ran S, Wang Y, et al. Altered structural covariance among the dorsolateral prefrontal cortex and amygdala in treatment-naïve patients with major depressive disorder. Front Psychiatry 2018;9:323. [Crossref] [PubMed]
- Johnstone T, Van Reekum CM, Urry HL, et al. Failure to regulate: counterproductive recruitment of top-down prefrontal-subcortical circuitry in major depression. J Neurosci 2007;27:8877-84. [Crossref] [PubMed]
- Scherling C, Collins B, Mackenzie J, et al. Pre-chemotherapy differences in visuospatial working memory in breast cancer patients compared to controls: an FMRI study. Front Hum Neurosci 2011;5:122. [Crossref] [PubMed]
- Ahles TA, Root JC, Ryan EL. Cancer-and cancer treatment–associated cognitive change: an update on the state of the science. J Clin Oncol 2012;30:3675. [Crossref] [PubMed]
- Simó M, Rifà-Ros X, Vaquero L, et al. Brain functional connectivity in lung cancer population: an exploratory study. Brain Imaging Behav 2018;12:369-82. [Crossref] [PubMed]
- Simó M, Root JC, Vaquero L, et al. Cognitive and brain structural changes in a lung cancer population. J Thorac Oncol 2015;10:38-45. [Crossref] [PubMed]
- Deprez S, Amant F, Yigit R, et al. Chemotherapy‐induced structural changes in cerebral white matter and its correlation with impaired cognitive functioning in breast cancer patients. Hum Brain Mapp 2011;32:480-93. [Crossref] [PubMed]
- McDonald BC, Conroy SK, Ahles TA, et al. Alterations in brain activation during working memory processing associated with breast cancer and treatment: a prospective functional magnetic resonance imaging study. J Clin Oncol 2012;30:2500. [Crossref] [PubMed]
- Bromis K, Gkiatis K, Karanasiou I, et al. Altered brain functional connectivity in small-cell lung Cancer patients after chemotherapy treatment: A resting-state fMRI study. Comput Math Methods Med 2017;2017:1403940.
- Müller MJ, Dragicevic A. Standardized rater training for the Hamilton Depression Rating Scale (HAMD-17) in psychiatric novices. J Affect Disord 2003;77:65-9. [Crossref] [PubMed]
- Shear MK, Vander Bilt J, Rucci P, et al. Reliability and validity of a structured interview guide for the Hamilton Anxiety Rating Scale (SIGH‐A). Depress Anxiety 2001;13:166-78. [Crossref] [PubMed]
- Tzourio-Mazoyer N, Landeau B, Papathanassiou D, et al. Automated anatomical labeling of activations in SPM using a macroscopic anatomical parcellation of the MNI MRI single-subject brain. NeuroImage 2002;15:273-89. [Crossref] [PubMed]
- Rubinov M, Sporns O. Complex network measures of brain connectivity: uses and interpretations. NeuroImage 2010;52:1059-69. [Crossref] [PubMed]
- Achard S, Salvador R, Whitcher B, et al. A resilient, low-frequency, small-world human brain functional network with highly connected association cortical hubs. J Neurosci 2006;26:63-72. [Crossref] [PubMed]
- Liao X, Vasilakos AV, He Y. Small-world human brain networks: perspectives and challenges. Neurosci Biobehav Rev 2017;77:286-300. [Crossref] [PubMed]
- Zhang J, Wang J, Wu Q, et al. Disrupted brain connectivity networks in drug-naive, first-episode major depressive disorder. Biol Psychiatry 2011;70:334-42. [Crossref] [PubMed]
- Zhang T, Wang J, Yang Y, et al. Abnormal small-world architecture of top–down control networks in obsessive–compulsive disorder. J Psychiatry Neurosci 2011;36:23-31. [Crossref] [PubMed]
- Hiser J, Koenigs M. The multifaceted role of the ventromedial prefrontal cortex in emotion, decision making, social cognition, and psychopathology. Biol Psychiatry 2018;83:638-47. [Crossref] [PubMed]
- Ironside M, O’shea J, Cowen PJ, et al. Frontal cortex stimulation reduces vigilance to threat: implications for the treatment of depression and anxiety. Biol Psychiatry 2016;79:823-30. [Crossref] [PubMed]
- Taylor VA, Grant J, Daneault V, et al. Impact of mindfulness on the neural responses to emotional pictures in experienced and beginner meditators. NeuroImage 2011;57:1524-33. [Crossref] [PubMed]
- Hamilton JP, Gotlib IH. Neural substrates of increased memory sensitivity for negative stimuli in major depression. Biol Psychiatry 2008;63:1155-62. [Crossref] [PubMed]
- Sexton CE, Mackay CE, Ebmeier KP. A systematic review and meta-analysis of magnetic resonance imaging studies in late-life depression. Am J Geriatr Psychiatry 2013;21:184-95. [Crossref] [PubMed]
- Koolschijn PCM, Van Haren NE, Lensvelt‐Mulders GJ, et al. Brain volume abnormalities in major depressive disorder: A meta‐analysis of magnetic resonance imaging studies. Hum Brain Mapp 2009;30:3719-35. [Crossref] [PubMed]
- Belzung C, Willner P, Philippot P. Depression: from psychopathology to pathophysiology. Curr Opin Neurobiol 2015;30:24-30. [Crossref] [PubMed]