Moving towards a customized approach for drug development: lessons from clinical trials with immune checkpoint inhibitors in lung cancer
Introduction
For a long time, the disheartening results obtained in early and advanced investigation with immunotherapeutic agents were justified by the employment of inactive or only marginally active agents and by the incomplete understanding of human tumor immunology. In lung cancer in particular, these disappointing results translated into the common belief that lung cancer represented a non-immunogenic disease, where immunotherapy could achieve only a marginal success.
In recent years, immunologic and clinical science significantly evolved, refusing this preliminary uncorrected axiom and providing a new portrait of lung cancer as an immunological targetable disease. In this regard, the recent thrilling discovery that the high mutational burden, characterizing in particular the not oncogene-driven non-small cell lung cancer (NSCLC), is associated with improved clinical benefit from antibody targeting programmed cell death-1 (PD-1), identifies NSCLC as an ideal target for immunotherapy, similarly to melanoma (1). Considering the now-validated immunogenic potential of lung cancer, the only marginal benefit observed in past years with immunotherapy should probably be attributed to the employment of inadequate statistical methodologies, unable to completely capture the real benefit deriving from the use of immunotherapeutic agents (2).
The intrinsic biological differences existing in term of mechanism of action and kinetic between immunotherapy and chemotherapy or biological therapy, indicates that a new drug development paradigm is needed. Immunotherapeutic agents act as a continuum of biological events, that starts early with immune system activation and that procrastinates until the potential ideal obtainment of a usually delayed clinical benefit.
These biological differences imply that the clinical benefit of immunotherapy, when present, manifests as an overall survival (OS) benefit, replacing the main role achieved by the progression-free survival (PFS) in the era of targeted agents. Thus, the global way to design clinical trials should be theoretically revolutionized in order to adapt to the survival behavior of patients treated with immunotherapeutic agents (graphically represented by the new shape of Kaplan-Meier curves where long survivors and delayed benefit strongly matter).
All major issues and future perspectives regarding the development of immunotherapeutic agents in lung cancer, including choice of adequate endpoints, use of modified statistical methods and introduction of predictive biomarkers in immunotherapy clinical trials, will be explored and argued in this review.
Analysis of end-points in the context of randomized trials
The traditional end-point for advanced NSCLC from both the clinical trial design and the regulatory agencies perspectives has been OS, at least unless molecularly featured patients’ subsets were identified, and specific targeted agents demonstrated to change the prognosis of such particular (and relatively rare) diseases (3). Indeed, with the exception of those drugs approved according to the expression of a specific biomarker (i.e., EGFR, ALK) (4-7), a series of targeted agents failed to be approved by regulatory agencies on the basis of PFS alone, even if positive and/or clinically meaningful (8). The most represented drug-class in this regard refers to antiangiogenics, although bevacizumab and, recently, ramucirumab and nintedanib, have been worldwide approved in the context of unselected or histology-restricted patients (8-11).
The mathematical model which medical oncologist were used to, traditionally attributed to PFS a larger benefit in favour of the investigational drug in comparison with OS. Although controversial and with different interpretations, PFS was introduced as a reasonable end-point for new drugs to be approved from regulatory agencies in a series of solid tumors, including lung cancer. Indeed, in the context of successful trials, experimental drugs were expected to significantly improve PFS with a larger difference in comparison with the standard treatment (usually preceded by an improvement in response rates), than what expected for OS. That was the scenario for many targeted agents for unselected patients, with many trials reproducing the results of the AVAIL trial, where bevacizumab significantly improved PFS, without any benefit in OS (12). As already reported in a series of analysis, the presence of a correlation, its strength and power, between end-points may significantly vary according to drug types (so depending by their mechanism of action) and disease setting (13). As a general rule, in many scenarios a significant correlation between PFS and OS was demonstrated, indicating the larger benefit in PFS did translate in smaller advantages in OS (14). To simplify, we can speculate by assuming that generally with chemotherapy, and with a series of targeted agents, the ratio between the hazard ratio (HR) of PFS and the HR of OS has been since now almost always less the 1, although few (but important) exceptions (15). This paradigm failed to be replicated in the context of randomized trials with immune checkpoint inhibitors for advanced NSCLC (Table 1).
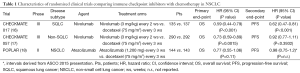
Full table
Nivolumab was recently tested for pretreated advanced NSCLC in the phase III fashion in comparison with the standard of care docetaxel; two twin trials (Checkmate 017 and 057) were prospectively designed to demonstrated the superiority of nivolumab over docetaxel according to histology. These data represent the first release of phase III data with immune-checkpoint inhibitors for NSCLC in the context of unselected patients for (any) biomarker (16,17,19).
With regard to the Checkmate 017, the study was designed to determine a HR of 0.74 at the final analysis (power 90%), with a difference in median OS of 2.5 (7-9.5) months, in favour of nivolumab for patients candidate to receive second-line docetaxel (randomization 1:1), affected by squamous NSCLC. At a (estimated) median follow-up of 15 months (272 enrolled patients, 73% of deaths, with censored patients almost exclusively in the right part of the Kaplan-Meier curve), nivolumab significantly improved OS in comparison with docetaxel, with a HR of 0.59 [95% confidence interval (CI), 0.44-0.79; P<0.001]. Median OS was significantly longer for patients receiving the experimental drug (9.2 months) in comparison with docetaxel (6 months); of particular note, 1-year OS resulted to be almost doubled with nivolumab (42% vs. 24%). According to what reported in the paper, no difference in third line treatment are present. Progression-free-survival resulted to be improved for patients receiving nivolumab with a HR of 0.62 (95% CI, 0.47-0.81; P<0.001), although the difference in medians is actually too small to be considered clinically relevant (from 2.8 to 3.5 months). The study was originally designed with overall response rate (ORR) as co-primary end-point, assuming to detect a response rate difference of 25% (power 90%); ORR resulted to be higher with nivolumab (P=0.008), although less than what expected (20% vs. 9%). To summarize, just looking at the HR, we can conclude that in the case of nivolumab for squamous NSCLC, the ratio between the HRs of PFS and OS results to be higher than 1 (1.05).
In the Checkmate 057 (designed to compare survival in patients with non-squamous NSCLC), at a (estimated) median follow-up of 17 months, nivolumab significantly improved OS in comparison with docetaxel, with a HR of 0.73 (95% CI, 0.59-0.89; P=0.0015). Median OS was significantly longer for patients receiving the experimental drug (12.2 months) in comparison with docetaxel (9.4 months), with a 1-year OS of 51% for nivolumab vs. 39% for docetaxel. No significant difference in PFS was determined, with a HR of 0.92 (95% CI, 0.77-1.11). Similarly, a small difference in ORR in the whole populations was found (19% vs. 12%). Thus, in the context of non-squamous NSCLC, the ratio between the HRs of PFS and OS results to be 1.26.
The interim analysis of the randomized phase II trial POPLAR, at a (estimated) median follow-up of 11 months, showed that atezolizumab did not significantly improve OS (HR, 0.77; 95% CI, 0.55-1.06) nor PFS (HR, 0.98) in comparison with docetaxel for second line NSCLC (regardless of histology) (18). In this case, the ratio between the HRs of PFS and OS results to be 1.27.
Thus, on the basis of the randomized trials to date available comparing these drugs vs. chemotherapy, as reported before, immune checkpoint inhibitors seem to alter the current paradigm assessing that the benefit in PFS is larger than that obtained in OS, given their PFS/OS ratio ranging from 1.05 to 1.27.
The potential role of biomarkers in clinical trials with immune checkpoint inhibitors
The potential predictive role of immune-related biomarkers represents a crucial aspect in recent clinical investigation with immune checkpoint inhibitors, in order to identify that proportion of patients most likely to benefit from immunotherapy, leading to an optimized therapeutic index for these agents.
The detection of PD-L1 (which can be constitutively or inducible expressed on either the surface of tumor cells or upon cancer-infiltrating T-cells) as a potential predictive biomarker, represents one of the most investigated strategy in clinical trials (20). Several early-phase trials in NSCLC have suggested that PD-L1 expression, usually detected by immunohistochemistry (IHC) on tumor cells, may increase the likelihood of response to anti-PD-1 or anti-PD-L1 antibodies. In this regard, we recently conducted a sensitivity analysis of trials in the context of advanced melanoma, NSCLC and genitourinary cancers demonstrating that nivolumab, pembrolizumab and atezolizumab provide a significant differential effect in terms of activity according to PD-L1 expression on tumor cells (21).
Taking into account the randomized trials with anti-PD1 pathway inhibitors in the context of NSCLC patients, the potential predictive value of PD-L1 expression did not constantly emerged. Indeed, the Checkmate 017 trial, demonstrated a benefit in terms of objective response, PFS and OS with nivolumab regardless of tumor cells IHC PD-L1 status, across all the pre-specified expression levels subgroups (1%, 5%, and 10%) (16,19). On the contrary, in the Checkmate 057 trial, the tumor PD-L1 positivity, starting at the lowest expression level (1%), emerged as a significantly predictive biomarker of benefit for nivolumab in terms of both activity and efficacy (17). Moreover, the differential effect of the biomarker was independent from the PD-L1 cut-offs adopted (1%, 5% and 10%). Similar results are reported by Spira et al. in the POPLAR trial, where atezolizumab provided a significant differential effect in objective response, PFS and OS according to the IHC PD-L1 expression on tumor cells and/or tumor-infiltrating immune cells (18). At this regard, others studies demonstrate that, besides the PD-L1 expression by tumor cells, the localization of PD-L1 expression on immune cells infiltrating the tumor may impact on the employment of PD-L1 as a potential predictor of clinical response (22,23). The different cut-offs and methods adopted for the detection and the quantification of PD-L1, such as the type of anti-PD-L1 antibody, the staining techniques, the criteria for classify a ‘positive’ tumor and the samples used for the assay, do not allow to easily compare the results of studies and to deeply speculate about the reliance and consistency between them.
Despite these limitations, the PD-L1 expression on tumor cells or infiltrating immune cells may potentially represent a reasonable candidate biomarker for the selection of patients affected by NSCLC, in order to optimize the treatment strategy with immune checkpoint antibodies. Thus, the analytical validation of this potential biomarker according to a universally shared positivity cut-off is warranted. In addition, the clinical validation of PD-L1 expression as a predictor of efficacy requires further phase III trials stratified according to PD-L1 status and prospective analysis in large cohorts of patients with PD-L1 positive or negative NSCLC.
Recent findings suggest that several other immune regulatory pathways may be involved in the response to PD-1/PD-L1 pathway blockade, such as PD-L2, another PD-1 ligand, PD-1 expression, tumor-infiltrating lymphocytes (TILs) and immunogenic neo-antigens, these last associated with a high mutation burden, that play an important role in immunogenicity (22,24,25). At this regard, the results reported by Rizvi and collegues analyzing a population of NSCLC patients treated with pembrolizumab, demonstrated that a high mutation burden is strongly associated with clinical benefit from PD-1 blockade. Efficacy also correlated with rate of nonsynonymous mutations, alteration in DNA repair pathway, molecular smoking signature and high neoantigens burden (1).
Perspectives for trial design with immune checkpoint inhibitors
As previously described, OS represents the traditional end-point for cancer clinical trials from both the clinical trial design and the regulatory agencies perspectives. To avoid the indiscriminate approval of drugs on the basis of only small survival benefit, restrictive criteria have been defined in order to establish the minimum incremental improvement over standard therapy that would define a clinically meaningful outcome (26).
The results of the Checkmate 017 and 057 randomized trials (and the POPLAR as well, even if a phase II) clearly indicate that, at least for the two immune check-point inhibitors in the more advance phase of development (nivolumab and atezolizumab), the common belief that large benefits in PFS should be obtained in order to determine a significant (despite smaller) benefit in OS, should be abandoned. In addition, the results of these trials in terms of medians strongly call for a deep reflection upon which outcome measures should be chosen to better intercept the effect of an experimental drug.
Traditionally, trials are designed and sized upon the basis of expected differences in terms of medians or proportional reduction in the risk of the chosen events (i.e., the Cox model, if applicable). Such modeling may be significantly affected by the ‘shape’ of the Kaplan-Meier curves, so that these two methods may not easily intercept the benefit of a given drug or treatment. In this regard, it must be addressed that the analysis of the reduction of the proportional risk of a certain end-point (represented by the HR) is just one of the way to look to differences in continuous variables, and the introduction of immunological agents for cancer therapy may represent a crucial point to revolution the traditional way to design clinical trials and to evolve towards trials ‘customized’ upon the drug-type, the disease setting and (hopefully) the biological background.
Figure 1 displays three typical survival curves (according to the Kaplan-Meier model) which each individual oncologist and regulatory agencies may have to deal with when interpreting trial results in the context of a specific clinical scenario:
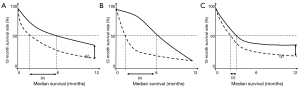
- Panel A shows the results of a theoretical clinical trial whereas the effect of the experimental drug (continuous line) is captured by either the difference in median survival (x) and the HR (i.e., the reduction in the risk of event is maintained proportional during the observation). In addition, the difference captured by the median, is later maintained when performing a landmark analysis of patients event-free at 12 months (y). In this case, we can imagine that the drug starts to work early (curves initiate to separate at the beginning of the study), and continues to be active along the treatment is administered in the same way. Thus, for the clinical trial in panel A, both median and HR would have been ideal when prospectively designed the study;
- In panel B, the results of a clinical study whereas the effect of the drug starts very early (curves dramatically separate immediately). The large benefit in favour of the experimental drug is captured entirely by the medians (x), but, as long as patients relapse in both arms, the differences disappear, and no difference at the 12-month landmark analysis is determined. This is the typical curve of the PFS of targeted agents for the case of oncogene-addicted disease (i.e., EGFR mutant or ALK-rearranged); the drug has a dramatic efficacy earlier (as mirrored by the strong activity in terms of response rates), but resistance occurs and the vast majority of patients (at 12 months in this case) has required a further line of treatment. In such case, to design and power the study on the basis of a difference in medians may allow to provide a trial with a relatively small sample; the drug-efficacy may be captured by using HR as well, although the curves-crossing at a certain time may render this measure not entirely appropriate;
- Panel C shows what to date randomized trials with immune checkpoint inhibitors are displaying in terms of PFS and OS. The benefit of the experimental drug in comparison with the standard is detected later in the study. Medians do not capture the effect of the drug (x), while the landmark analysis may be the best tool to magnify the (y). This is particularly true for immunotherapy in general (i.e., cytokines, ipilimumab and immune checkpoint inhibitors for melanoma), where the effect showed with a landmark analysis is replicated at 18- and 24-month in both lung and melanoma (27). As for panel B, HR may be used, although the reduction in the risk of the event in the first part of the curve is not proportional.
Actually, we cannot consider these tools similar or one substitute of the other, not only for the operative characteristics as explained before. Indeed, each one of them (medians, HR, landmark analysis) may mirror the efficacy of the drug in different contexts. As recently reported, a different key may be adopted to explain deeply this effect: median and HR may replicate the average benefit in the context of the whole sample randomized in the study, while the landmark analysis may identify long-terms survivors, i.e., those (few) patients really benefiting from the drug in terms of survival (as the drug which works is able to select patients on the basis of prognosis) (28).
This intriguing interpretation has implication for translational research: as reported before, the effect of drug dramatically working from the beginning (for example: tyrosine kinase inhibitors for EGFR mutant NSCLC patients), which may be captured by medians (or HR), may be easily monitored at early phases of the trial we are conducting. That allows to change hypotheses to better increase the success rate of the study and to provide more reliable data for clinical practice as well. That represents the principle of adaptive designs for the early phases, which may easily been applied in such context with this kind of drugs. This fascinating approach (i.e., adaptive design) is hard to be applied for drugs working lately (Figure 1C), and in general, in those context whereas the cancer cells are not addicted to a specific pathway which is significantly killed by a given inhibitor.
With these perspectives, the magnification of the benefit to be captured by more appropriately designs for trials and disease settings whereas immune checkpoints inhibitors are employed represents a challenge, given that: (I) the correlation with traditional intermediate end-points (such as PFS) does not work, as reported in precedent chapters; (II) the expression of PD-L1 demonstrate to significantly interact with the treatment effect in non-squamous disease, while no interaction is revealed for squamous histology; (III) biomarkers currently investigated (i.e., PD-L1, mutational load, lymphocytes infiltrate) suffer from analytical and reproducibility issues.
With regard to the latest issue, it seems clear for example that patients with high levels of PD-L1 derive more benefit from these inhibitors, as it seems particularly true when the cut-off is higher for pembrolizumab (29), but the effect is seen in negative patients as well, and it could not entirely considered negligible. According to the results of the Checkmate 057, a patient displaying a 4% PD-L1 expression can potential benefit from nivolumab if considering the 1% threshold as well as not if considering the 5% cut-off (17). Thus, the methodology and the cut-off represents a crucial issue, without forgetting that the expression of such factors depends upon where it is measured (cancer cells, immune cells, etc.) and when it is measured according to the exposure to other treatments (chemotherapy, radiotherapy, etc.). The immune reaction to cancer cells is a dynamic process and patients will be required to be monitored for that, although we currently do not have clear, reproducible and reliable methods to do that, although we have hints that other serological parameters may potentially have a role in this regard (22). Thus, unless a reliable biomarker (whatever it will be, PD-L1, immune cells infiltrate, mutational load, other) is identified, the current ways to improve the success rate of trials (basket and umbrella designs) do not seem to be appropriate for immune-oncology. In this regard, we recently understood that the simple molecular abnormality (for example BRAF mutation V600E) does not imply the efficacy of the specific inhibitor (i.e., vemurafenib), and that the disease context (and the histology) significantly matters, thus not confirming the absolute reliance of basket trials (30). The significant role of histology is highlighted by the results of the Checkmate 017 and 057 as well, whereas PD-L1 results to be predictive only for non-squamous histology.
From the pure trial perspective, the curve’s shape we face with immunotherapy (Figure 1C), requires appropriate designs, given it may deviate from proportional hazards, and the delayed separation may imply a loss of the statistical power (low number of events), with significant implication for the sample size (31). For such reasons, alternative statistical methods should be considered to compute the required number of events for delayed separated curves (simulation, numerical integration) and quantification of the delayed effect (2). In this regard, for the reasons already described, none of the usual surrogate end-point (i.e., PFS, responses, etc.) can be considered useful for immune-oncology, and survival must remain the primary end-point of randomized trials.
In order to better capture the efficacy of the newest upcoming immune checkpoint inhibitors, a non-proportional hazard model should be adopted, and outcome measure assessing events as landmark analysis should be incorporated for the trial’ hypotheses and the sample size quantification. In this regard, landmark analysis may consider absolute differences at a certain time-point (i.e., absolute difference between patients event-free at 1 year) or relative differences at that time-point (i.e., event-based risk- or odds-ratio). Given the peculiar shape of the curve (Figure 1C), whereas HR works only after the median, trials would better designed if the primary end-point would be targeted with both the proportional assumption (i.e., the target HR) and the relative (or absolute) landmark analysis. That would allow increasing the chances to intercept the eventual benefit of the investigated immunotherapy, and to avoid the risk to consider negative a study whereas the drugs is able to identify a patients’ subgroup who significantly benefit from the newest treatment. A graphical summary of the emerging issues of immunotherapy clinical trials design with the possible solutions is proposed in Figure 2.
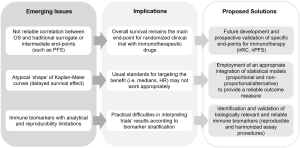
Certainly, intermediate end-point is anyway needed, in order to accelerate the process to introduce a good drug in the context of the available treatments for a given disease. Thus, cancer research is going forward to develop specific end-points for immunotherapy. In this regard, immune-related criteria for both response and adverse events are to date under validation, and immune-related PFS is going forward to be prospectively considered for future trials with these drugs.
Acknowledgements
Funding: This work was supported by a Fellowship Award of the International Association for the Study of Lung Cancer (IASLC) and a grant of the Italian Association for Cancer Research (AIRC My First AIRC Grant No. 14282).
Footnote
Conflicts of Interest: The authors have no conflicts of interest to declare.
References
- Rizvi NA, Hellmann MD, Snyder A, et al. Cancer immunology. Mutational landscape determines sensitivity to PD-1 blockade in non-small cell lung cancer. Science 2015;348:124-8. [PubMed]
- Hoos A, Eggermont AM, Janetzki S, et al. Improved endpoints for cancer immunotherapy trials. J Natl Cancer Inst 2010;102:1388-97. [PubMed]
- Garon EB. Issues surrounding clinical trial endpoints in solid malignancies with a focus on metastatic non-small cell lung cancer. Lung Cancer 2012;77:475-81. [PubMed]
- Rosell R, Carcereny E, Gervais R, et al. Erlotinib versus standard chemotherapy as first-line treatment for European patients with advanced EGFR mutation-positive non-small-cell lung cancer (EURTAC): a multicentre, open-label, randomised phase 3 trial. Lancet Oncol 2012;13:239-46. [PubMed]
- Rosell R, Moran T, Queralt C, et al. Screening for epidermal growth factor receptor mutations in lung cancer. N Engl J Med 2009;361:958-67. [PubMed]
- Shaw AT, Kim DW, Nakagawa K, et al. Crizotinib versus chemotherapy in advanced ALK-positive lung cancer. N Engl J Med 2013;368:2385-94. [PubMed]
- Solomon BJ, Mok T, Kim DW, et al. First-line crizotinib versus chemotherapy in ALK-positive lung cancer. N Engl J Med 2014;371:2167-77. [PubMed]
- Pilotto S, Bonomi M, Massari F, et al. Anti-angiogenic drugs and biomarkers in non-small-cell lung cancer: a 'hard days night'. Curr Pharm Des 2014;20:3958-72. [PubMed]
- Garon EB, Ciuleanu TE, Arrieta O, et al. Ramucirumab plus docetaxel versus placebo plus docetaxel for second-line treatment of stage IV non-small-cell lung cancer after disease progression on platinum-based therapy (REVEL): a multicentre, double-blind, randomised phase 3 trial. Lancet 2014;384:665-73. [PubMed]
- Reck M, Kaiser R, Mellemgaard A, et al. Docetaxel plus nintedanib versus docetaxel plus placebo in patients with previously treated non-small-cell lung cancer (LUME-Lung 1): a phase 3, double-blind, randomised controlled trial. Docetaxel plus nintedanib versus docetaxel plus placebo in patients with previously treated non-small-cell lung cancer (LUME-Lung 1): a phase 3, double-blind, randomised controlled trial. Lancet Oncol 2014;15:143-55. [PubMed]
- Sandler A, Gray R, Perry MC, et al. Paclitaxel-carboplatin alone or with bevacizumab for non-small-cell lung cancer. N Engl J Med 2006;355:2542-50. [PubMed]
- Reck M, von Pawel J, Zatloukal P, et al. Overall survival with cisplatin-gemcitabine and bevacizumab or placebo as first-line therapy for nonsquamous non-small-cell lung cancer: results from a randomised phase III trial (AVAiL). Ann Oncol 2010;21:1804-9. [PubMed]
- Bria E, Massari F, Maines F, et al. Progression-free survival as primary endpoint in randomized clinical trials of targeted agents for advanced renal cell carcinoma. Correlation with overall survival, benchmarking and power analysis. Crit Rev Oncol Hematol 2015;93:50-9. [PubMed]
- Ciani O, Davis S, Tappenden P, et al. Validation of surrogate endpoints in advanced solid tumors: systematic review of statistical methods, results, and implications for policy makers. Int J Technol Assess Health Care 2014;30:312-24. [PubMed]
- Scagliotti GV, Parikh P, von Pawel J, et al. Phase III study comparing cisplatin plus gemcitabine with cisplatin plus pemetrexed in chemotherapy-naive patients with advanced-stage non-small-cell lung cancer. J Clin Oncol 2008;26:3543-51. [PubMed]
- Brahmer J, Reckamp KL, Baas P, et al. Nivolumab versus Docetaxel in Advanced Squamous-Cell Non-Small-Cell Lung Cancer. N Engl J Med 2015;373:123-35. [PubMed]
- Borghaei H, Paz-Ares L, Horn L, et al. Nivolumab versus Docetaxel in Advanced Nonsquamous Non-Small-Cell Lung Cancer. N Engl J Med 2015;373:1627-39. [PubMed]
- Spira AI, Park K, Mazieres J, et al. Efficacy, safety and predictive biomarker results from a randomized phase II study comparing MPDL3280A vs docetaxel in 2L/3L NSCLC (POPLAR). J Clin Oncol 2015;33:abstr 8010.
- Spigel DR, Reckamp KL, Rizvi NA, et al. A phase III study (CheckMate 017) of nivolumab (NIVO; anti-programmed death-1 (PD-1)) vs docetaxel (DOC) in previously treated advanced or metastatic squamous (SQ) cell non-small cell lung cancer (NSCLC). J Clin Oncol 2015;33:abstr 8009.
- Patel SP, Kurzrock R. PD-L1 Expression as a Predictive Biomarker in Cancer Immunotherapy. Mol Cancer Ther 2015;14:847-56. [PubMed]
- Carbognin L, Pilotto S, Milella M, et al. Differential Activity of Nivolumab, Pembrolizumab and MPDL3280A according to the Tumor Expression of Programmed Death-Ligand-1 (PD-L1): Sensitivity Analysis of Trials in Melanoma, Lung and Genitourinary Cancers. PLoS One 2015;10:e0130142. [PubMed]
- Herbst RS, Soria JC, Kowanetz M, et al. Predictive correlates of response to the anti-PD-L1 antibody MPDL3280A in cancer patients. Nature 2014;515:563-7. [PubMed]
- Tumeh PC, Harview CL, Yearley JH, et al. PD-1 blockade induces responses by inhibiting adaptive immune resistance. Nature 2014;515:568-71. [PubMed]
- Schalper KA, Brown J, Carvajal-Hausdorf D, et al. Objective measurement and clinical significance of TILs in non-small cell lung cancer. J Natl Cancer Inst 2015;107. [PubMed]
- Snyder A, Wolchok JD, Chan TA. Genetic basis for clinical response to CTLA-4 blockade. N Engl J Med 2015;372:783. [PubMed]
- Ellis LM, Bernstein DS, Voest EE, et al. American Society of Clinical Oncology perspective: Raising the bar for clinical trials by defining clinically meaningful outcomes. J Clin Oncol 2014;32:1277-80. [PubMed]
- Schadendorf D, Hodi FS, Robert C, et al. Pooled Analysis of Long-Term Survival Data From Phase II and Phase III Trials of Ipilimumab in Unresectable or Metastatic Melanoma. J Clin Oncol 2015;33:1889-94. [PubMed]
- Sobrero AF, Pastorino A, Sargent DJ, et al. Raising the bar for antineoplastic agents: how to choose threshold values for superiority trials in advanced solid tumors. Clin Cancer Res 2015;21:1036-43. [PubMed]
- Garon EB, Rizvi NA, Hui R, et al. Pembrolizumab for the treatment of non-small-cell lung cancer. N Engl J Med 2015;372:2018-28. [PubMed]
- Hyman DM, Puzanov I, Subbiah V, et al. Vemurafenib in Multiple Nonmelanoma Cancers with BRAF V600 Mutations. N Engl J Med 2015;373:726-36. [PubMed]
- Chen TT. Milestone Survival: A Potential Intermediate Endpoint for Immune Checkpoint Inhibitors. J Natl Cancer Inst 2015;107. [PubMed]